Statistical models are able to predict ionic liquid viscosity across a wide range of chemical functionalities and experimental conditions
MOLECULAR SYSTEMS DESIGN & ENGINEERING(2018)
Abstract
Herein we present a method of developing predictive models of viscosity for ionic liquids (ILs) using publicly available data in the ILThermo database and the open-source software toolkits PyChem, RDKit, and SciKit-Learn. The process consists of downloading similar to 700 datapoints from ILThermo, generating similar to 1200 physiochemical features with PyChem and RDKit, selecting 11 features with the least absolute shrinkage selection operator (LASSO) method, and using the selected features to train a multi-layer perceptron regressor-a class of feedforward artificial neural network (ANN). The interpretability of the LASSO model allows a physical interpretation of the model development framework while the flexibility and non-linearity of the hidden layer of the ANN optimizes performance. The method is tested on a range of temperatures, pressures, and viscosities to evaluate its efficacy in a general-purpose setting. The model was trained on 578 datapoints including a temperature range of 273.15-373.15 K, pressure range of 60-160 kPa, viscosity range of 0.0035-0.993 Pa s, and ILs of imidazolium, phosphonium, pyridinium, and pyrrolidinium classes to give 33 different salts altogether. The model had a validation set mean squared error of 4.7 x 10(-4) +/- 2.4 x 10(-5) Pa s or relative absolute average deviation of 7.1 +/- 1.3%.
MoreTranslated text
Key words
ionic liquid viscosity,chemical functionalities,statistical models
AI Read Science
Must-Reading Tree
Example
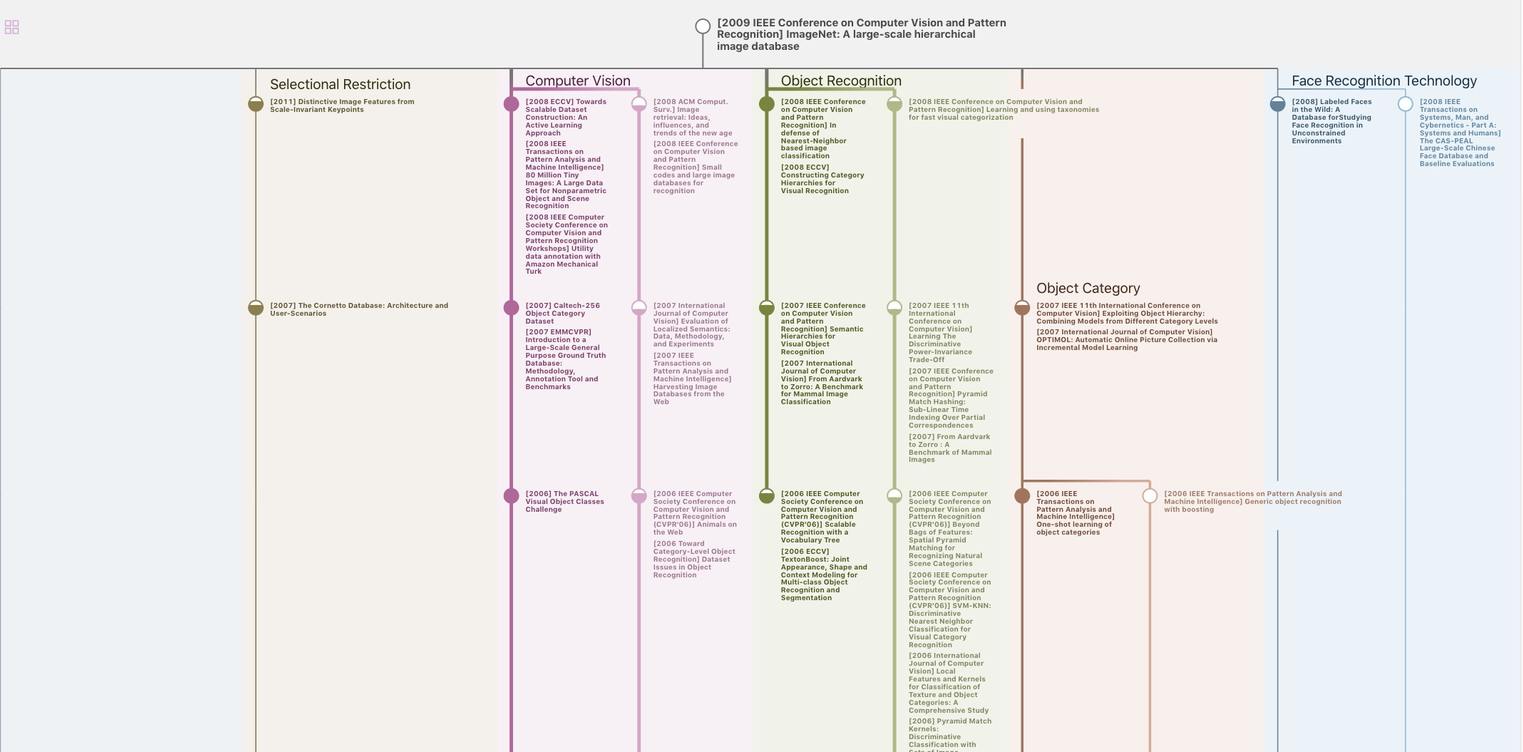
Generate MRT to find the research sequence of this paper
Chat Paper
Summary is being generated by the instructions you defined