Robustly Learning Mixtures of k Arbitrary Gaussians
PROCEEDINGS OF THE 54TH ANNUAL ACM SIGACT SYMPOSIUM ON THEORY OF COMPUTING (STOC '22)(2022)
摘要
We give a polynomial-time algorithm for the problem of robustly estimating a mixture of k arbitrary Gaussians in R-d, for any fixed k, in the presence of a constant fraction of arbitrary corruptions. This resolves the main open problem in several previous works on algorithmic robust statistics, which addressed the special cases of robustly estimating (a) a single Gaussian, (b) a mixture of TV-distance separated Gaussians, and (c) a uniform mixture of two Gaussians. Our main tools are an efficient partial clustering algorithm that relies on the sum-of-squares method, and a novel tensor decomposition algorithm that allows errors in both Frobenius norm and low-rank terms.
更多查看译文
关键词
Gaussian Mixture Model, Robust Statistics, Tensor Decomposition, Sum-of-Squares
AI 理解论文
溯源树
样例
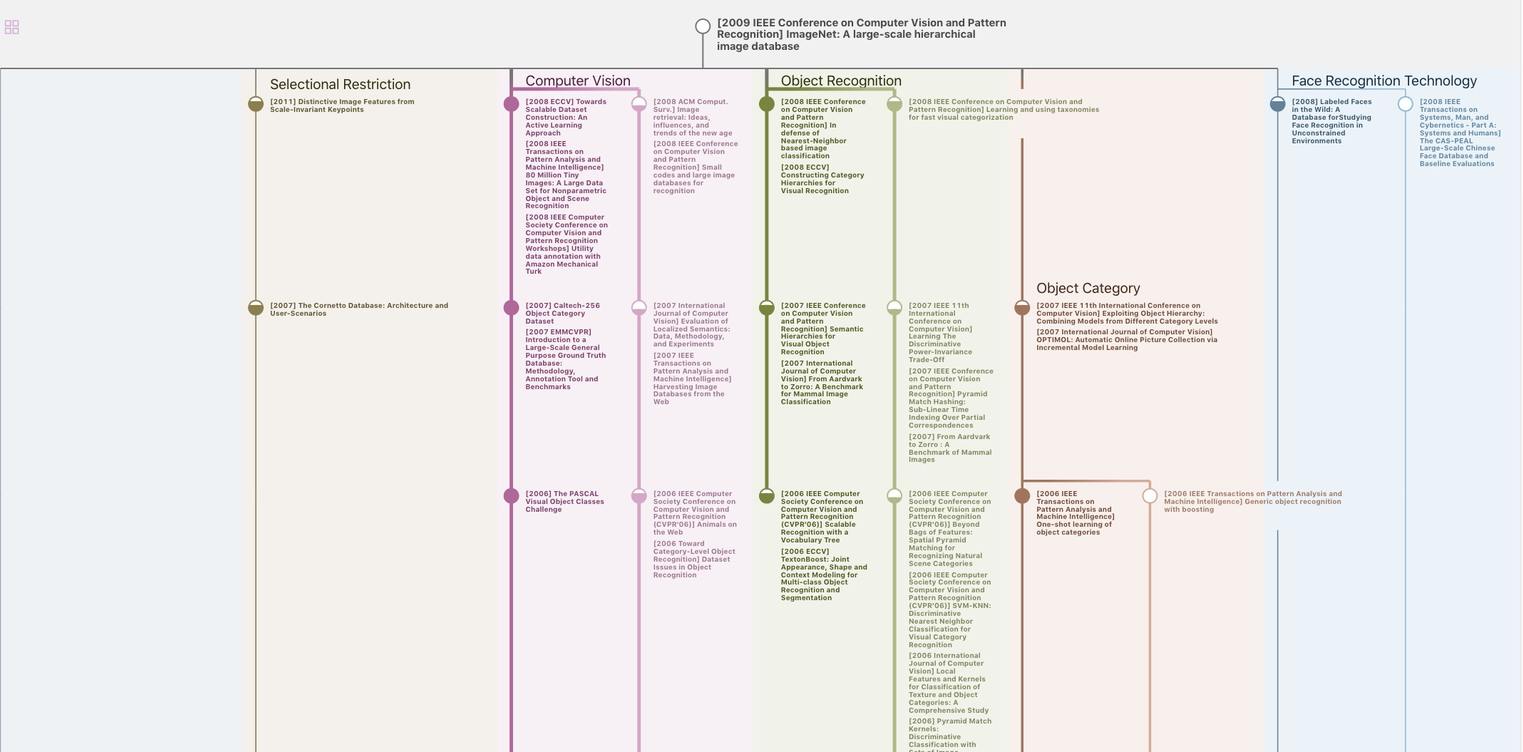
生成溯源树,研究论文发展脉络
Chat Paper
正在生成论文摘要