A Distributed Threshold Additive Homomorphic Encryption for Federated Learning with Dropout Resiliency Based on Lattice
CYBERSPACE SAFETY AND SECURITY, CSS 2022(2022)
摘要
In federated learning, a parameter server needs to aggregate user gradients and a user needs the privacy of their individual gradients. Among all the possible solutions, additive homomorphic encryption is a natural choice. As users may drop out during a federated learning process, and an adversary could corrupt users and the parameter server, a dropout-resilient scheme with distributed key generation is required. We present a lattice based distributed threshold additive homomorphic encryption scheme with provable security that could be used in the federated learning. The evaluation shows that our proposal has a lower communication overhead among all lattice based proposals when the number of users in FL exceeds 26.
更多查看译文
关键词
Federated learning,Privacy protection,Additive homomorphic encryption
AI 理解论文
溯源树
样例
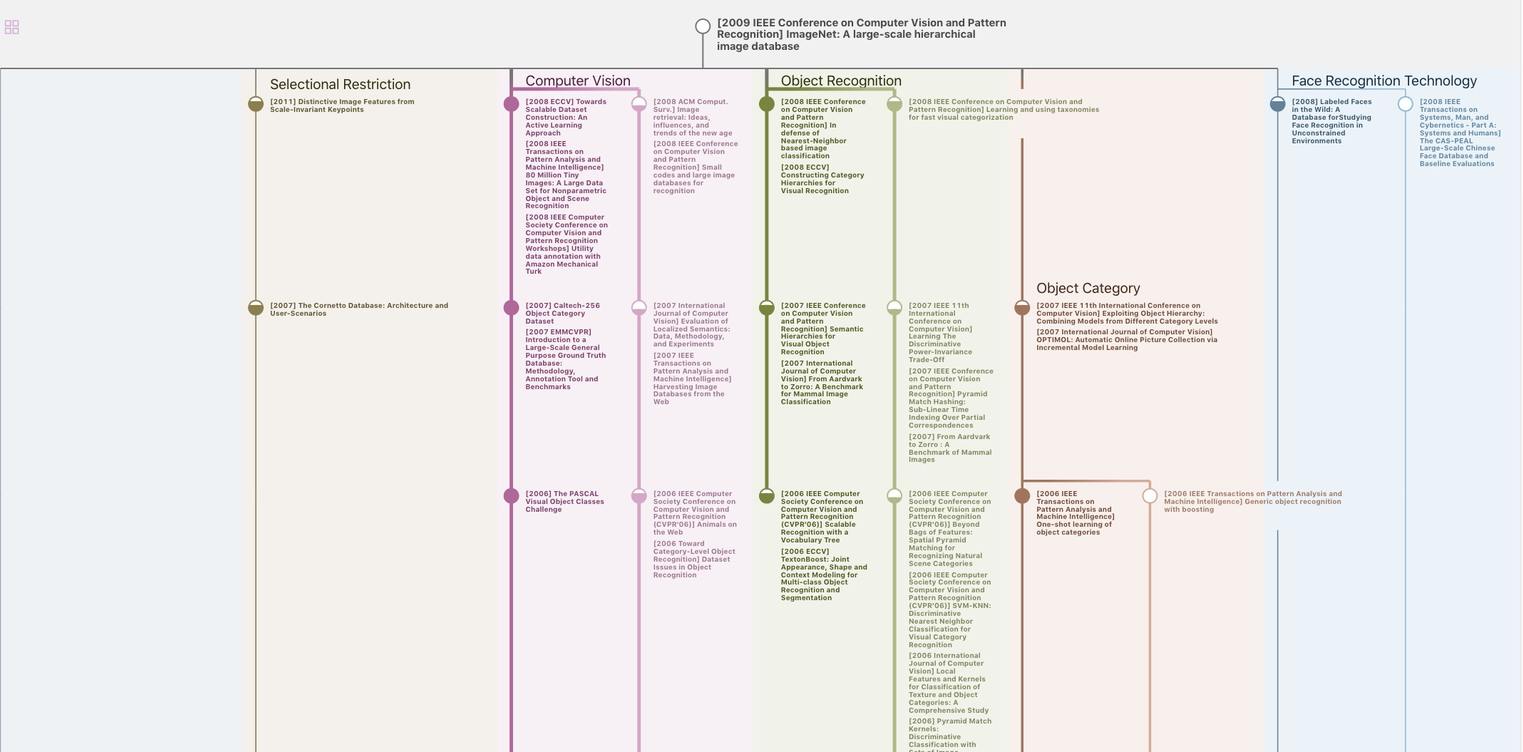
生成溯源树,研究论文发展脉络
Chat Paper
正在生成论文摘要