Coarse Raman and optical diffraction tomographic imaging enable label-free phenotyping of isogenic breast cancer cells of varying metastatic potential
Biosensors & bioelectronics(2021)
摘要
Identification of the metastatic potential represents one of the most important tasks for molecular imaging of cancer. While molecular imaging of metastases has witnessed substantial progress as an area of clinical inquiry, determining precisely what differentiates the metastatic phenotype has proven to be more elusive. In this study, we utilize both the morphological and molecular information provided by 3D optical diffraction tomography and Raman spectroscopy, respectively, to propose a label-free route for optical phenotyping of cancer cells at single-cell resolution. By using an isogenic panel of cell lines derived from MDA-MB-231 breast cancer cells that vary in their metastatic potential, we show that 3D refractive index tomograms can capture subtle morphological differences among the parental, circulating tumor cells, and lung metastatic cells. By leveraging its molecular specificity, we demonstrate that coarse Raman microscopy is capable of rapidly mapping a sufficient number of cells for training a random forest classifier that can accurately predict the metastatic potential of cells at a single-cell level. We also perform multivariate curve resolution alternating least squares decomposition of the spectral dataset to demarcate spectra from cytoplasm and nucleus, and test the feasibility of identifying metastatic phenotypes using the spectra only from the cytoplasmic and nuclear regions. Overall, our study provides a rationale for employing coarse Raman mapping to substantially reduce measurement time thereby enabling the acquisition of reasonably large training datasets that hold the key for label-free single-cell analysis and, consequently, for differentiation of indolent from aggressive phenotypes.
更多查看译文
关键词
Raman spectroscopy,Optical diffraction tomography,Breast cancer,Metastasis,Random forests,Single-cell phenotyping
AI 理解论文
溯源树
样例
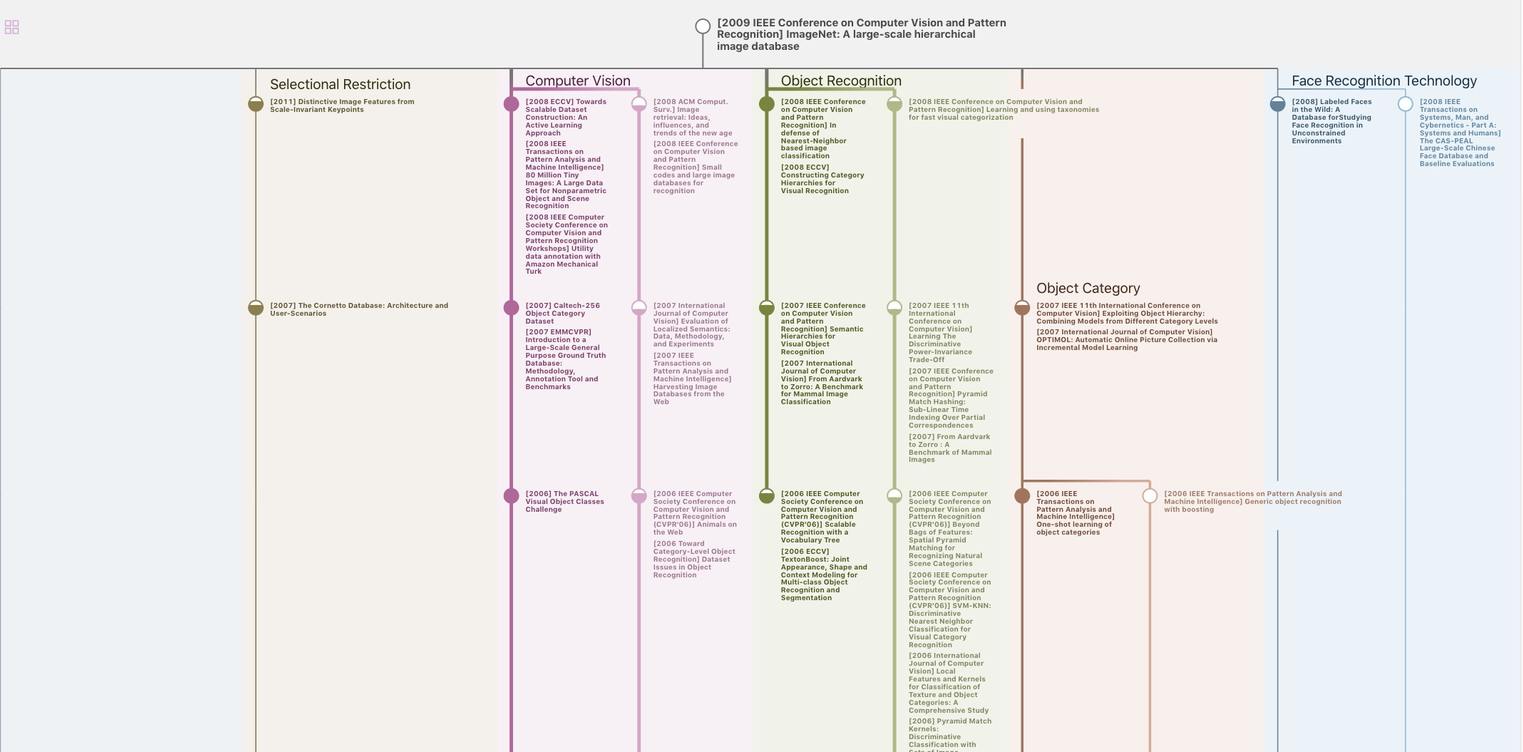
生成溯源树,研究论文发展脉络
Chat Paper
正在生成论文摘要