Enhancement of Spatial Clustering-Based Time-Frequency Masks using LSTM Neural Networks
arxiv(2020)
摘要
Recent works have shown that Deep Recurrent Neural Networks using the LSTM architecture can achieve strong single-channel speech enhancement by estimating time-frequency masks. However, these models do not naturally generalize to multi-channel inputs from varying microphone configurations. In contrast, spatial clustering techniques can achieve such generalization but lack a strong signal model. Our work proposes a combination of the two approaches. By using LSTMs to enhance spatial clustering based time-frequency masks, we achieve both the signal modeling performance of multiple single-channel LSTM-DNN speech enhancers and the signal separation performance and generality of multi-channel spatial clustering. We compare our proposed system to several baselines on the CHiME-3 dataset. We evaluate the quality of the audio from each system using SDR from the BSS\_eval toolkit and PESQ. We evaluate the intelligibility of the output of each system using word error rate from a Kaldi automatic speech recognizer.
更多查看译文
关键词
lstm neural networks,neural networks,clustering-based,time-frequency
AI 理解论文
溯源树
样例
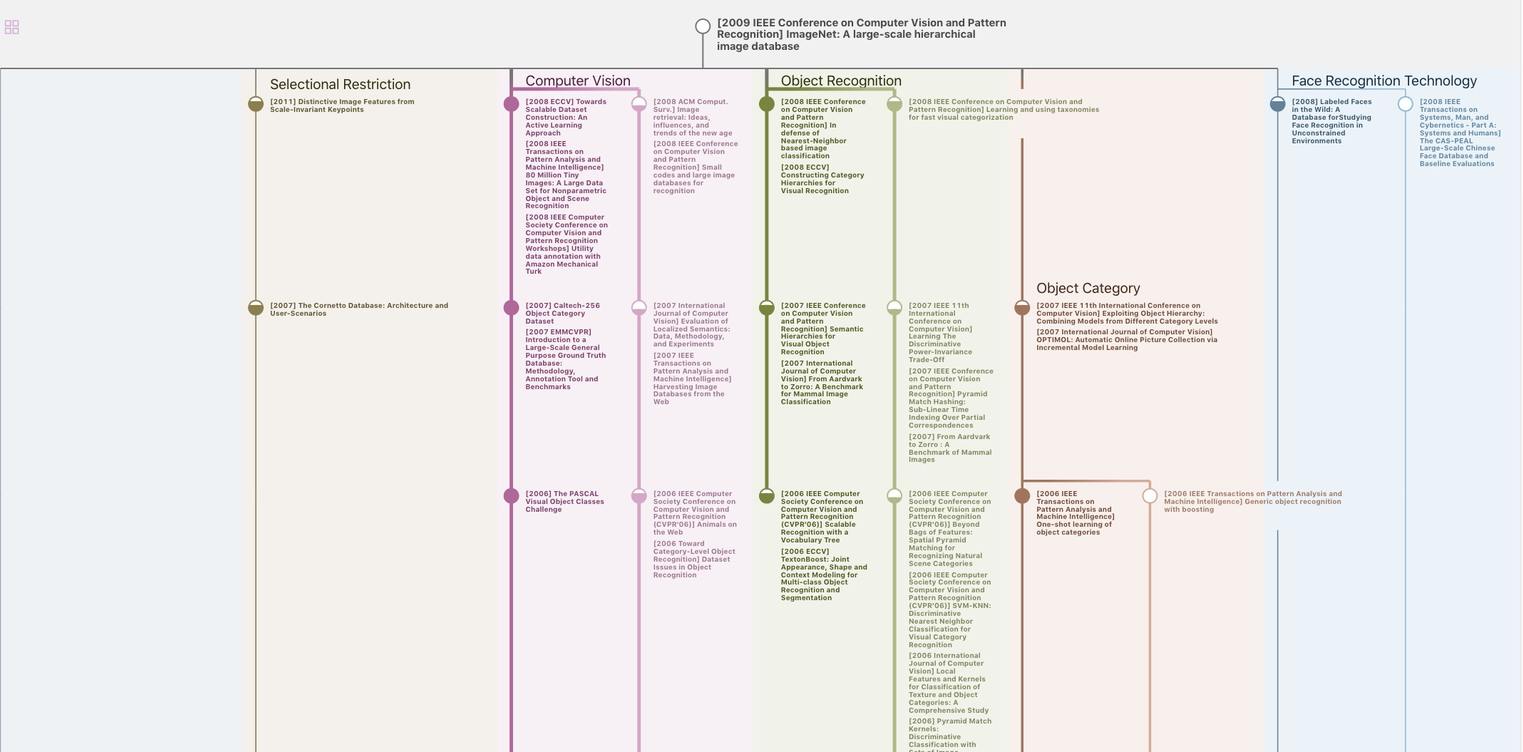
生成溯源树,研究论文发展脉络
Chat Paper
正在生成论文摘要