EPISTEMIC UNCERTAINTY IDENTIFICATION VIA DIFFERENT BAYESIAN INFERENCE METHODS
Engineering Mechanics(2016)
摘要
Currently the Bayesian inference becomes increasingly popular and more widespread approach to parameter identification, which allows to estimate values of input parameters together with appropriate uncertainties by combining a priori information and experimental measurements. In this case, observations are assumed to be performed for the specific yet unknown values of input parameters and epistemic uncertainty arising from experimental errors and lack of knowledge is reduced with an increasing number of experimental observations. Bayes' rule provides an elegant solution to this inverse problem by making it well-posed. However the resulting a posteriori probability distribution usually has a complicated formulation, which cannot be treated analytically. To overcome this obstacle, several methods were developed. The most commonly referred techniques in literature are based on the Markov chain Monte Carlo method, less mentioned approaches utilize the Kalman filter or optimal transport maps. The aim of this contribution is to review and compare these methods of the Bayesian inference.
更多查看译文
关键词
Epistemic uncertainty,Bayesian inference,Markov chain Monte Carlo,Kalman filter,Optimal transport map
AI 理解论文
溯源树
样例
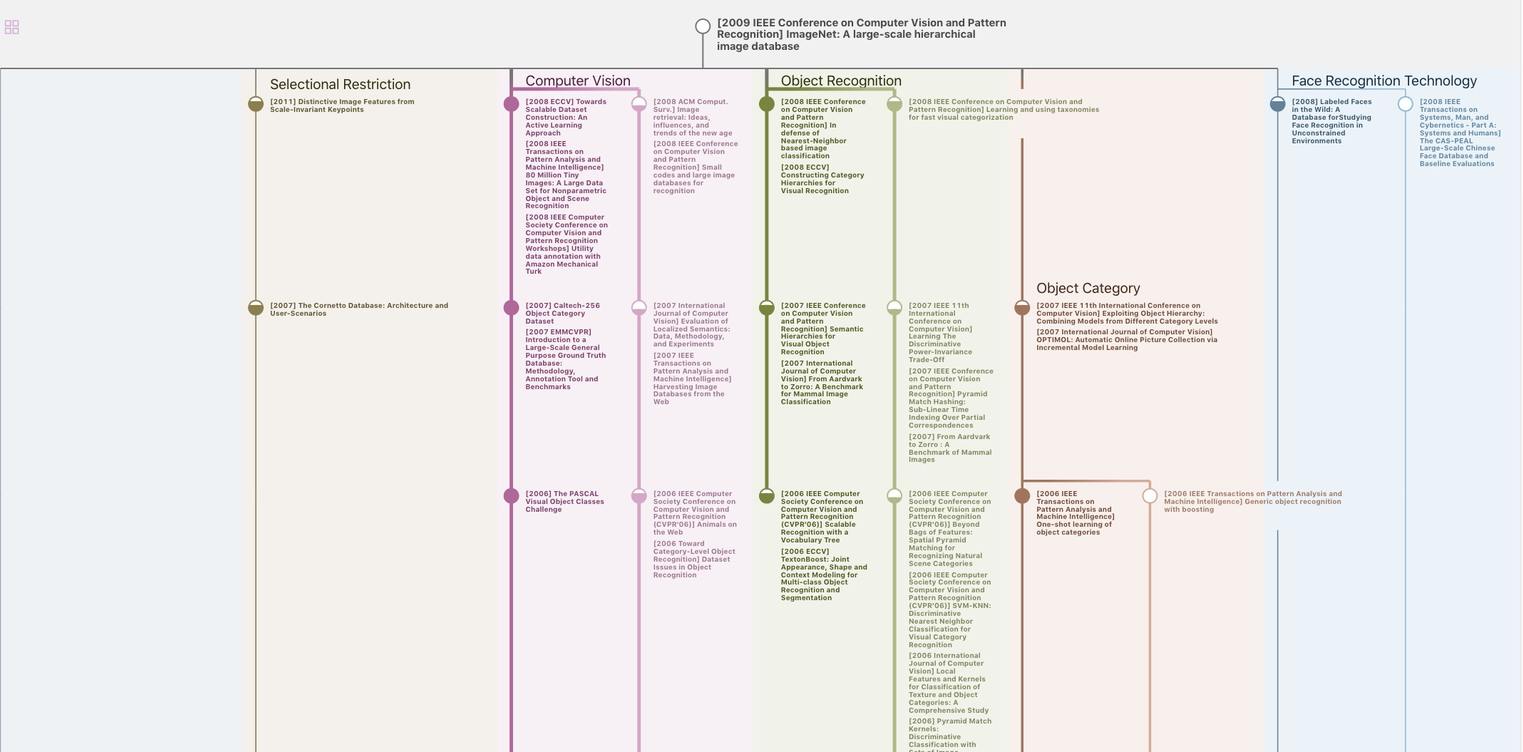
生成溯源树,研究论文发展脉络
Chat Paper
正在生成论文摘要