Predicting the Electrical Energy Consumption of Electric Arc Furnaces Using Statistical Modeling
METALS(2019)
摘要
Statistical modeling, also known as machine learning, has gained increased attention in part due to the Industry 4.0 development. However, a review of the statistical models within the scope of steel processes has not previously been conducted. This paper reviews available statistical models in the literature predicting the Electrical Energy (EE) consumption of the Electric Arc Furnace (EAF). The aim was to structure published data and to bring clarity to the subject in light of challenges and considerations that are imposed by statistical models. These include data complexity and data treatment, model validation and error reporting, choice of input variables, and model transparency with respect to process metallurgy. A majority of the models are never tested on future heats, which essentially renders the models useless in a practical industrial setting. In addition, nonlinear models outperform linear models but lack transparency with regards to which input variables are influencing the EE consumption prediction. Some input variables that heavily influence the EE consumption are rarely used in the models. The scrap composition and additive materials are two such examples. These observed shortcomings have to be correctly addressed in future research applying statistical modeling on steel processes. Lastly, the paper provides three key recommendations for future research applying statistical modeling on steel processes.
更多查看译文
关键词
electrical energy consumption,Electric Arc Furnace,statistical modeling,machine learning
AI 理解论文
溯源树
样例
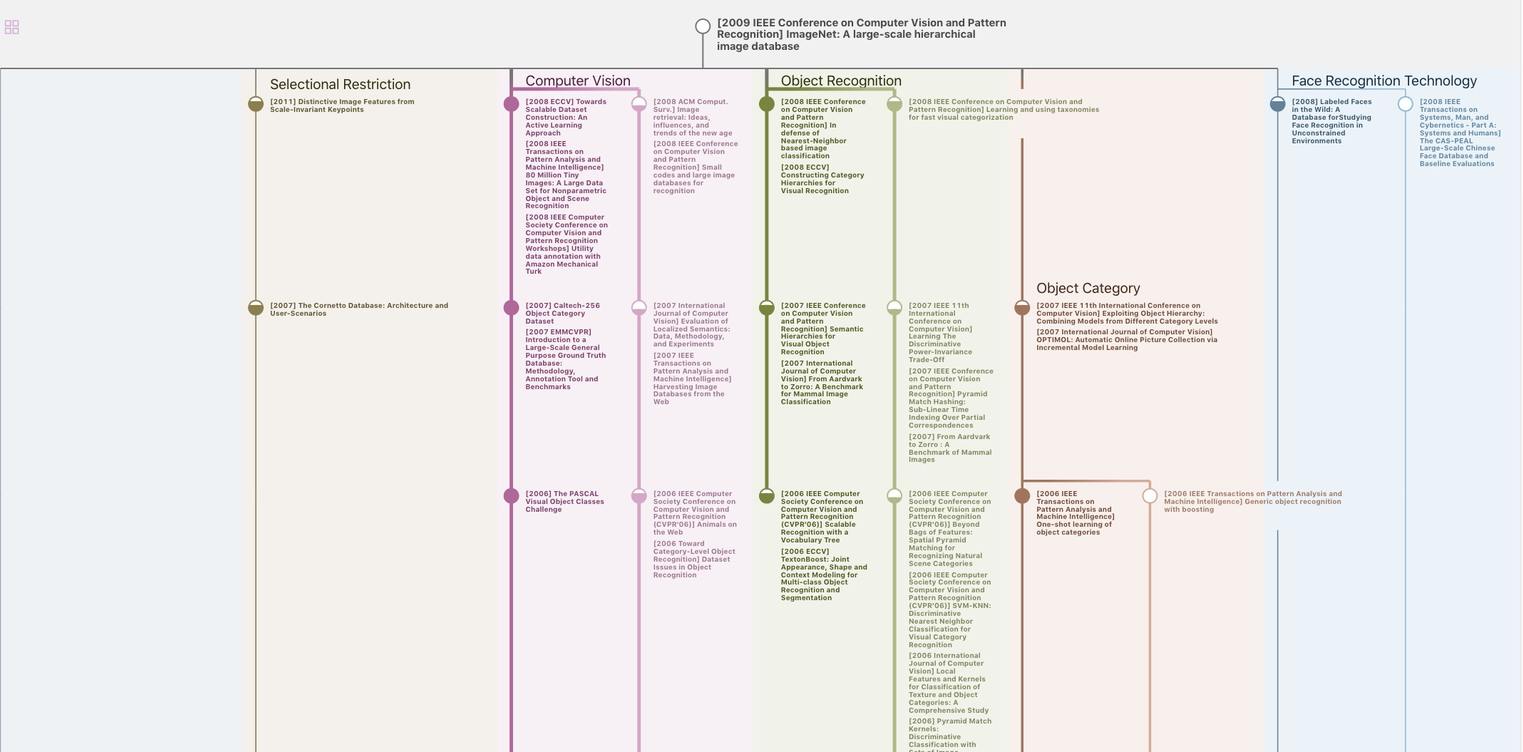
生成溯源树,研究论文发展脉络
Chat Paper
正在生成论文摘要