Machine Learning Augmented Time-Lapse Bathymetric Surveys: A Case Study From the Mississippi River Delta Front
GEOPHYSICAL RESEARCH LETTERS(2020)
Abstract
The subaqueous Mississippi River Delta Front is prone to seabed instabilities >1 m of vertical bathymetric change per year, but the ability to predict the location and magnitude of instability-driven depth change is limited. Here we demonstrate that data-driven geospatial models can predict MRDF depth change from a small amount (1% of full coverage) of training data. We predict depth change at 100 m(2) resolution between 2005 and 2017 over a similar to 100 km(2) area. Models trained on similar to 1% of full-coverage depth change data produce comparable and relatively low average predicted depth change errors (1-2 cm). K-nearest neighbors best reproduce the spatial variability of depth change and can interpolate and extrapolate from training data. This approach has immediate applications for geohazard monitoring on the MRDF and other geologically similar settings and can be applied in other settings if the drivers of depth change variance are well known.
MoreTranslated text
Key words
bathymetry,geohazards,machine learning,deltas,depth change
AI Read Science
Must-Reading Tree
Example
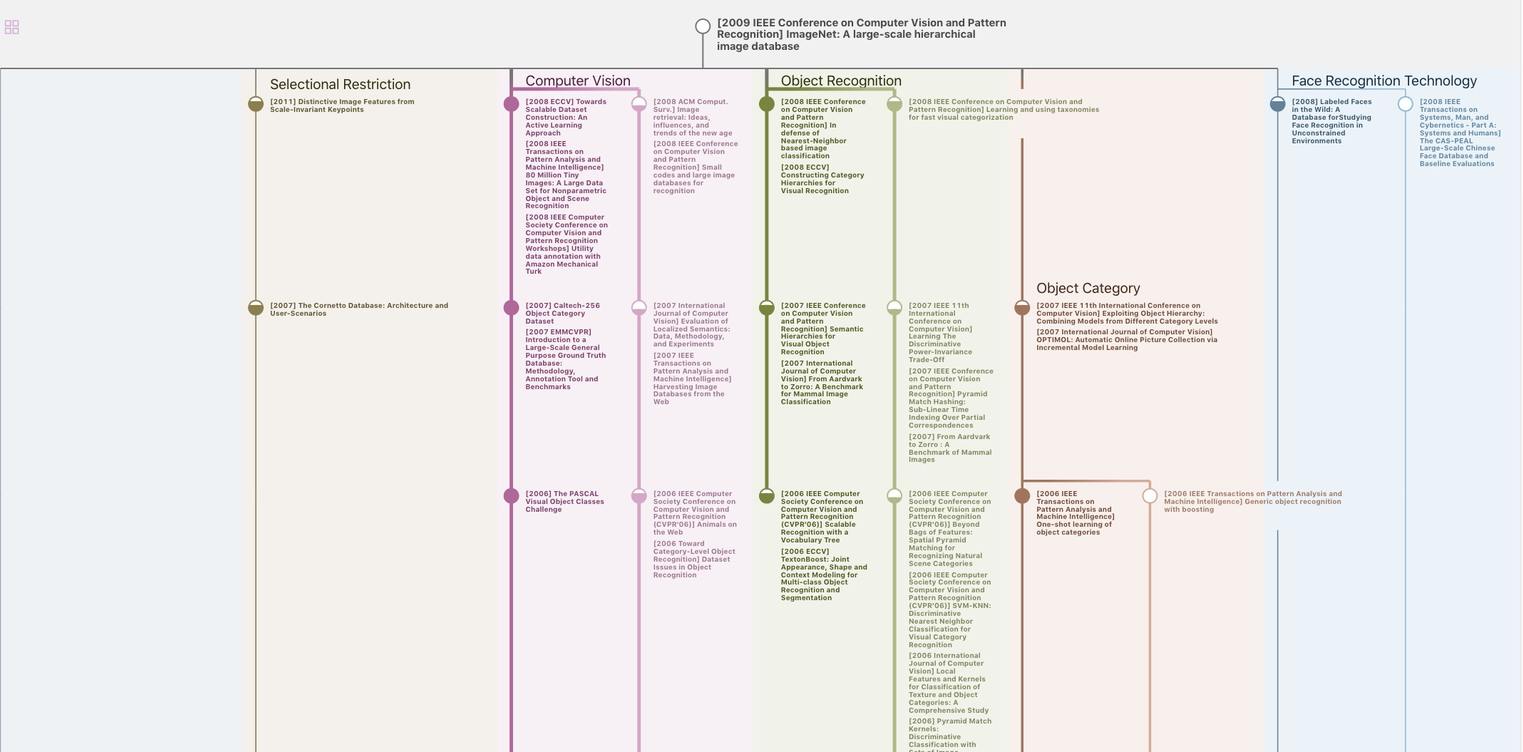
Generate MRT to find the research sequence of this paper
Chat Paper
Summary is being generated by the instructions you defined