A Computational Approach to Bounding the Mixing Time of a Metropolis - Hastings Sampler
MARKOV PROCESSES AND RELATED FIELDS(2020)
摘要
Markov chain Monte Carlo (MCMC) methods are commonly used to obtain approximate samples from intractable probability distributions. Any time an MCMC algorithm is used, it is important to be able to obtain a sense of how long the underlying Markov chain takes to get close to its stationary distribution. While several theory-based approaches to obtaining such information exist in the literature, many of them are difficult to use in practice. As an alternative, the literature is rich with techniques for assessing convergence based on the output of the chain. These techniques suffer from their own limitations, so this work presents a computational approach to estimating an upper bound on the mixing time of the chain based on auxiliary simulations. Specifically, this work estimates the drift and minorization coefficients for random-walk Metropolis algorithms that explore R-m, and it provides a description of how the methods contained herein may be applied to other settings.
更多查看译文
关键词
random-walk Metropolis,mixing time,convergence,Metropolis-Hastings algorithm,Lyapunov conditions
AI 理解论文
溯源树
样例
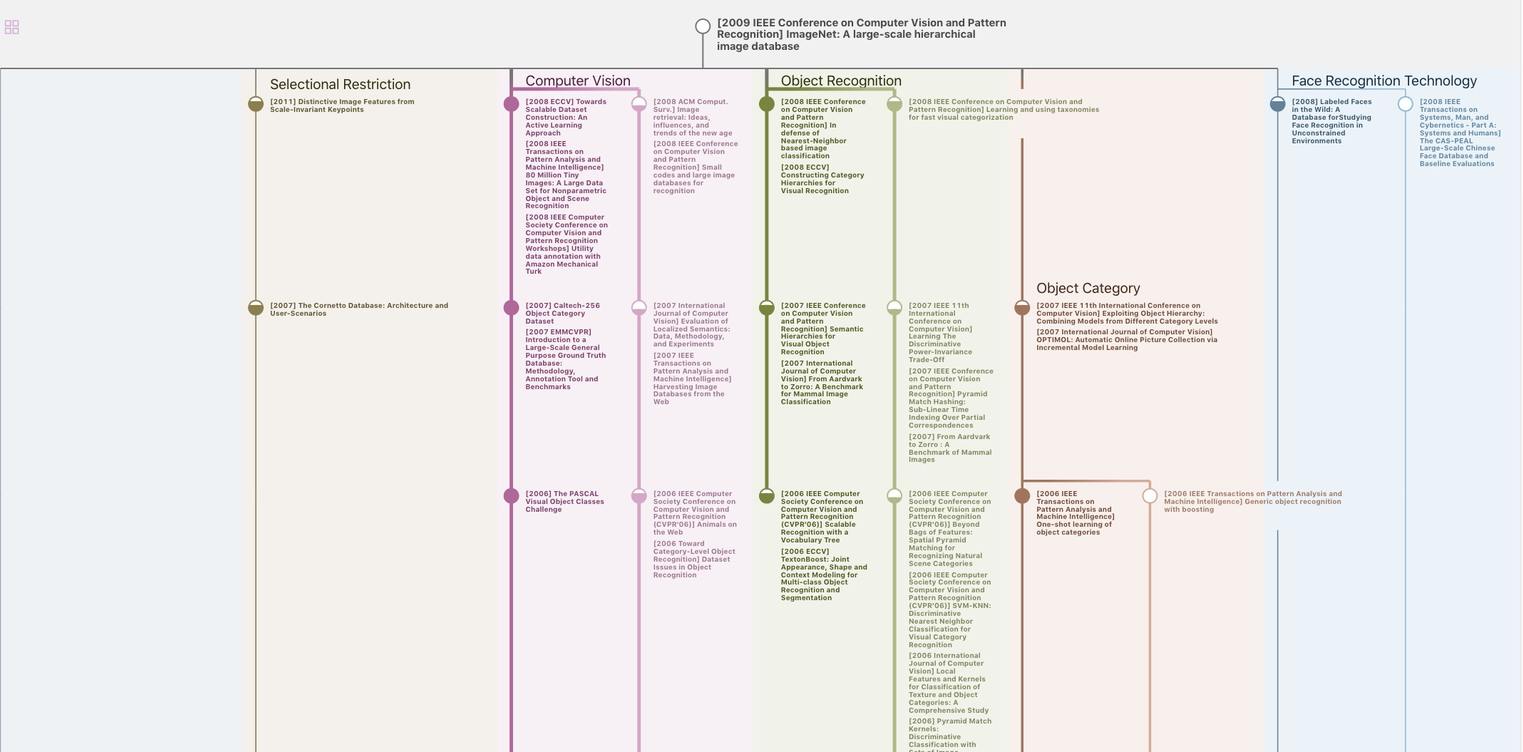
生成溯源树,研究论文发展脉络
Chat Paper
正在生成论文摘要