Deep Learning for PET Image Reconstruction
IEEE Transactions on Radiation and Plasma Medical Sciences(2021)
摘要
This article reviews the use of a subdiscipline of artificial intelligence (AI), deep learning, for the reconstruction of images in positron emission tomography (PET). Deep learning can be used either directly or as a component of conventional reconstruction, in order to reconstruct images from noisy PET data. The review starts with an overview of conventional PET image reconstruction and then covers the principles of general linear and convolution-based mappings from data to images, and proceeds to consider nonlinearities, as used in convolutional neural networks (CNNs). The direct deep-learning methodology is then reviewed in the context of PET reconstruction. Direct methods learn the imaging physics and statistics from scratch, not relying on a priori knowledge of these models of the data. In contrast, model-based or physics-informed deep-learning uses existing advances in PET image reconstruction, replacing conventional components with deep-learning data-driven alternatives, such as for the regularization. These methods use trusted models of the imaging physics and noise distribution, while relying on training data examples to learn deep mappings for regularization and resolution recovery. After reviewing the main examples of these approaches in the literature, the review finishes with a brief look ahead to future directions.
更多查看译文
关键词
Artificial intelligence (AI),deep learning,image reconstruction,machine learning,positron emission tomography (PET)
AI 理解论文
溯源树
样例
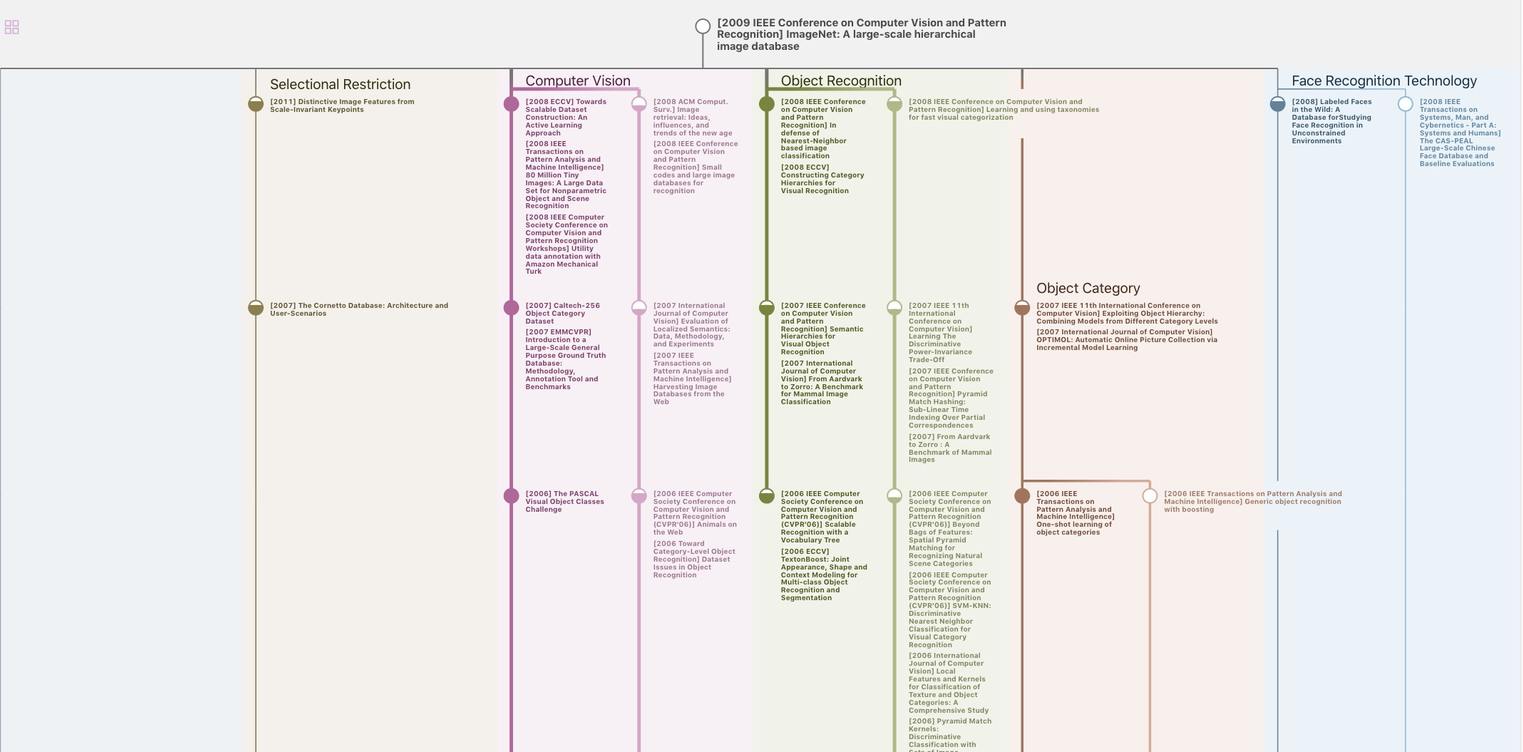
生成溯源树,研究论文发展脉络
Chat Paper
正在生成论文摘要