Scalable nested partition-based clustering with two-stage sampling
ASIA LIFE SCIENCES(2015)
摘要
As the size of databases has grown tremendously in modern times, it becomes very important to be able to extract meaningful information from enormous datasets, within a reasonable computation time. Accordingly, the data mining community has made efforts to enhance the scalability of most data mining algorithms, such as with clustering. Since the induction time of clustering algorithms is generally affected by the number of attributes and instances, a subset of instances is often used to improve the scalability. This paper suggests a scalable clustering algorithm with a two-stage statistical selection that extends the NP-based (Nested Partition-based) clustering method so as to make it into a tool to improve scalability. The two-stage NP-based clustering algorithm that we propose in this paper, (TS_NPCLUSTER), augmented by properly adjusting the sample size of solutions in each partitioning region of the NP framework, has the capability to resolve noisy performance problems that arise when using a subset of instances. The two-stage sampling of TS_NPCLUSTER can effectively resolve such problems as unwanted backtrackings caused by the noisy performance problem that are the critical cause of long induction times. The numerical results show that the TS_NPCLUSTER has better similarity of clusters and more acceptable computation time with various test datasets than the other benchmark clustering schemes.
更多查看译文
关键词
clustering,two-stage sampling,nested partition,data mining,metaheuristics
AI 理解论文
溯源树
样例
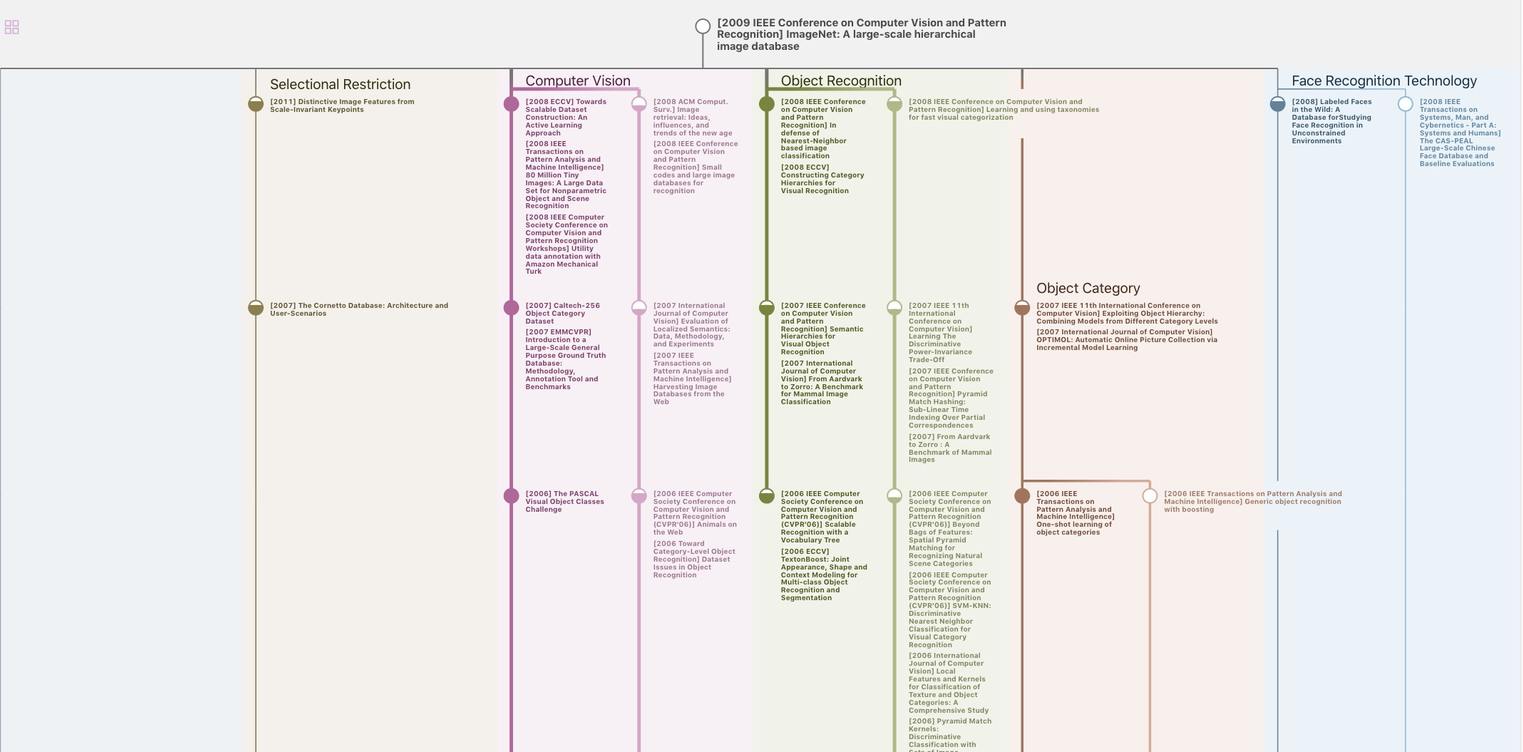
生成溯源树,研究论文发展脉络
Chat Paper
正在生成论文摘要