Mitigating Bias in Federated Learning
CoRR(2020)
Abstract
As methods to create discrimination-aware models develop, they focus on centralized ML, leaving federated learning (FL) unexplored. FL is a rising approach for collaborative ML, in which an aggregator orchestrates multiple parties to train a global model without sharing their training data. In this paper, we discuss causes of bias in FL and propose three pre-processing and in-processing methods to mitigate bias, without compromising data privacy, a key FL requirement. As data heterogeneity among parties is one of the challenging characteristics of FL, we conduct experiments over several data distributions to analyze their effects on model performance, fairness metrics, and bias learning patterns. We conduct a comprehensive analysis of our proposed techniques, the results demonstrating that these methods are effective even when parties have skewed data distributions or as little as 20% of parties employ the methods.
MoreTranslated text
Key words
federated learning,bias
AI Read Science
Must-Reading Tree
Example
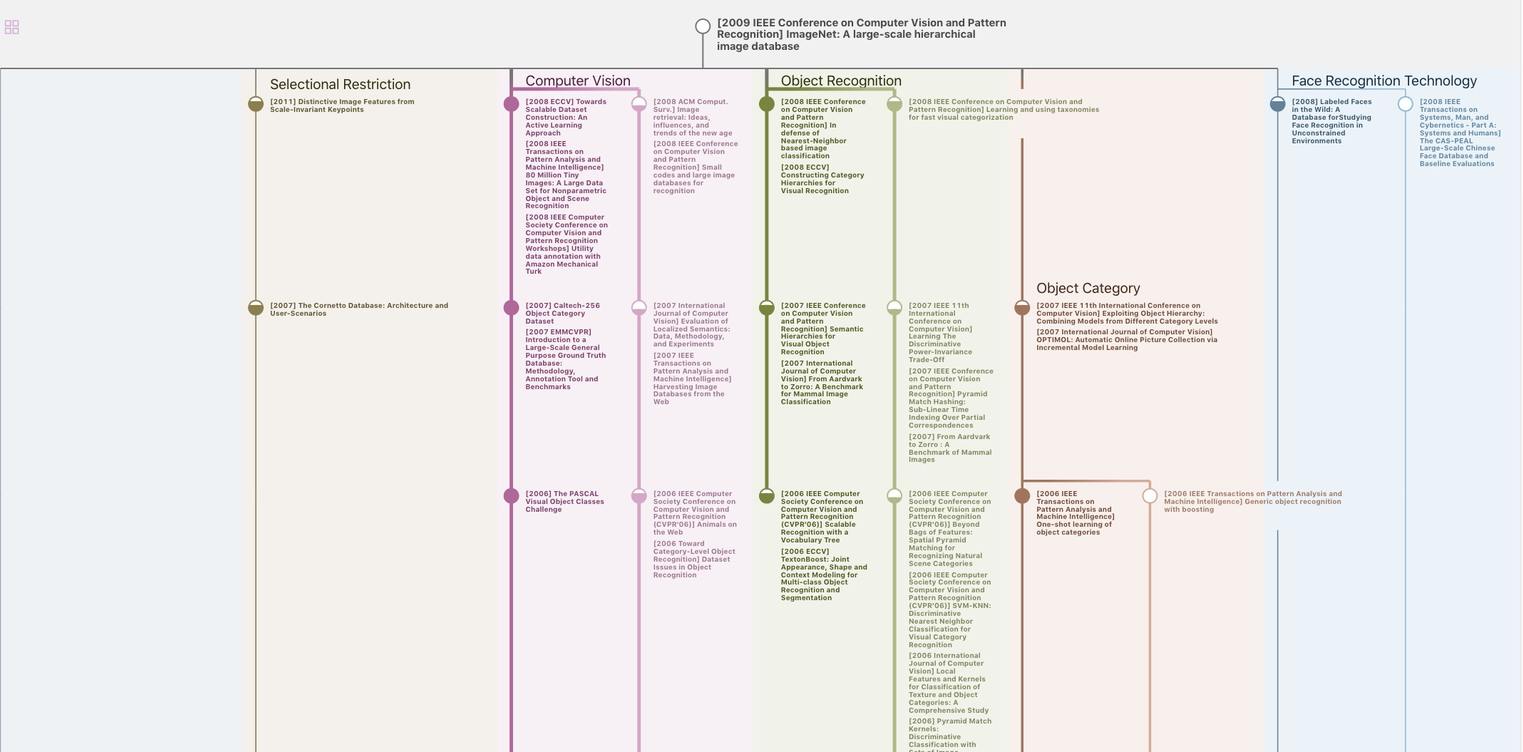
Generate MRT to find the research sequence of this paper
Chat Paper
Summary is being generated by the instructions you defined