Machine learning approaches for defect classification on aircraft fuselage images acquired by an UAV
Proceedings of SPIE(2019)
摘要
In order to ease visual inspections of exterior aircraft fuselage, new technical approaches have been recently deployed. Automated UAVs are now acquiring high quality images of the aircraft in order to perform offline analysis. At first, some acquisitions are annotated by human operators in order to provide a large dataset required to train machine learning methods, especially for critical defects detection. An intrinsic problem of this dataset is its extreme imbalance (i.e there is an unequal distribution between classes): the rarest and most valuable samples represent few elements among thousands of annotated objects. Deep Learning-only based approaches have proven to be very effective when a sufficient amount of data is available for each desired class, whereas less complex systems such as Support Vector Machine theoretically need less data, and few-shot learning dedicated methods (Matching Network, Prototypical Network, etc.) can learn from only few examples. Those approaches are compared on our applicative case. Preliminary results show the existence of empirical frontiers in term of training dataset volume that indicate which approach might be favored. Based on those results, we propose a method to combine different approaches in order to achieve best performances on categorical accuracy, with special attention paid to underrepresented category.
更多查看译文
关键词
Deep Learning,Few-shot Learning,Hybrid model,Defect Detection,Support Vector Machine,Visual Inspection
AI 理解论文
溯源树
样例
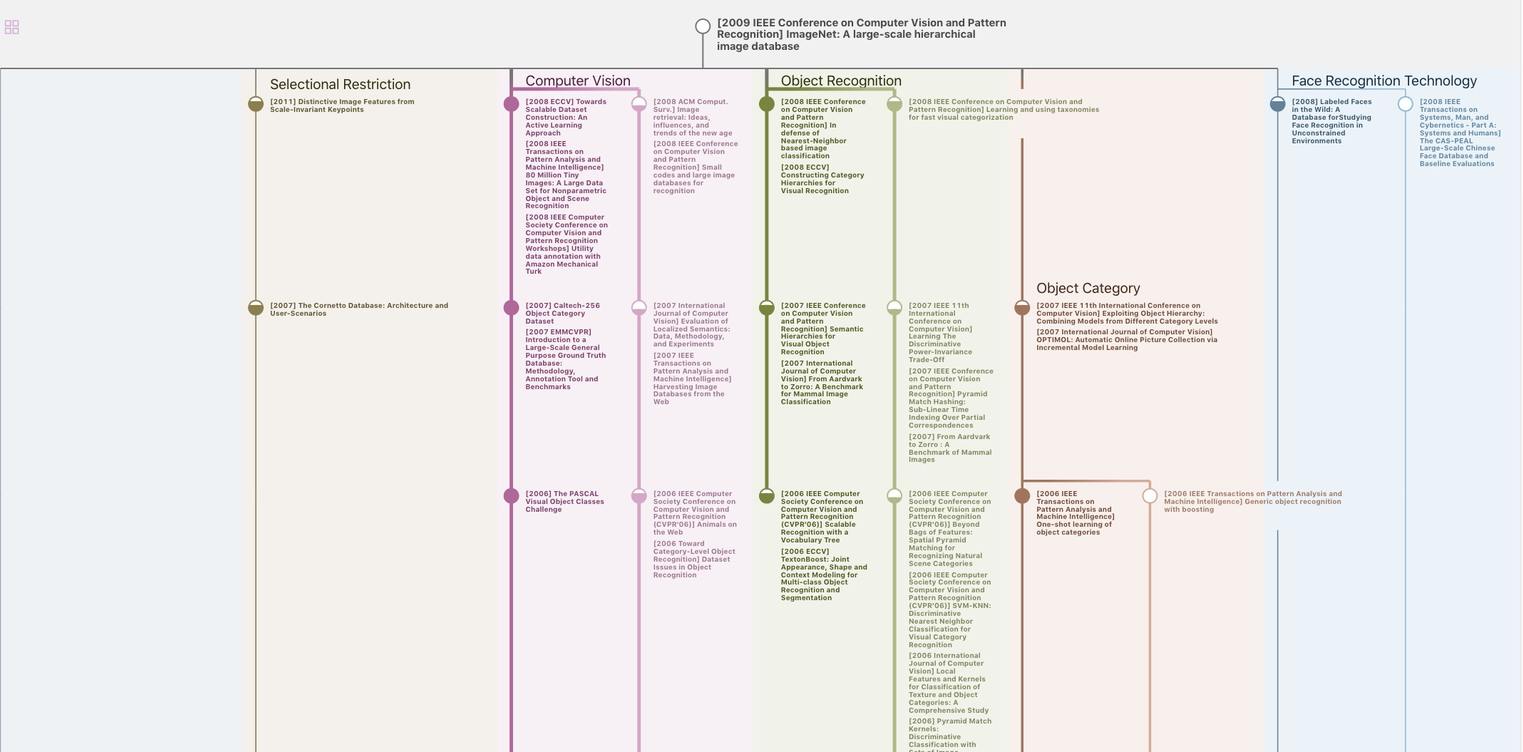
生成溯源树,研究论文发展脉络
Chat Paper
正在生成论文摘要