Towards Constraining Warm Dark Matter With Stellar Streams Through Neural Simulation-Based Inference
MONTHLY NOTICES OF THE ROYAL ASTRONOMICAL SOCIETY(2021)
摘要
A statistical analysis of the observed perturbations in the density of stellar streams can in principle set stringent constraints on the mass function of dark matter subhaloes, which in turn can be used to constrain the mass of the dark matter particle. However, the likelihood of a stellar density with respect to the stream and subhaloes parameters involves solving an intractable inverse problem which rests on the integration of all possible forward realizations implicitly defined by the simulation model. In order to infer the subhalo abundance, previous analyses have relied on Approximate Bayesian Computation (ABC) together with domain-motivated but handcrafted summary statistics. Here, we introduce a likelihood-free Bayesian inference pipeline based on Amortised Approximate Likelihood Ratios (AALR), which automatically learns a mapping between the data and the simulator parameters and obviates the need to handcraft a possibly insufficient summary statistic. We apply the method to the simplified case where stellar streams are only perturbed by dark matter subhaloes, thus neglecting baryonic substructures, and describe several diagnostics that demonstrate the effectiveness of the new method and the statistical quality of the learned estimator.
更多查看译文
关键词
methods: statistical, galaxies: structure, dark matter
AI 理解论文
溯源树
样例
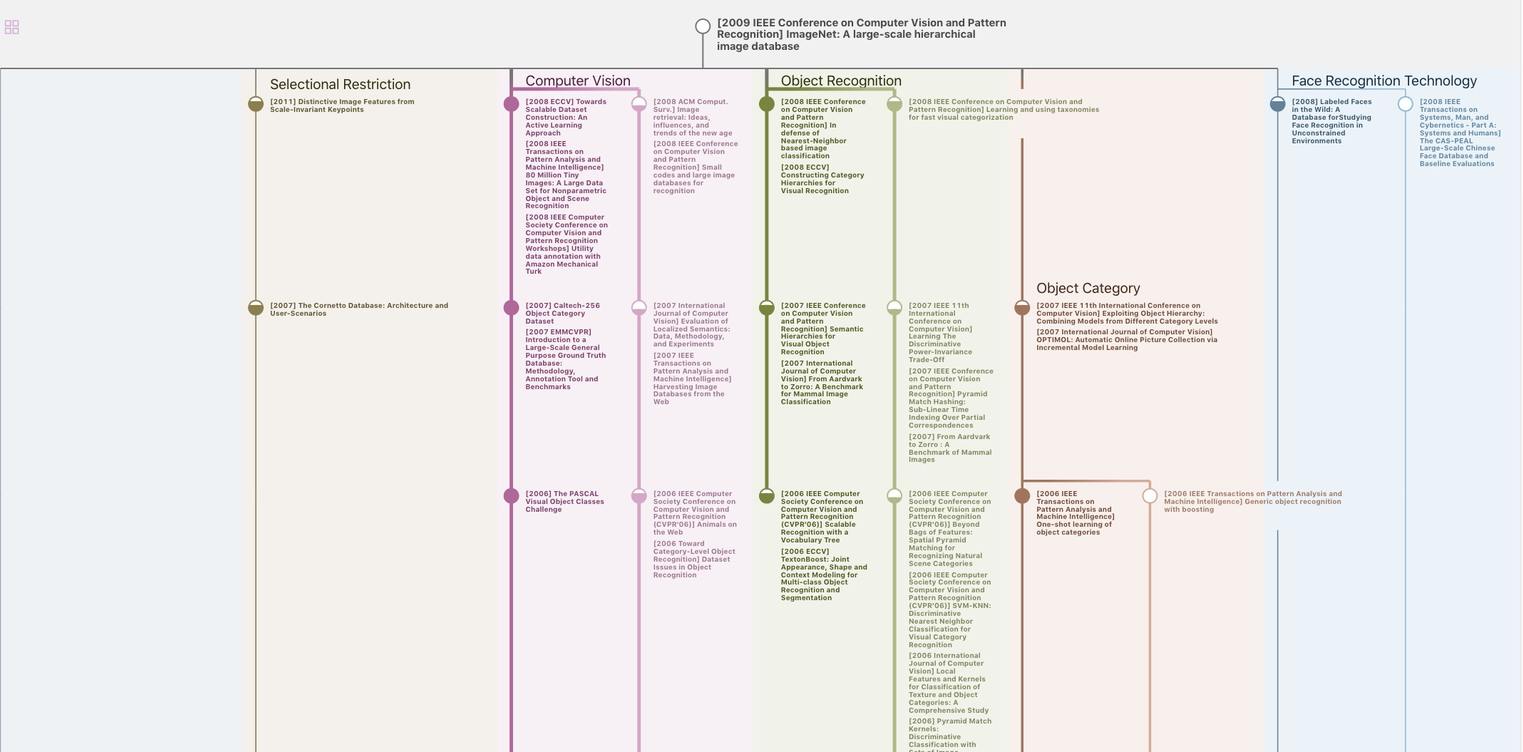
生成溯源树,研究论文发展脉络
Chat Paper
正在生成论文摘要