Calibration for multivariate Lévy-driven Ornstein-Uhlenbeck processes with applications to weak subordination
Statistical Inference for Stochastic Processes(2021)
摘要
Consider a multivariate Lévy-driven Ornstein-Uhlenbeck process where the stationary distribution or background driving Lévy process is from a parametric family. We derive the likelihood function assuming that the innovation term is absolutely continuous. Two examples are studied in detail: the process where the stationary distribution or background driving Lévy process is given by a weak variance alpha-gamma process, which is a multivariate generalisation of the variance gamma process created using weak subordination. In the former case, we give an explicit representation of the background driving Lévy process, leading to an innovation term which is a discrete and continuous mixture, allowing for the exact simulation of the process, and a separate likelihood function. In the latter case, we show the innovation term is absolutely continuous. The results of a simulation study demonstrate that maximum likelihood numerically computed using Fourier inversion can be applied to accurately estimate the parameters in both cases.
更多查看译文
关键词
Lévy process, Ornstein-Uhlenbeck process, Self-decomposability, Likelihood inference, Multivariate subordination, Weak subordination, Variance gamma process, Primary: 62M05, 60G51, 60G10, Secondary: 62F10, 60E10, 60H05
AI 理解论文
溯源树
样例
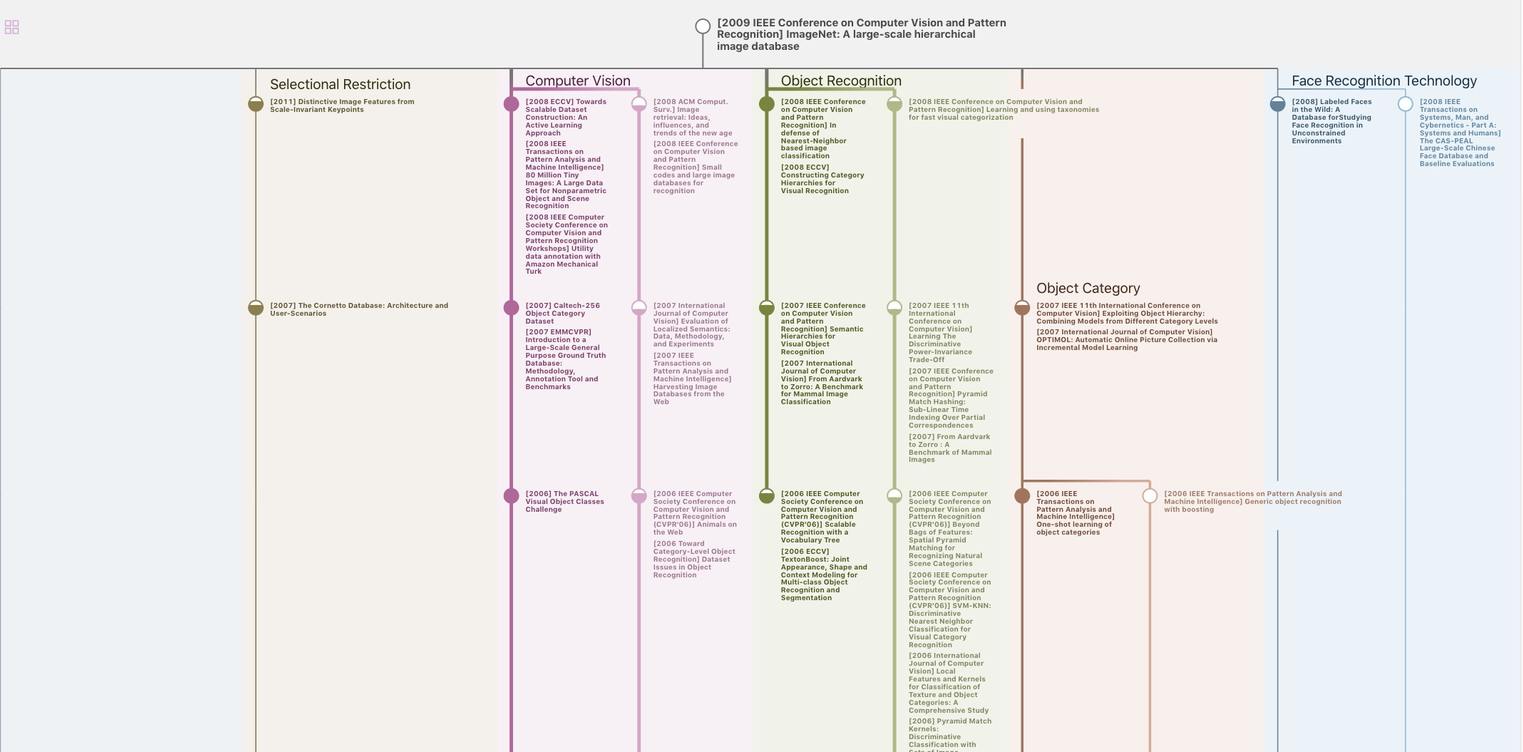
生成溯源树,研究论文发展脉络
Chat Paper
正在生成论文摘要