BAYESIAN PARAMETER IDENTIFICATION OF LATTICE DISCRETE PARTICLE MODEL FOR CONCRETE
Engineering Mechanics(2018)
摘要
The investigated lattice discrete particle model allows to simulate the failure behaviour of concrete. The main characteristic of this model is its response dependence on randomly generated geometrical features of material internal structure according to the basic concrete properties and the size distribution of the aggregates. This makes the model stochastic and the corresponding calibration difficult. On top of this, the model simulation is computationally demanding which limits usage of the full numerical model in an identification procedure. To overcome these obstacles, the polynomial chaos based surrogate model is employed. This approximation eradicates the original model stochasticity, but the corresponding uncertainty can be estimated from the approximation error. The quantified uncertainty is then involved in the Bayesian parameter inference based on Markov chain Monte Carlo sampling with use of cheap surrogate evaluations. The proposed identification procedure is verified on a basis of synthetic experimental data set from notched three-point-bending tests and cube compression tests.
更多查看译文
关键词
lattice discrete particle model,stochastic modelling,polynomial chaos expansion,Bayesian inference,Markov chain Monte Carlo
AI 理解论文
溯源树
样例
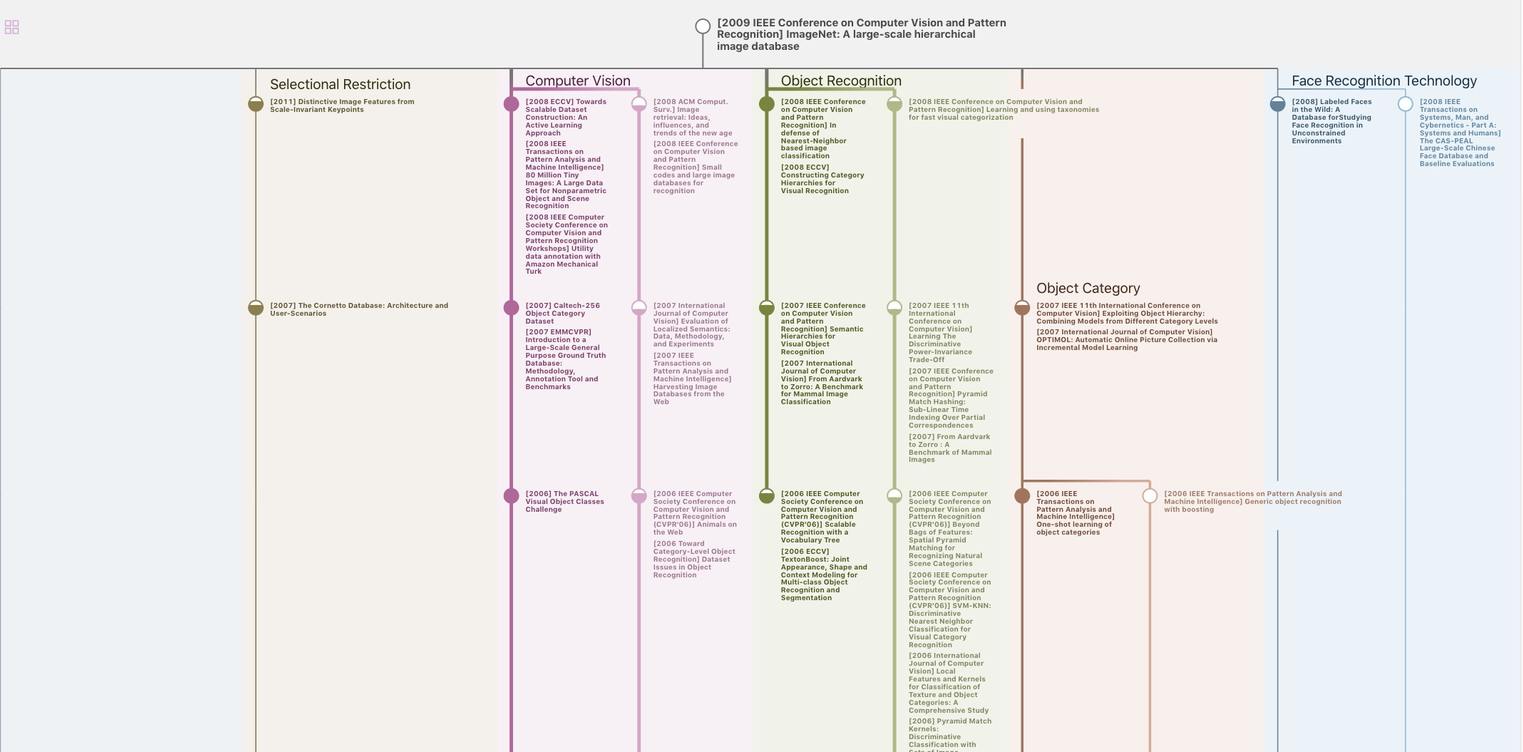
生成溯源树,研究论文发展脉络
Chat Paper
正在生成论文摘要