CanonPose: Self-Supervised Monocular 3D Human Pose Estimation in the Wild
2021 IEEE/CVF CONFERENCE ON COMPUTER VISION AND PATTERN RECOGNITION, CVPR 2021(2021)
Abstract
Human pose estimation from single images is a challenging problem in computer vision that requires large amounts of labeled training data to be solved accurately. Unfortunately, for many human activities (e.g. outdoor sports) such training data does not exist and is hard or even impossible to acquire with traditional motion capture systems. We propose a self-supervised approach that learns a single image 3D pose estimator from unlabeled multi-view data. To this end, we exploit multi-view consistency constraints to disentangle the observed 2D pose into the underlying 3D pose and camera rotation. In contrast to most existing methods, we do not require calibrated cameras and can therefore learn from moving cameras. Nevertheless, in the case of a static camera setup, we present an optional extension to include constant relative camera rotations over multiple views into our framework. Key to the success are new, unbiased reconstruction objectives that mix information across views and training samples. The proposed approach is evaluated on two benchmark datasets (Human3.6M and MPII-INF-3DHP) and on the in-the-wild SkiPose dataset.
MoreTranslated text
AI Read Science
Must-Reading Tree
Example
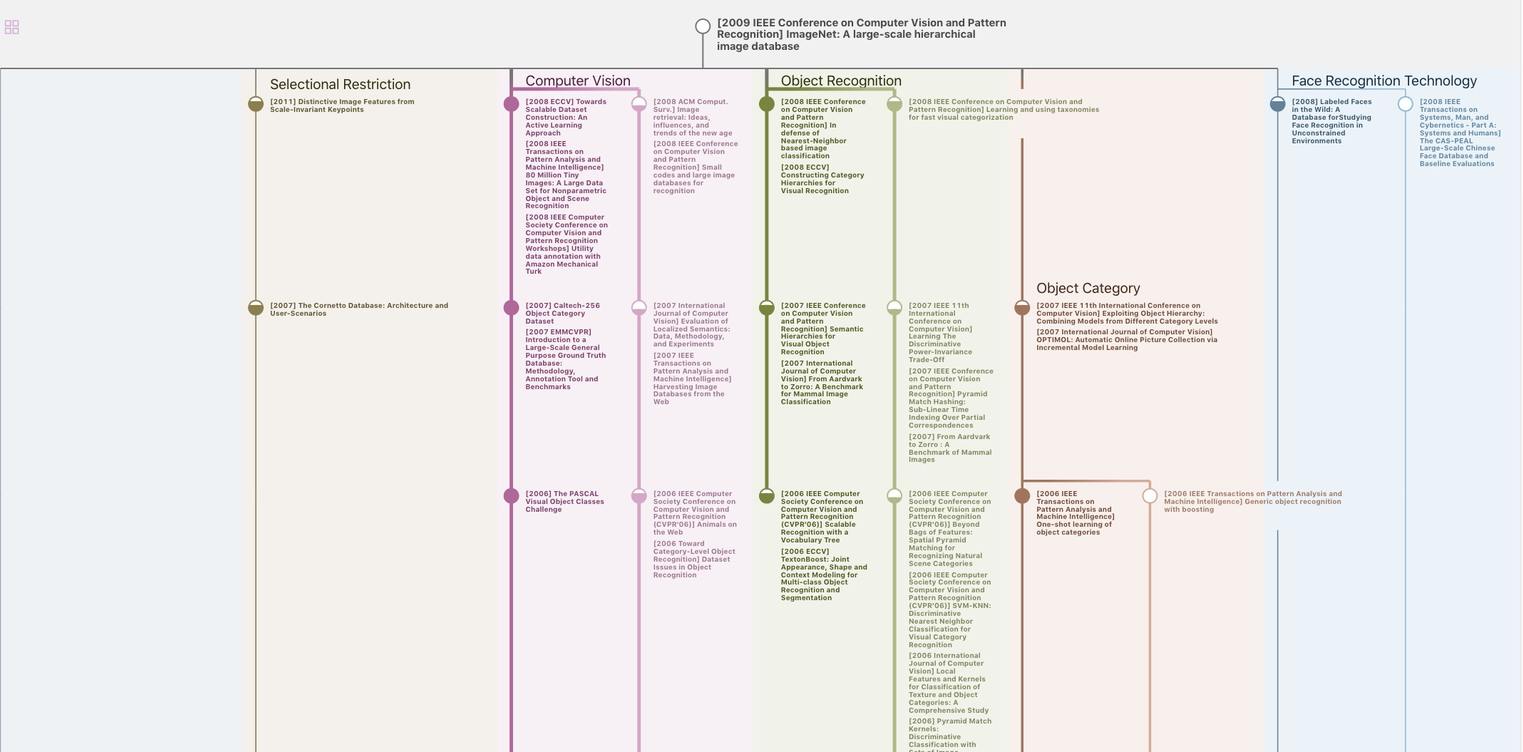
Generate MRT to find the research sequence of this paper
Chat Paper
Summary is being generated by the instructions you defined