Comparing Classifiers that Exploit Random Subspaces
Proceedings of SPIE(2019)
Abstract
Many current classification models, such as Random Kitchen Sinks and Extreme Learning Machines (ELM), minimize the need for expert-defined features by transforming the measurement spaces into a set of "features" via random functions or projections. Alternatively, Random Forests exploit random subspaces by limiting tree partitions (i.e. nodes of the tree) to be selected from randomly generated subsets of features. For a synthetic aperture RADAR classification task, and given two orthonormal measurement representations (spatial and multi-scale Haar wavelet), this work compares and contrasts ELM and Random Forest classifier performance as a function of (a) input measurement representation, (b) classifier complexity, and (c) measurement domain mismatch. For the ELM classifier, we also compare two random projection encodings.
MoreTranslated text
Key words
Extreme Learning Machine,Random Forests,Random Subspaces,Random Projections
AI Read Science
Must-Reading Tree
Example
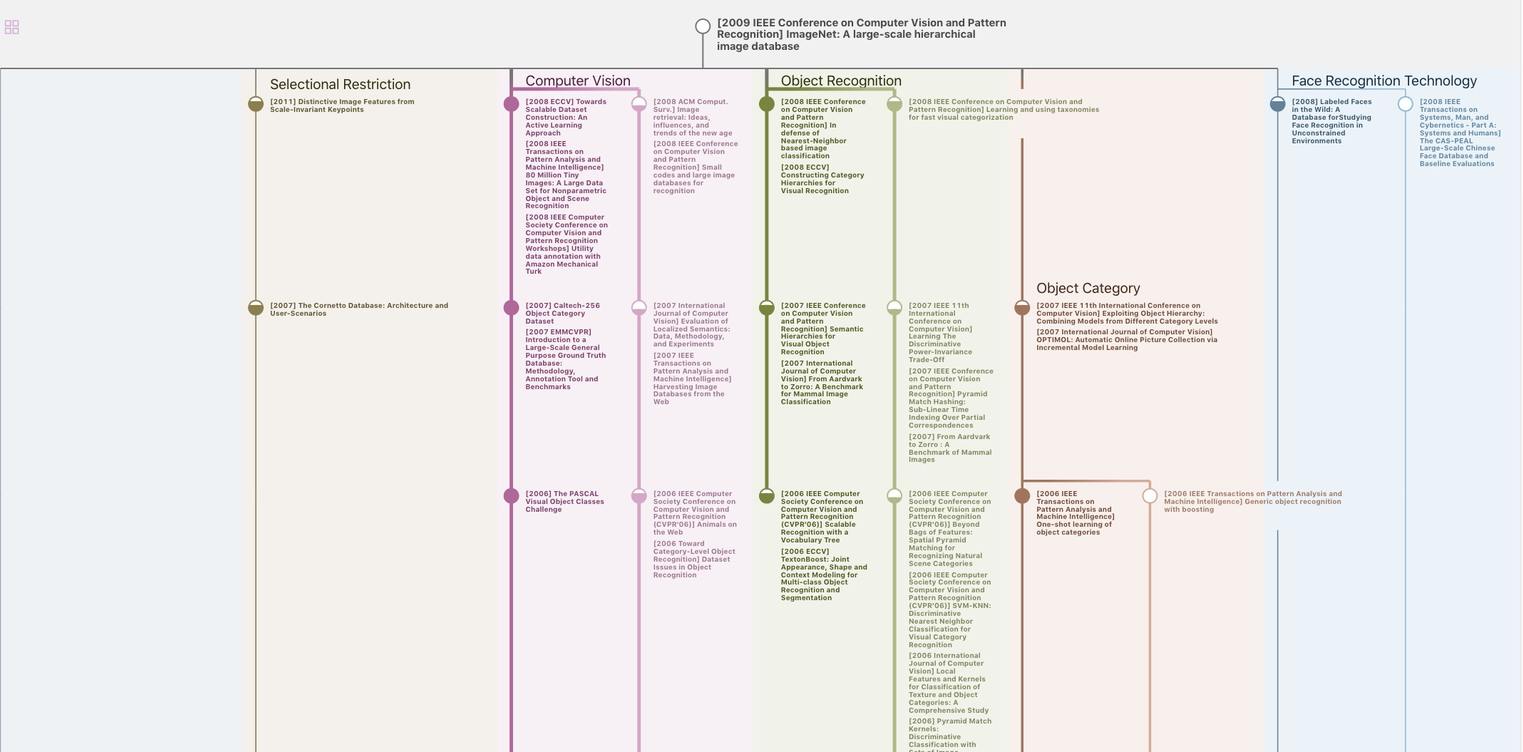
Generate MRT to find the research sequence of this paper
Chat Paper
Summary is being generated by the instructions you defined