QRS complex detection based on continuous density hidden Markov models using univariate observations
Journal of Physics Conference Series(2018)
Abstract
In the electrocardiogram (ECG), the detection of QRS complexes is a fundamental step in the ECG signal processing chain since it allows the determination of other characteristics waves of the ECG and provides information about heart rate variability. In this work, an automatic QRS complex detector based on continuous density hidden Markov models (HMM) is proposed. HMM were trained using univariate observation sequences taken either from QRS complexes or their derivatives. The detection approach is based on the log-likelihood comparison of the observation sequence with a fixed threshold. A sliding window was used to obtain the observation sequence to be evaluated by the model. The threshold was optimized by receiver operating characteristic curves. Sensitivity (Sen), specificity (Spc) and F1 score were used to evaluate the detection performance. The approach was validated using ECG recordings from the MIT-BIH Arrhythmia database. A 6-fold cross-validation shows that the best detection performance was achieved with 2 states HMM trained with QRS complexes sequences (Sen = 0.668, Spc = 0.360 and F1 = 0.309). We concluded that these univariate sequences provide enough information to characterize the QRS complex dynamics from HMM. Future works are directed to the use of multivariate observations to increase the detection performance.
MoreTranslated text
Key words
complex detection,qrs,markov models,univariate observations
AI Read Science
Must-Reading Tree
Example
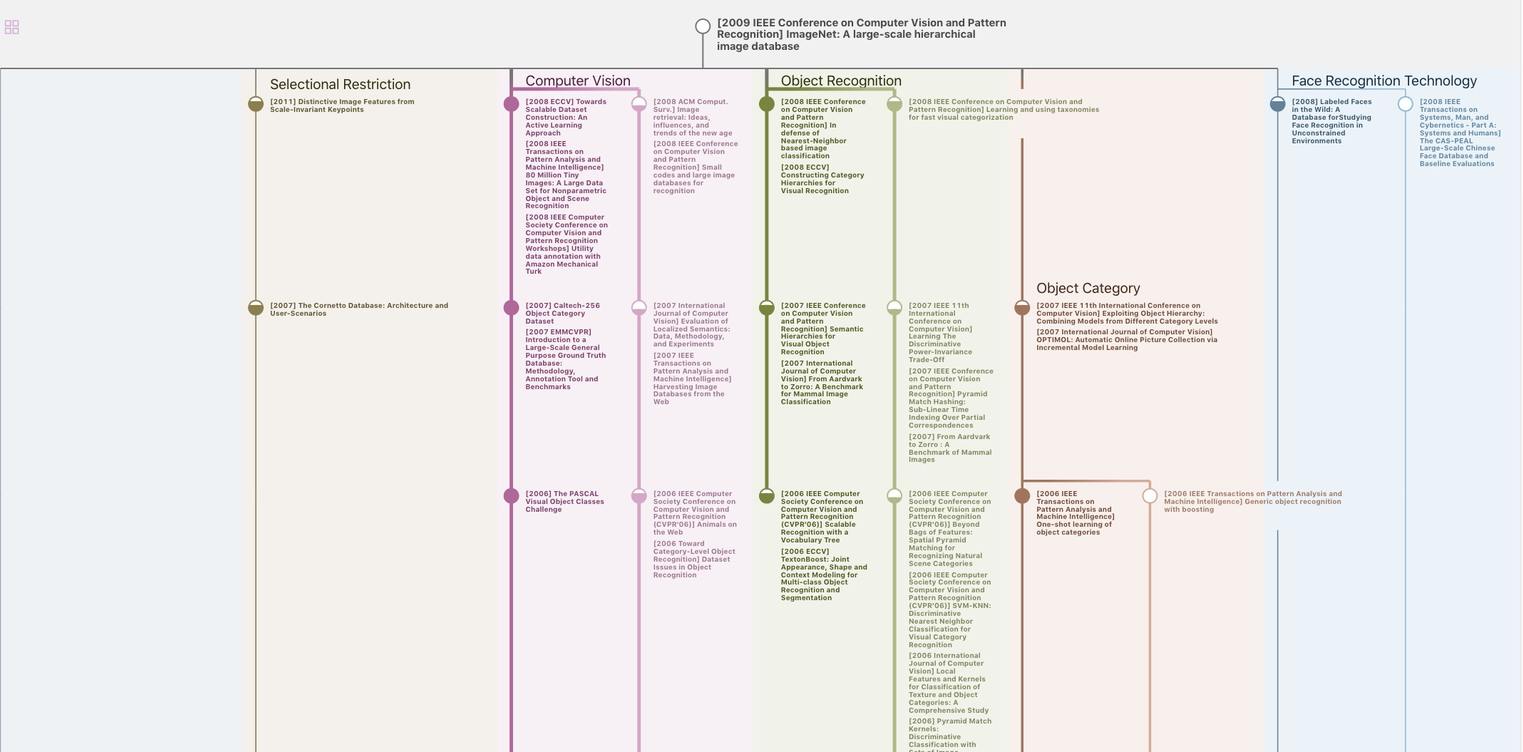
Generate MRT to find the research sequence of this paper
Chat Paper
Summary is being generated by the instructions you defined