Group testing for overlapping communities
IEEE INTERNATIONAL CONFERENCE ON COMMUNICATIONS (ICC 2021)(2021)
摘要
In this paper, we propose algorithms that leverage a known community structure to make group testing more efficient. We consider a population organized in connected communities: each individual participates in one or more communities, and the infection probability of each individual depends on the communities (s)he participates in. Use cases include students who participate in several classes, and workers who share common spaces. Group testing reduces the number of tests needed to identify the infected individuals by pooling diagnostic samples and testing them together. We show that making testing algorithms aware of the community structure, can significantly reduce the number of tests needed both for adaptive and non-adaptive group testing.
更多查看译文
关键词
infected individuals,nonadaptive group testing,community structure,connected communities,adaptive group testing,pooling diagnostic samples,overlapping communities
AI 理解论文
溯源树
样例
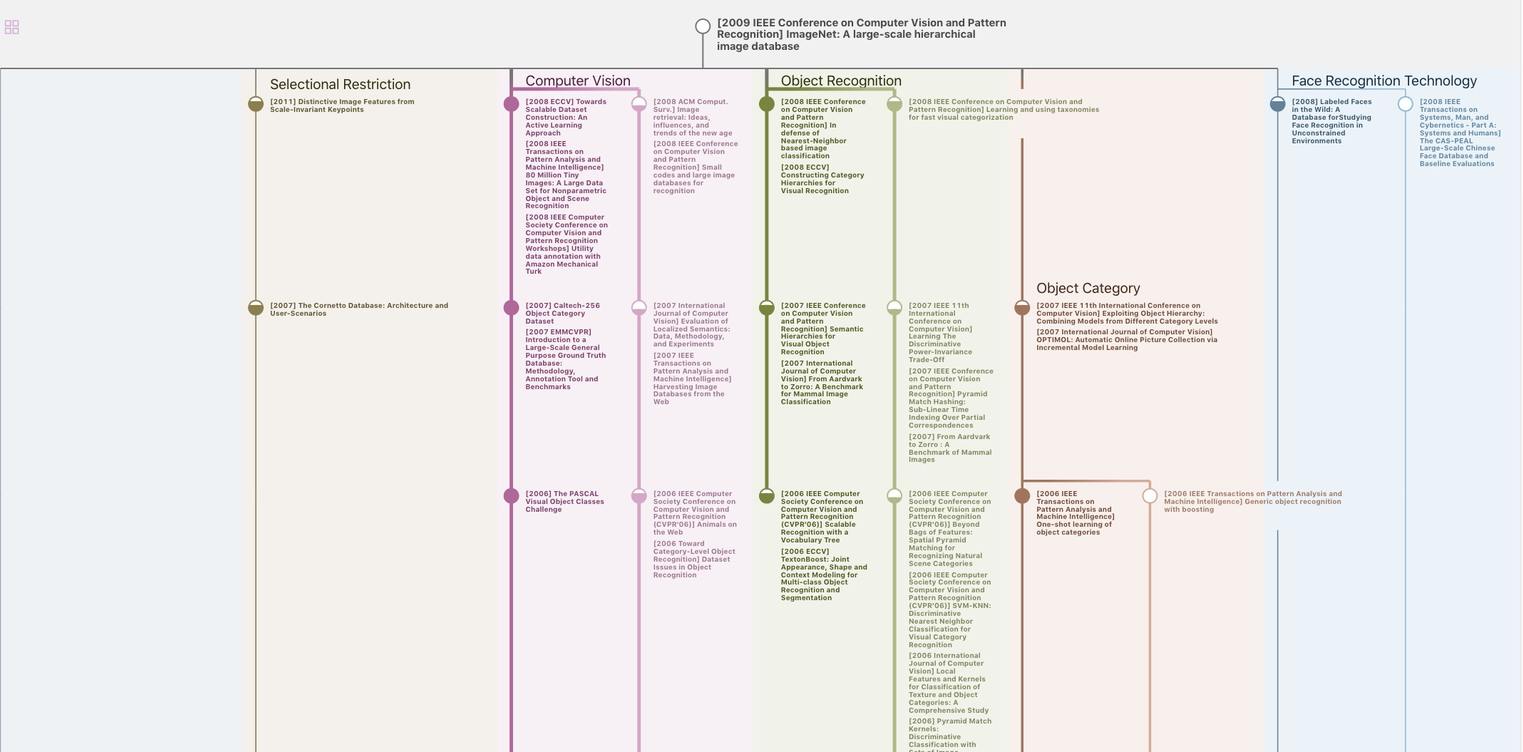
生成溯源树,研究论文发展脉络
Chat Paper
正在生成论文摘要