Combination of Multiple Neural Networks Using Transfer Learning and Extensive Geometric Data Augmentation for Assessing Cellularity Scores in Histopathology Images
MEDICAL PHYSICS(2019)
摘要
Classification of cancer cellularity within tissue samples is currently a manual process performed by pathologists. This process of correctly determining cancer cellularity can be time intensive. Deep Learning (DL) techniques in particular have become increasingly more popular for this purpose, due to the accuracy and performance they exhibit, which can be comparable to the pathologists. This work investigates the capabilities of two DL approaches to assess cancer cellularity in whole slide images (WSI) in the SPIE-AAPM-NCI BreastPathQ challenge dataset. The effects of training on augmented data via rotations, and combinations of multiple architectures into a single network were analyzed using a modified Kendall Tau-b prediction probability metric known as the average prediction probability PK. A deep, transfer learned, Convolutional Neural Network (CNN) InceptionV3 was used as a baseline, achieving an average PK value of 0.884, showing improvement from the average PK value of 0.83 achieved by pathologists. The network was then trained on additional training datasets which were rotated between 1 and 360 degrees, which saw a peak increase of PK up to 4.2%. An additional architecture consisting of the InceptionV3 network and VGG16, a shallow, transfer learned CNN, was combined in a parallel architecture. This parallel architecture achieved a baseline average PK value of 0.907, a statistically significantly improvement over either of the architectures' performances separately (p<0.0001 by unpaired t-test).
更多查看译文
关键词
histopathology images,cellularity scores,extensive geometric data augmentation,transfer learning
AI 理解论文
溯源树
样例
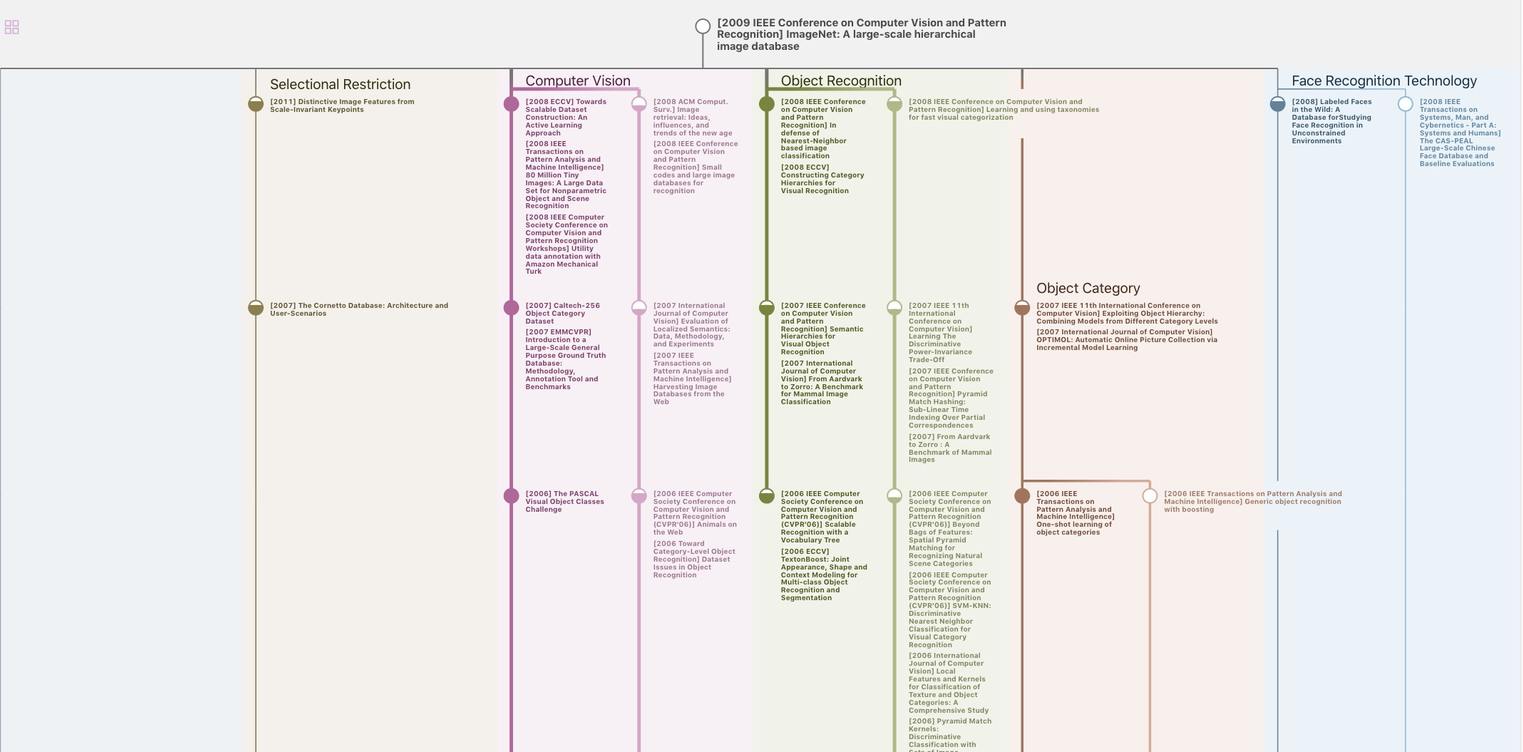
生成溯源树,研究论文发展脉络
Chat Paper
正在生成论文摘要