New Feature Selection Methods Using Sparse Representation for One-Class Classification of Remote Sensing Images
IEEE GEOSCIENCE AND REMOTE SENSING LETTERS(2021)
Abstract
In this letter, we proposed two novel feature selection methods using sparse representation for one-class classification of remote sensing images. In the first method, a sparse reconstructive weight matrix of the data set was obtained by reconstructing samples using sparse representation. The "good" features were then selected by evaluating reconstructing errors in weight matrix. The method is called feature selection based on sample reconstruction (FSSR). In the second method, the weight matrix was obtained by reconstructing features using sparse representation. Feature selection was then conducted by evaluating correlation among features using weight matrix. The method is called feature selection based on feature reconstruction (FSFR). The proposed feature selection methods were evaluated and compared with several state-of-the-art feature selection methods in two different case studies. The experimental results indicate that the proposed methods generally outperformed the existing methods. In particular, FSFR produced stable better performance.
MoreTranslated text
Key words
Feature extraction,Training,Sparse matrices,Image reconstruction,Hyperspectral imaging,Feature selection,one-class classification (OCC),sparse representation
AI Read Science
Must-Reading Tree
Example
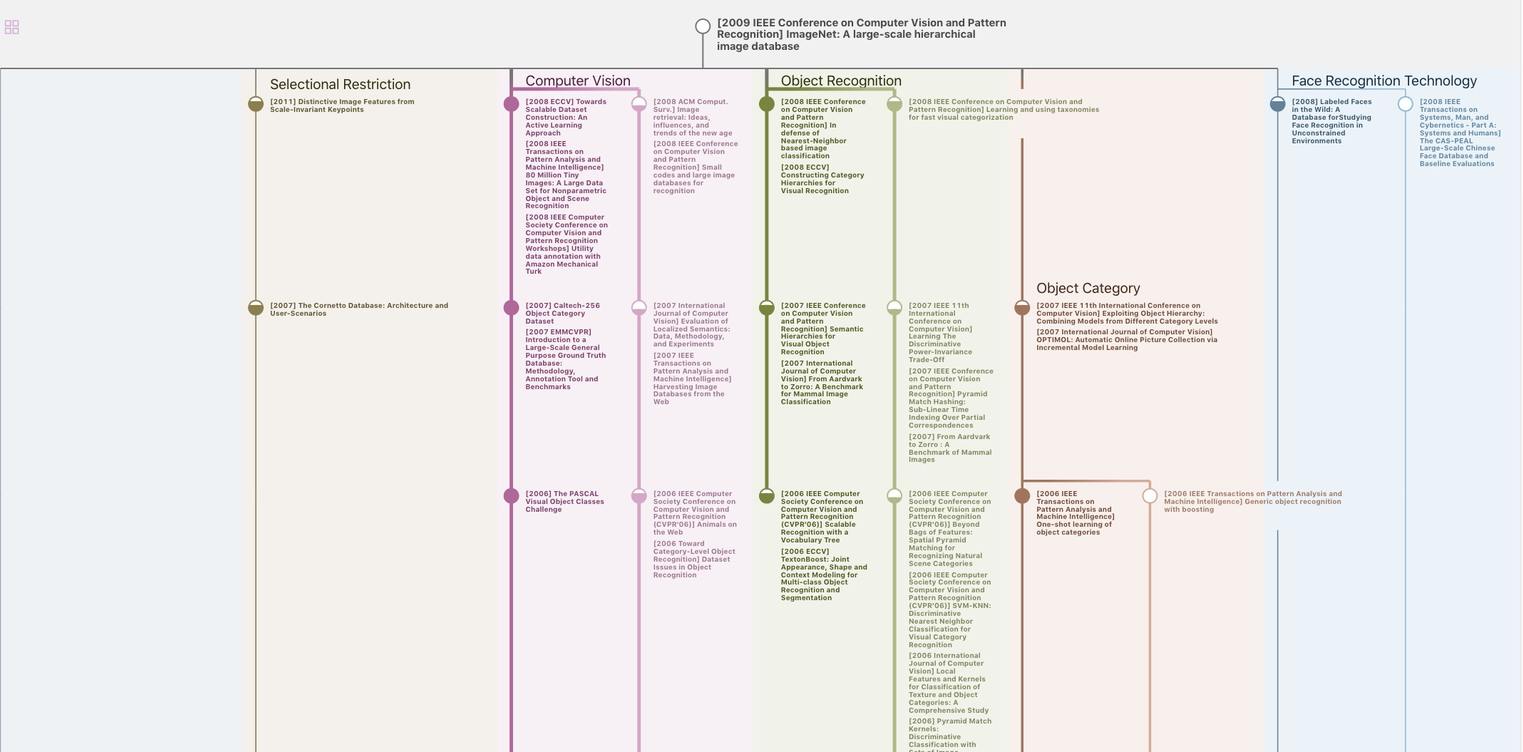
Generate MRT to find the research sequence of this paper
Chat Paper
Summary is being generated by the instructions you defined