Synthetic Lethality-Based Predictive Biomarker Identification of Splicing Modulators in Lung Cancer
CANCER RESEARCH(2019)
Key words
Gene Expression Regulation
AI Read Science
Must-Reading Tree
Example
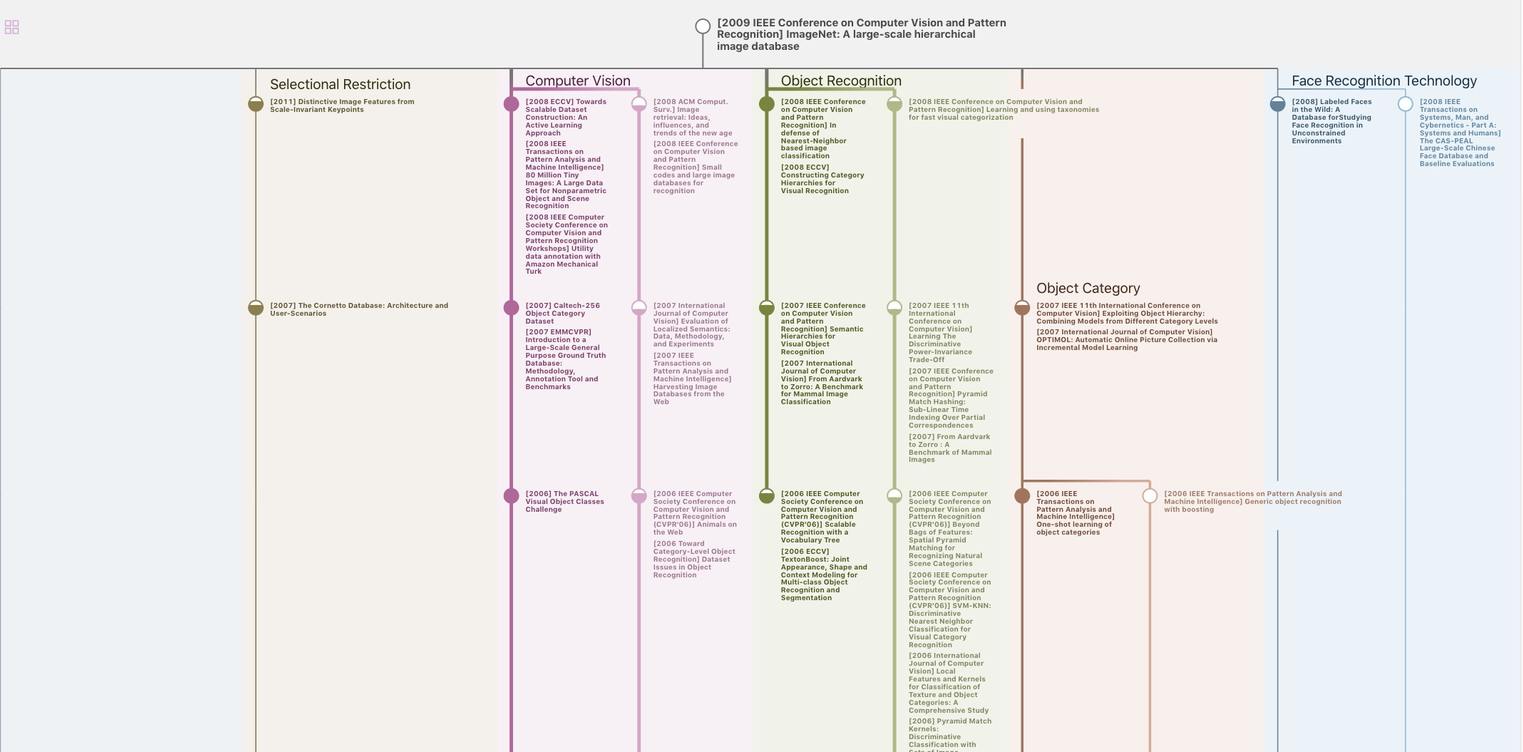
Generate MRT to find the research sequence of this paper
Chat Paper
Summary is being generated by the instructions you defined