Best Practices in Active Learning for Semantic Segmentation
PATTERN RECOGNITION, DAGM GCPR 2023(2024)
摘要
Active learning is particularly of interest for semantic segmentation, where annotations are costly. Previous academic studies focused on datasets that are already very diverse and where the model is trained in a supervised manner with a large annotation budget. In contrast, data collected in many driving scenarios is highly redundant, and most medical applications are subject to very constrained annotation budgets. This work investigates the various types of existing active learning methods for semantic segmentation under diverse conditions across three dimensions - data distribution w.r.t. different redundancy levels, integration of semi-supervised learning, and different labeling budgets. We find that these three underlying factors are decisive for the selection of the best active learning approach. As an outcome of our study, we provide a comprehensive usage guide to obtain the best performance for each case. It is the first systematic study that investigates these dimensions covering a wide range of settings including more than 3K model training runs. In this work, we also propose an exemplary evaluation task for driving scenarios, where data has high redundancy, to showcase the practical implications of our research findings.
更多查看译文
关键词
Active Learning,Semantic Segmentation
AI 理解论文
溯源树
样例
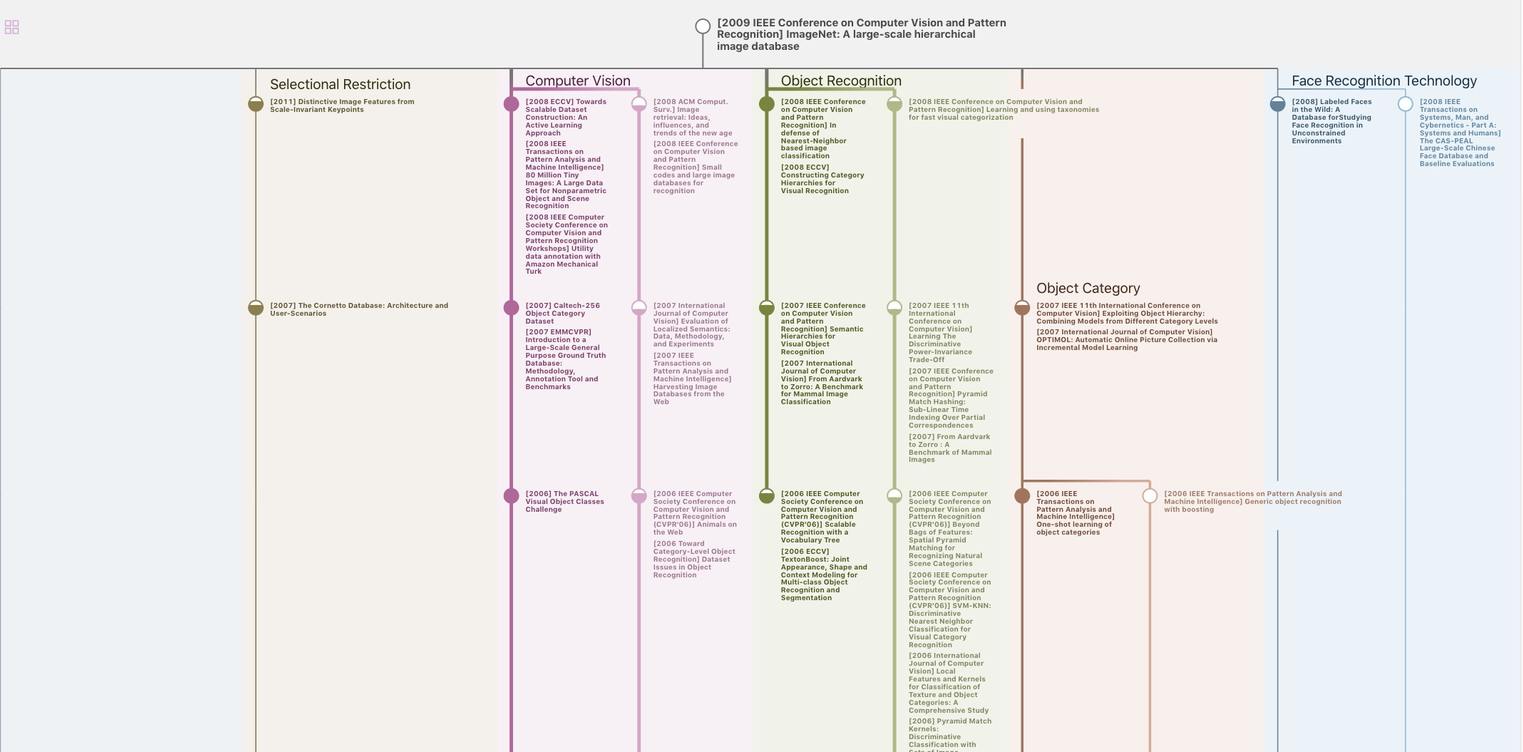
生成溯源树,研究论文发展脉络
Chat Paper
正在生成论文摘要