Direct or indirect on-shore hydrocarbon detection methods applied to hyperspectral data in tropical area
Proceedings of SPIE(2018)
摘要
Detecting onshore hydrocarbon is a major topic for both environmental monitoring and exploration. In this work, a hyperspectral image acquired nearby an old oil extraction site in tropical area is analyzed. The area of interest includes a pit filled with bio-degraded heavy oil, surrounded by herbaceous vegetation and many lagoons. First, we focused on methodologies that can detect oil pollution in an unsupervised manner. Based on the assumption that such oil pits are rare events in the image, statistical approach for anomalies detection, derived from the Reed-Xiaoli detector, is used. In order to decrease the number false alarms, some a priori knowledge about the spectral signature of the pits and about the background is introduced. This approach succeeds in detecting the pit with very few false alarms. Hydrocarbon pollution can have an impact on vegetation and leads to change in vegetation (bio) physical parameters (pigments, water content,...), according to species, pollutant type and exposition time. In order to map the polluted area without any a priori knowledge, several un-supervised classification, including an original method of automatic classification combining unmixing approach and SVM (support Vector Machine) are applied and compared. The results are compared with a partial "ground truth map" that has been derived from visual observations on the field, and with areas of stressed vegetation that have been mapped using combination of specific spectral indices. The classification results are consistent with the ground truth map and the retrieved stressed vegetation areas.
更多查看译文
关键词
Hyperspectral,Hydrocarbon detection,Spectral unmixing,Unsupervised classification,Anomaly detection,spectral index
AI 理解论文
溯源树
样例
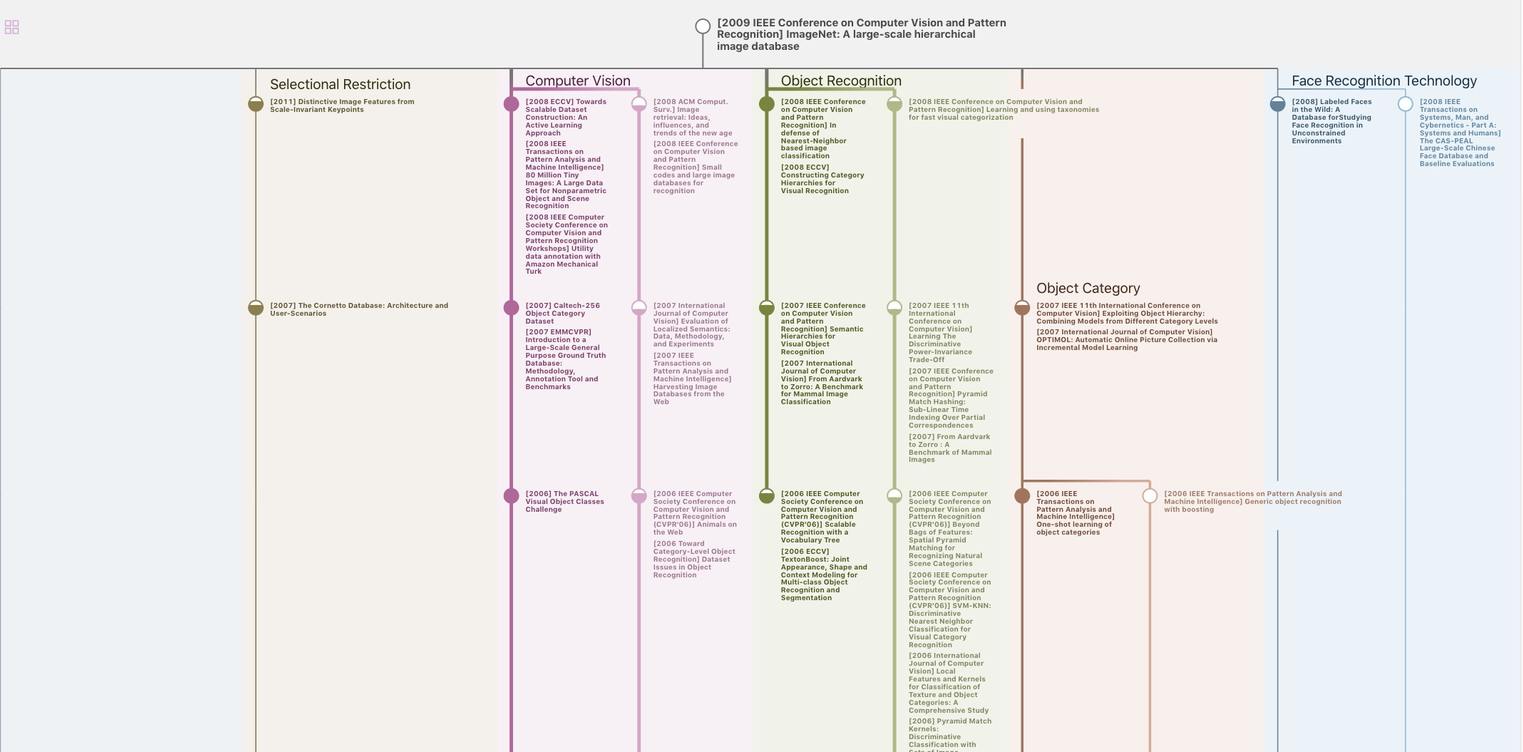
生成溯源树,研究论文发展脉络
Chat Paper
正在生成论文摘要