Triplet Entropy Loss: Improving The Generalisation of Short Speech Language Identification Systems
CoRR(2020)
摘要
We present several methods to improve the generalisation of language identification (LID) systems to new speakers and to new domains. These methods involve Spectral augmentation, where spectrograms are masked in the frequency or time bands during training and CNN architectures that are pre-trained on the Imagenet dataset. The paper also introduces the novel Triplet Entropy Loss training method, which involves training a network simultaneously using Cross Entropy and Triplet loss. It was found that all three methods improved the generalisation of the models, though not significantly. Even though the models trained using Triplet Entropy Loss showed a better understanding of the languages and higher accuracies, it appears as though the models still memorise word patterns present in the spectrograms rather than learning the finer nuances of a language. The research shows that Triplet Entropy Loss has great potential and should be investigated further, not only in language identification tasks but any classification task.
更多查看译文
关键词
language identification,speech,entropy
AI 理解论文
溯源树
样例
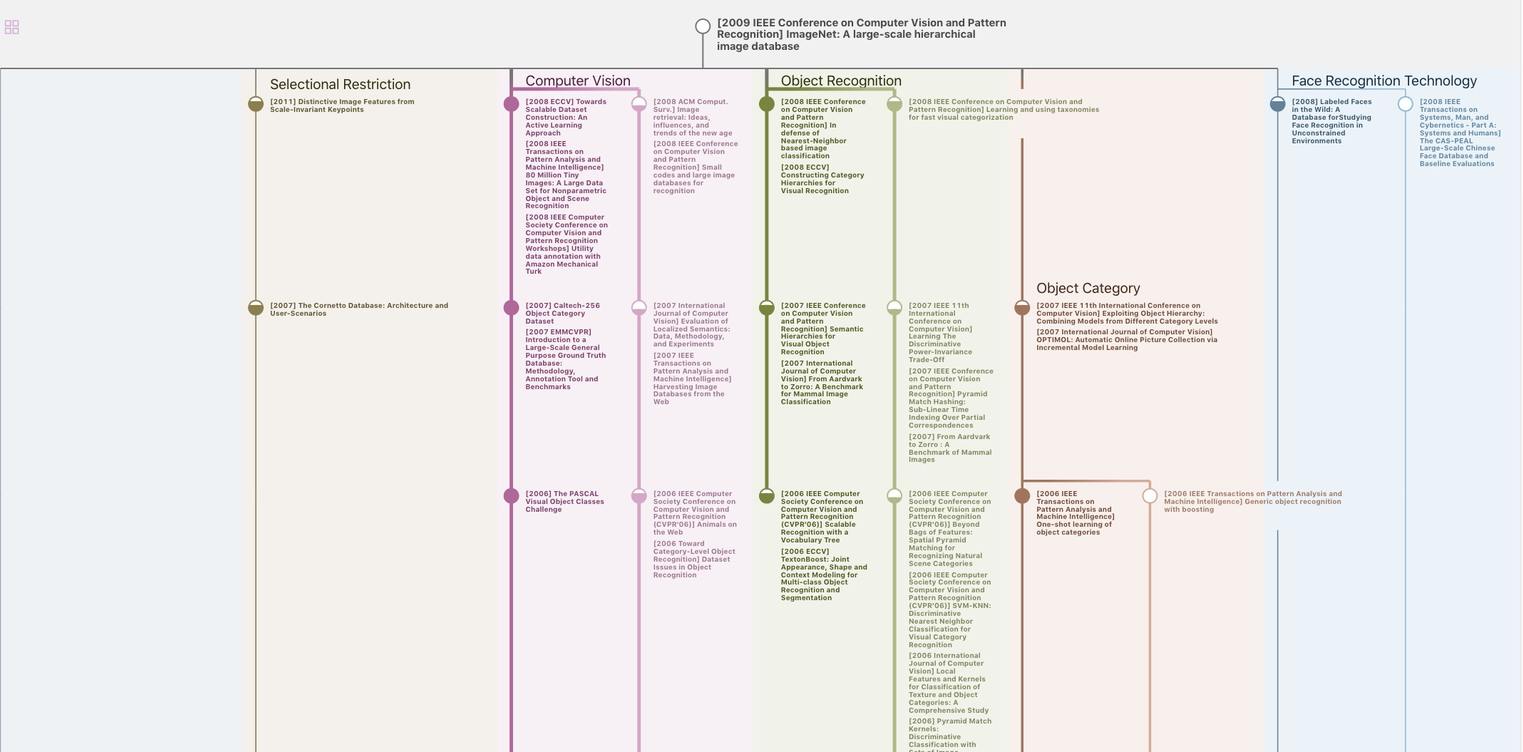
生成溯源树,研究论文发展脉络
Chat Paper
正在生成论文摘要