Constructing trading strategy ensembles by classifying market states
arXiv (Cornell University)(2020)
摘要
Rather than directly predicting future prices or returns, we follow a more recent trend in asset management and classify the state of a market based on labels. We use numerous standard labels and even construct our own ones. The labels rely on future data to be calculated, and can be used a target for training a market state classifier using an appropriate set of market features, e.g. moving averages. The construction of those features relies on their label separation power. Only a set of reasonable distinct features can approximate the labels. For each label we use a specific neural network to classify the state using the market features from our feature space. Each classifier gives a probability to buy or to sell and combining all their recommendations (here only done in a linear way) results in what we call a trading strategy. There are many such strategies and some of them are somewhat dubious and misleading. We construct our own metric based on past returns but penalising for a low number of transactions or small capital involvement. Only top score-performance-wise trading strategies end up in final ensembles. Using the Bitcoin market we show that the strategy ensembles outperform both in returns and risk-adjusted returns in the out-of-sample period. Even more so we demonstrate that there is a clear correlation between the success achieved in the past (if measured in our custom metric) and the future.
更多查看译文
关键词
trading strategy ensembles,market,states
AI 理解论文
溯源树
样例
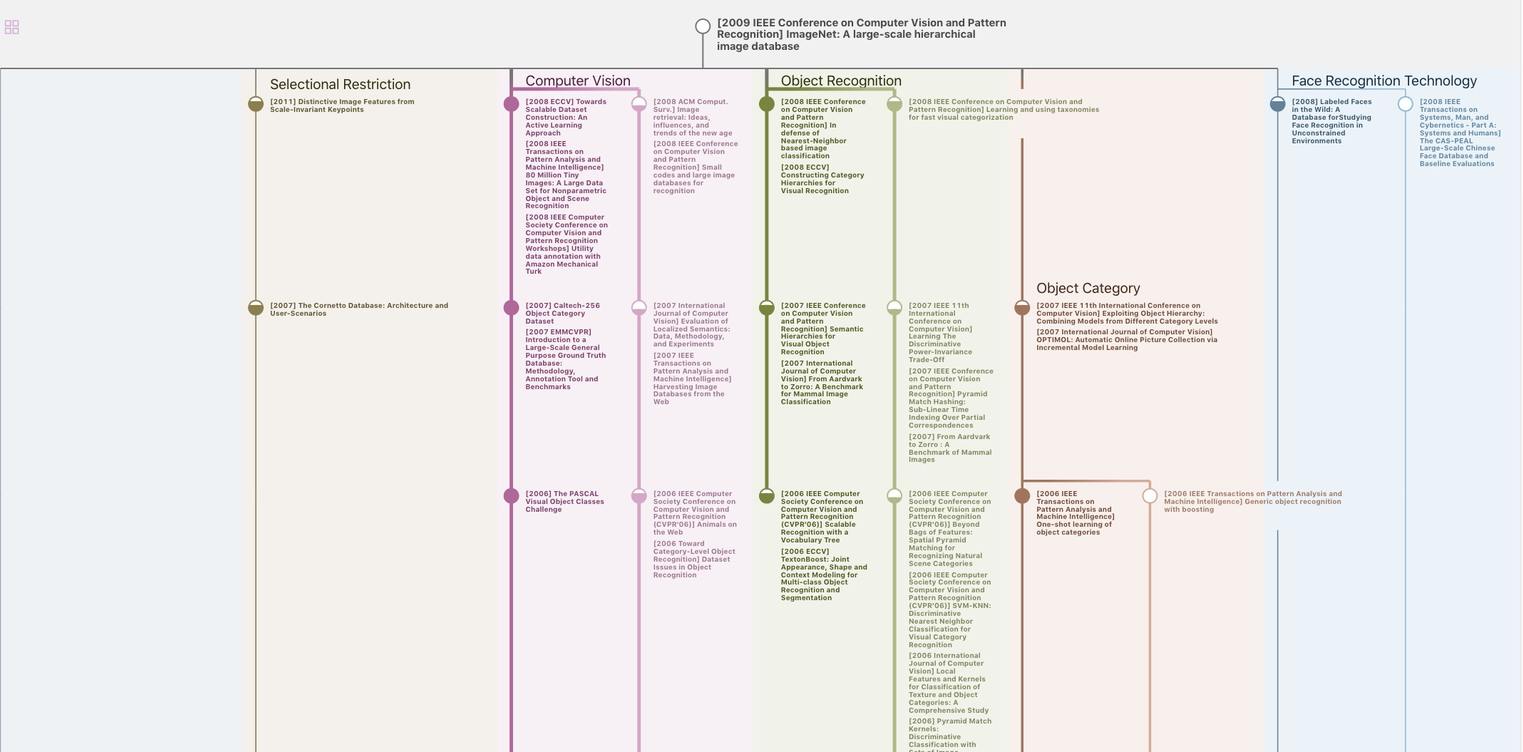
生成溯源树,研究论文发展脉络
Chat Paper
正在生成论文摘要