Improved MVDR Beamforming Using LSTM Speech Models to Clean Spatial Clustering Masks
arxiv(2020)
摘要
Spatial clustering techniques can achieve significant multi-channel noise reduction across relatively arbitrary microphone configurations, but have difficulty incorporating a detailed speech/noise model. In contrast, LSTM neural networks have successfully been trained to recognize speech from noise on single-channel inputs, but have difficulty taking full advantage of the information in multi-channel recordings. This paper integrates these two approaches, training LSTM speech models to clean the masks generated by the Model-based EM Source Separation and Localization (MESSL) spatial clustering method. By doing so, it attains both the spatial separation performance and generality of multi-channel spatial clustering and the signal modeling performance of multiple parallel single-channel LSTM speech enhancers. Our experiments show that when our system is applied to the CHiME-3 dataset of noisy tablet recordings, it increases speech quality as measured by the Perceptual Evaluation of Speech Quality (PESQ) algorithm and reduces the word error rate of the baseline CHiME-3 speech recognizer, as compared to the default BeamformIt beamformer.
更多查看译文
关键词
improved mvdr beamforming,clean spatial clustering masks,lstm speech models
AI 理解论文
溯源树
样例
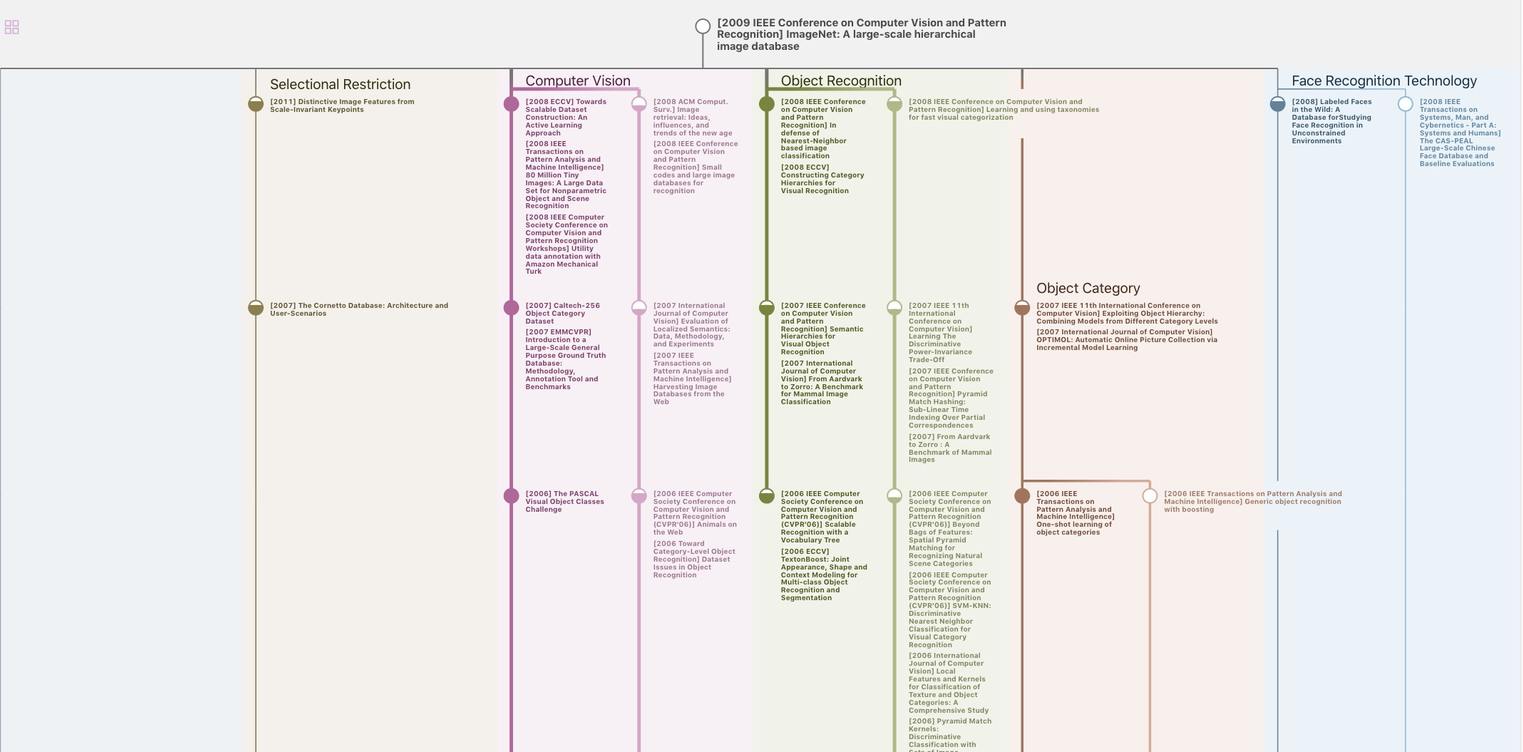
生成溯源树,研究论文发展脉络
Chat Paper
正在生成论文摘要