Accelerating MCMC algorithms through Bayesian Deep Networks
CoRR(2020)
摘要
Markov Chain Monte Carlo (MCMC) algorithms are commonly used for their versatility in sampling from complicated probability distributions. However, as the dimension of the distribution gets larger, the computational costs for a satisfactory exploration of the sampling space become challenging. Adaptive MCMC methods employing a choice of proposal distribution can address this issue speeding up the convergence. In this paper we show an alternative way of performing adaptive MCMC, by using the outcome of Bayesian Neural Networks as the initial proposal for the Markov Chain. This combined approach increases the acceptance rate in the Metropolis-Hasting algorithm and accelerate the convergence of the MCMC while reaching the same final accuracy. Finally, we demonstrate the main advantages of this approach by constraining the cosmological parameters directly from Cosmic Microwave Background maps.
更多查看译文
关键词
mcmc algorithms,bayesian deep networks
AI 理解论文
溯源树
样例
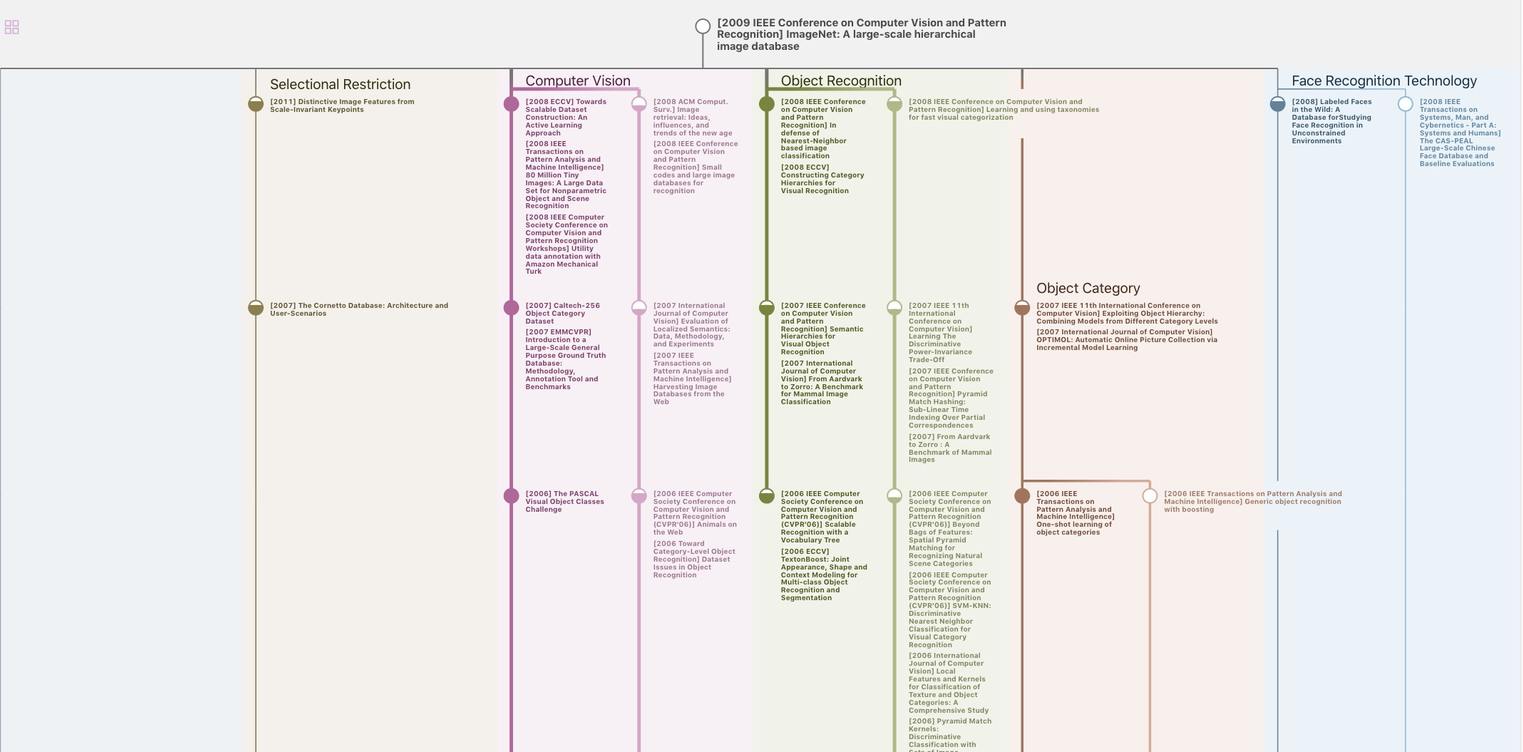
生成溯源树,研究论文发展脉络
Chat Paper
正在生成论文摘要