Towards automated human gait disease classification using phase space representation of intrinsic mode functions
Proceedings of SPIE(2017)
摘要
A novel analytical methodology for segregating healthy and neurological disorders from gait patterns is proposed by employing a set of oscillating components called intrinsic mode functions (IMF's). These IMF's are generated by the Empirical Mode Decomposition of the gait time series and the Hilbert transformed analytic signal representation forms the complex plane trace of the elliptical shaped analytic IMFs. The area measure and the relative change in the centroid position of the polygon formed by the Convex Hull of these analytic IMF's are taken as the discriminative features. Classification accuracy of 79.31% with Ensemble learning based Adaboost classifier validates the adequacy of the proposed methodology for a computer aided diagnostic (CAD) system for gait pattern identification. Also, the efficacy of several potential biomarkers like Bandwidth of Amplitude Modulation & Frequency Modulation IMF's and it's Mean Frequency from the Fourier-Bessel expansion from each of these analytic IMF's has been discussed for its potency in diagnosis of gait pattern identification & classification.
更多查看译文
关键词
Intrinsic mode functions,Empirical mode decomposition,Hilbert Transform,Convex Hull,SVM
AI 理解论文
溯源树
样例
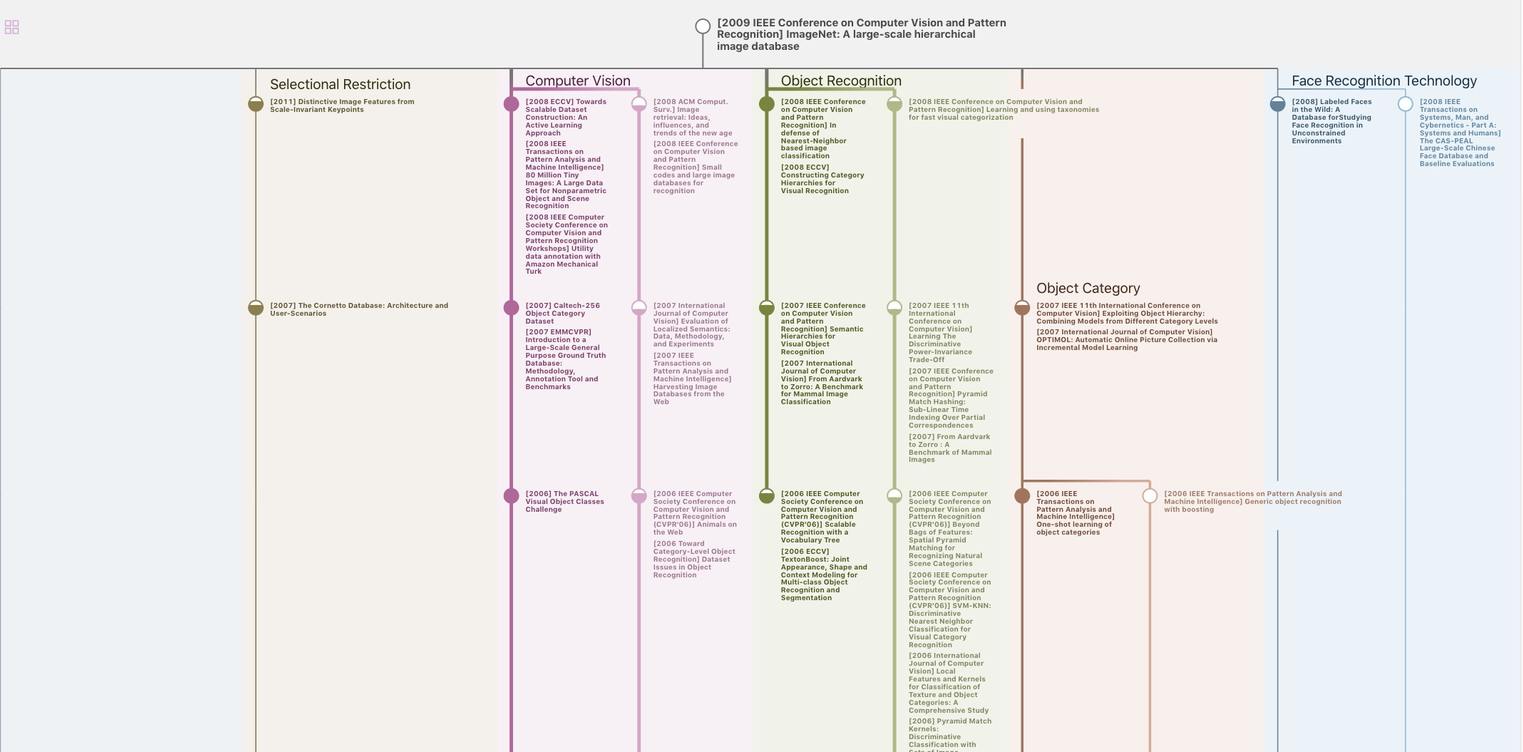
生成溯源树,研究论文发展脉络
Chat Paper
正在生成论文摘要