Mid-season county-level corn yield forecast for US Corn Belt integrating satellite imagery and weather variables
CROP SCIENCE(2020)
摘要
Yield estimations are of great interest to support interventions from governmental policies and to increase global food security. This study presents a novel model to perform in-season corn yield predictions at the US-county level, providing robust results under different weather and yield levels. The objectives of this study were to: (i) evaluate the performance of a random forest classification to identify corn fields using Normalized Difference Vegetation Index (NDVI), Enhanced Vegetation Index (EVI) and weather variables (temperature, precipitation, and vapor pressure deficit, VPD); (ii) evaluate the contribution of weather variables when forecasting corn yield via remote sensing data, and perform a sensitivity analysis to explore the model performance in different dates; and (iii) develop a model pipeline for performing in-season corn yield predictions at county-scale. Main outcomes from this study were: (i) high accuracy (87% on average) for corn field classification achieved in late August, (ii) corn yield forecasts with a mean absolute error (MAE) of 0.89 Mg ha(-1), (iii) weather variables (VPD and temperature) highly influenced the model performance, and (iv) model performance decreased when predictions were performed early in the season (mid-July), with MAE increasing from 0.87-1.36 Mg ha(-1) when forecast timing changed from day of year 232-192. This research portrays the benefits of integrating statistical techniques and remote sensing to field survey data in order to perform more reliable in-season corn yield forecasts.
更多查看译文
AI 理解论文
溯源树
样例
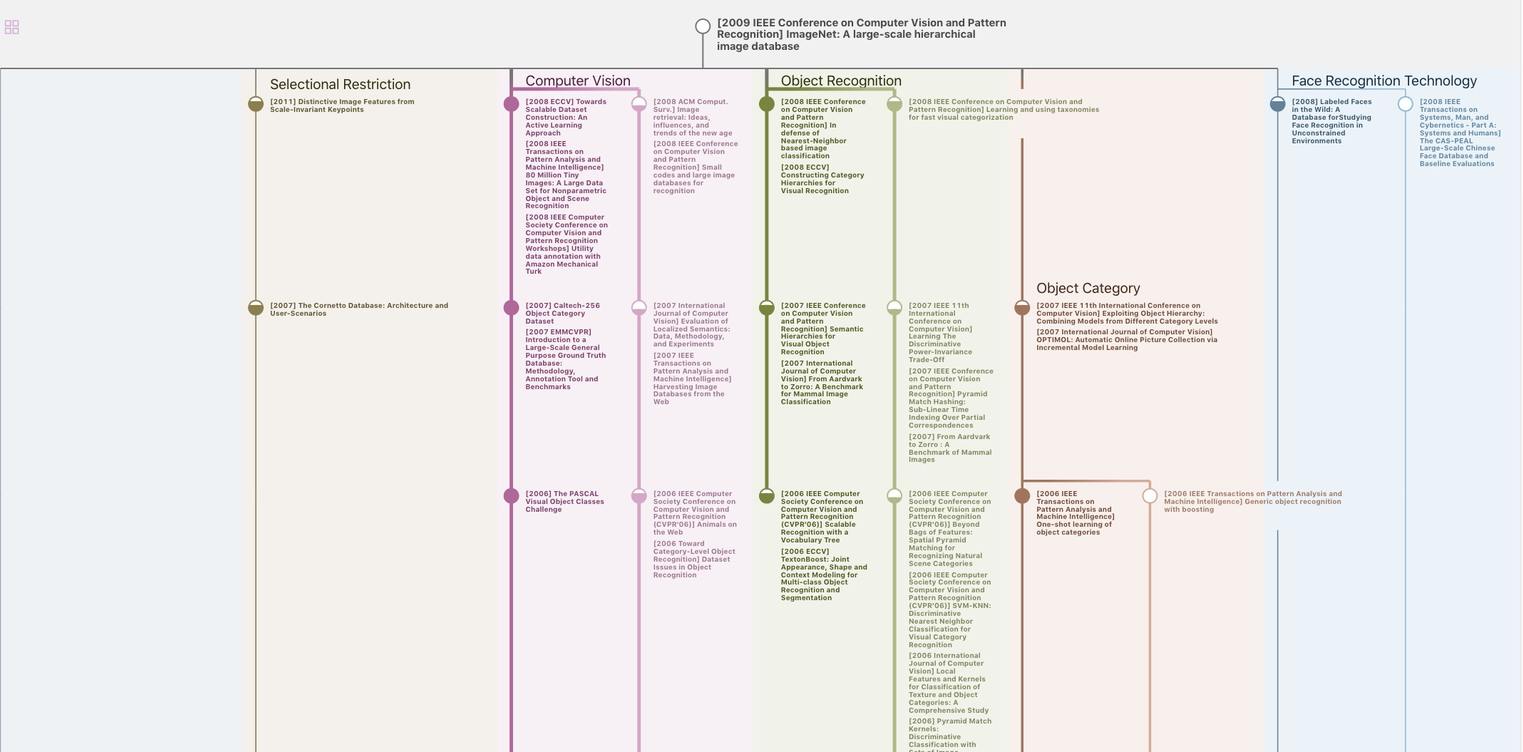
生成溯源树,研究论文发展脉络
Chat Paper
正在生成论文摘要