Quantum-Inspired Classical Algorithm for Slow Feature Analysis
CoRR(2020)
摘要
Recently, there has been a surge of interest for quantum computation for its ability to exponentially speed up algorithms, including machine learning algorithms. However, Tang suggested that the exponential speed up can also be done on a classical computer. In this paper, we proposed an algorithm for slow feature analysis, a machine learning algorithm that extracts the slow-varying features, with a run time O(polylog(n)poly(d)). To achieve this, we assumed necessary preprocessing of the input data as well as the existence of a data structure supporting a particular sampling scheme. The analysis of algorithm borrowed results from matrix perturbation theory, which was crucial for the algorithm's correctness. This work demonstrates the possible application and extent for which quantum-inspired computation can be used.
更多查看译文
关键词
algorithm,quantum-inspired
AI 理解论文
溯源树
样例
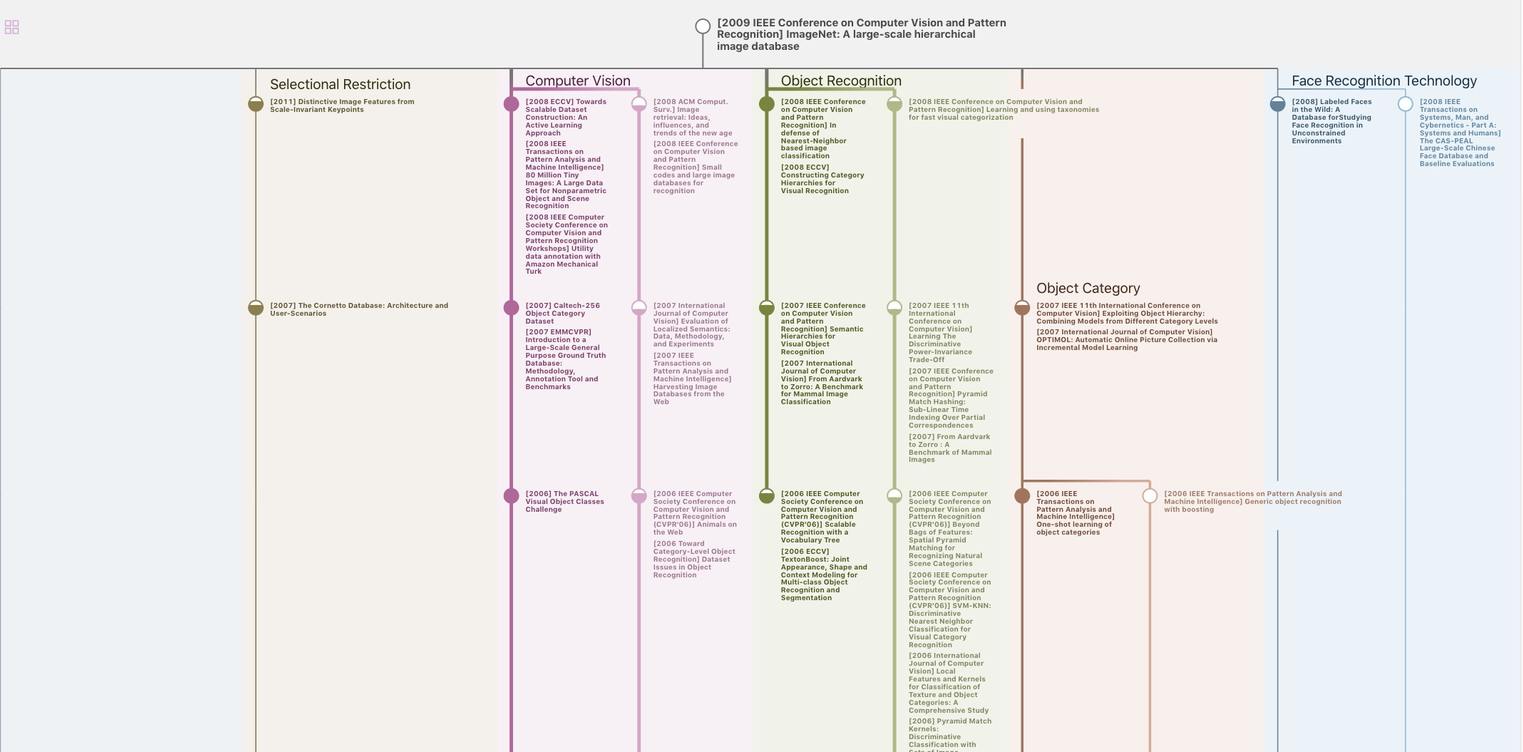
生成溯源树,研究论文发展脉络
Chat Paper
正在生成论文摘要