Method for Generating Malicious Code Adversarial Samples Based on Genetic Algorithm
JOURNAL OF ELECTRONICS & INFORMATION TECHNOLOGY(2020)
摘要
Machine learning is widely used in malicious code detection and plays an important role in malicious code detection products. Constructing adversarial samples for malicious code detection machine learning models is the key to discovering defects in malicious code detection models, evaluating and improving malicious code detection systems. This paper proposes a method for generating malicious code adversarial samples based on genetic algorithms. The generated samples combat effectively the malicious code detection model based on machine learning, while ensuring the consistency of the executable and malicious behavior of malicious code samples, and improving effectively the authenticity of the generated adversarial samples and the accuracy of the model adversarial evaluation are presented. The experiments show that the proposed method of generating adversarial samples reduces the detection accuracy of the MalConv malicious code detection model by 14.65%, and can directly interfere with four commercial machine-based malicious code detection engines in VirusTotal. Among them, the accuracy rate of Cylance detection is only 53.55%.
更多查看译文
关键词
Malware detection,Machine learning,Adversarial sample
AI 理解论文
溯源树
样例
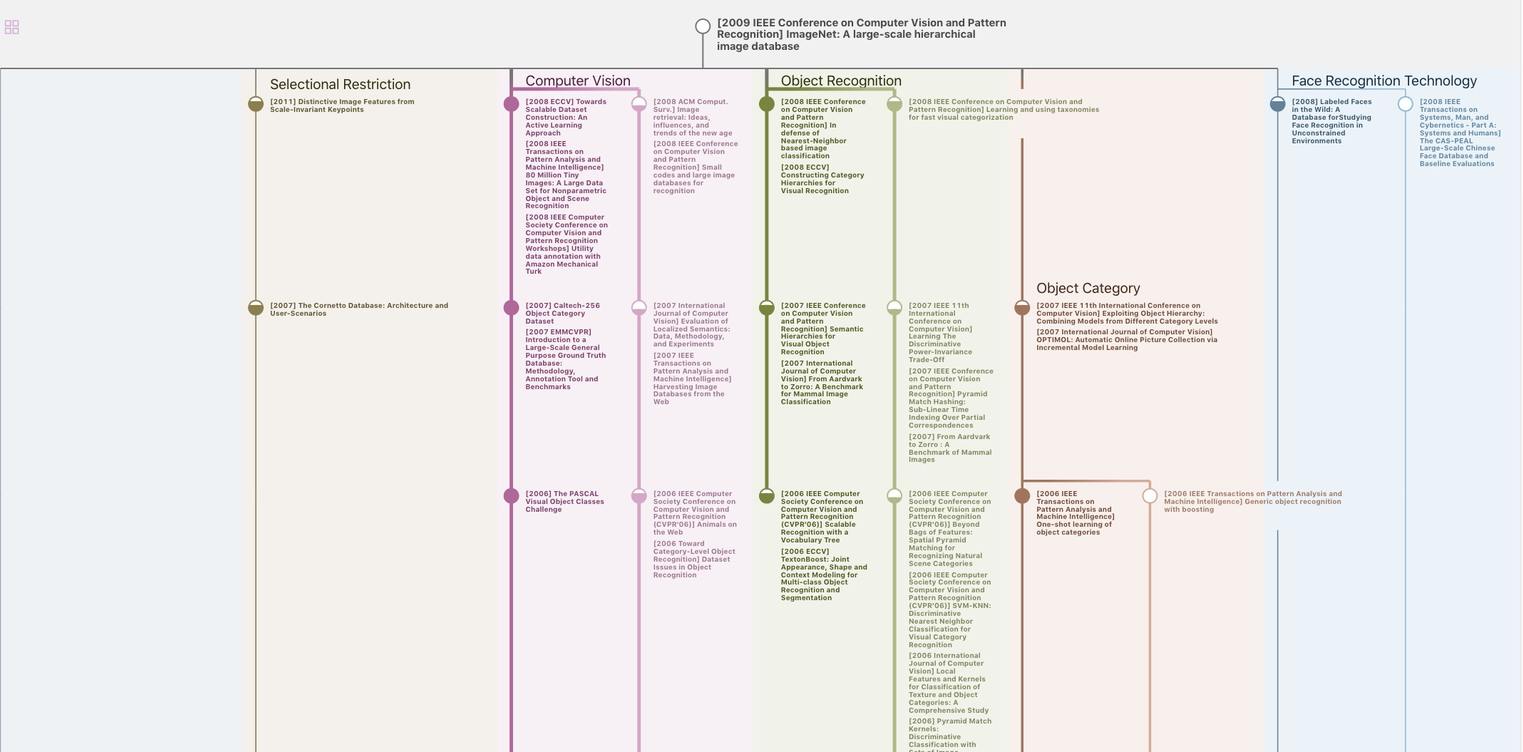
生成溯源树,研究论文发展脉络
Chat Paper
正在生成论文摘要