A NOVEL REMOTE SENSING RECOGNITION METHOD BASED ON DBN ENSEMBLE AND D-S EVIDENCE SYNTHESIS
JOURNAL OF NONLINEAR AND CONVEX ANALYSIS(2020)
Abstract
Deep belief network (DBN) is a generative learning strategy which has been proposed very early, similar to the rest deep learning algorithms, the complex network structure and the large size of parameters increase calculation cost greatly, the application is limited greatly. In order to solve this problem, a decision-level identification method named distributed DBN weighted Dempster-Shafer synthesis (DDWDS) is proposed for high-precision classification of remote sensing objects. Firstly, the low-dimensional features and a small number of samples are selected randomly, many distributed, independent and lean DBN networks are constructed and trained. Secondly, their classification results are used as a trust allocation function of the evidence focal element, and finally obtain the trust allocation function of each focal element based on weighted synthetic rules. With the multi-source remote sensing data of the light detection and ranging(LIDAR) system, the recognition accuracy of distributed DBNs which are constructed by 4 features and 20% samples is below 0.7, this situation shows great conflict, training cost decreases significantly, but the final classification recognition accuracy is raised to more than 0.9 with the proposed DD-WDS. The results show that the method can determine the scale of the network by itself, and achieves better performance.
MoreTranslated text
Key words
Deep believe network,conflict evidence-weighted integration,LIDAR land-cover classification,decision-level identification
AI Read Science
Must-Reading Tree
Example
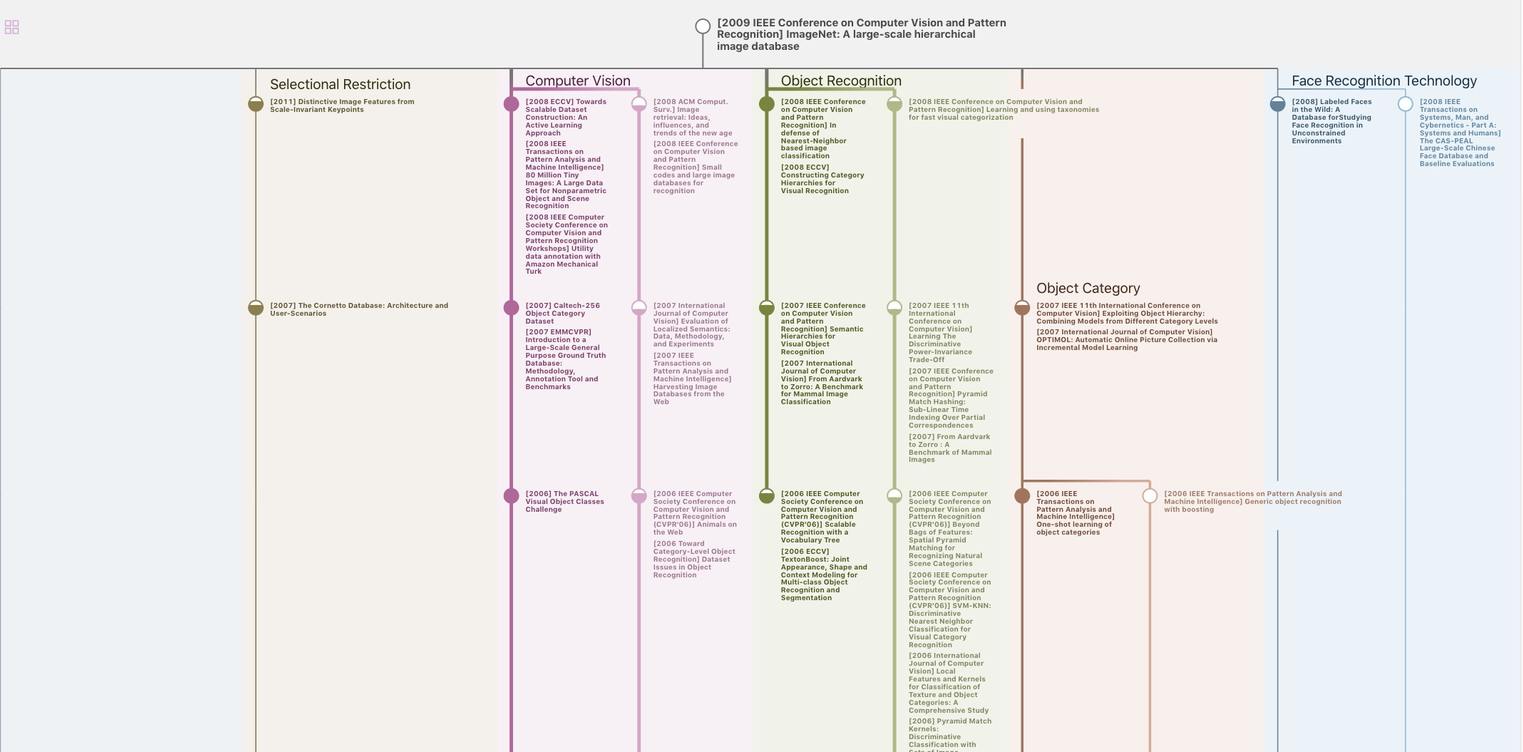
Generate MRT to find the research sequence of this paper
Chat Paper
Summary is being generated by the instructions you defined