EVALUATION OF CONVENTIONAL MACHINE LEARNING METHODS FOR BRAIN TUMOUR TYPE CLASSIFICATION
COMPTES RENDUS DE L ACADEMIE BULGARE DES SCIENCES(2020)
摘要
The purpose of this study is to provide an automated method in order to diagnose benign and malignant brain tumours using MR images. Four hundred and sixty-one glioma patients' MR images including low and high grades were downloaded from TCIA portal. After pre-processing, Fuzzy C-Means (FCM) segmentation method was used for tumour region extraction and texture features were extracted from gray level co-occurrence matrix (GLCM). Finally, three classifiers' performances were compared using sensitivity, accuracy and specificity measurements. Random Forest (RF) and K-Nearest Neighbour (KNN) were used without any parameter optimization and Support Vector Machine (SVM) and the parameters were optimized using Grasshopper optimization algorithm (GOA). The applied classifiers performance for different values of its parameters were calculated and the best result was reported as optimum value. For segmentation purpose, using FCM, based on visual inspection, the clusters' optimum number 3 has been measured. In case of RF, SVM with polynomial kernel and KNN, all three measurements were larger than 96% and therefore no optimization was applied. For SVM with Linear and RBF kernel, parameters were optimized using GOA and results demonstrated 39% increase in specificity of linear kernel after optimization. The performance of SVM, RF and KNN methods in classification of brain tumours has been investigated. Reports indicated the specificity and sensitivity for all reviewed methods are larger than 95% and approximate performance equality within results of all methods has been viewed. This high equally performance indicates the robustness of proposed method in glioma type classification.
更多查看译文
关键词
glioma,Fuzzy C-Means,Gray Level Co-occurrence Matrix,Grasshopper Optimization Algorithm,Support Vector Machine
AI 理解论文
溯源树
样例
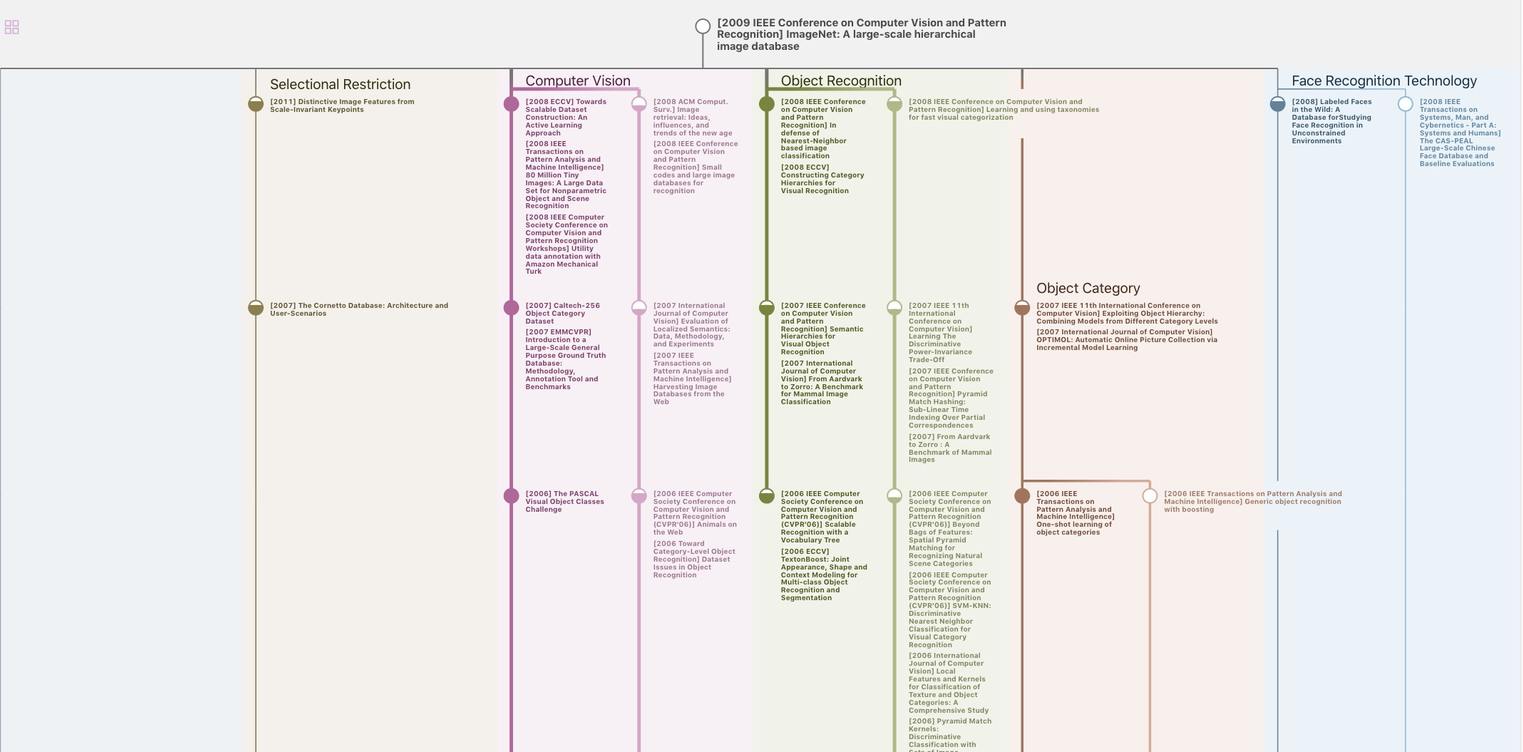
生成溯源树,研究论文发展脉络
Chat Paper
正在生成论文摘要