Wrapper Full-scale Sample Selection Algorithm for Thermal Process Modeling
AIP Conference Proceedings(2018)
摘要
The thermal process modeling based on the operating data is effective to overcome the deficiency of mechanism modeling. In order to reduce the computational complexity of data-driven modeling, many sample selection algorithms have been used to decreasing the training dataset scale. However, traditional sampling selection algorithm may fail to cover full-scale working condition of thermal process when the dataset has large density deviations, and the sample selection criteria is often irrelevant to the modeling prediction. To overcome these issues, this paper proposes a wrapper full-scale sample selection algorithm for thermal process modeling, in which, samples will be sequentially added until there is no improvement in prediction, and sample selection criteria is the prediction evaluated by KNN regression model beforehand. It reveals that our algorithm not only cover full-scale working condition of thermal process, but also has certain superiority in model accuracy. Numerical simulation and real thermal application are conducted to validate the performance of the proposed algorithm.
更多查看译文
关键词
thermal process modeling,sampling selection,density biased sampling,KNN regression
AI 理解论文
溯源树
样例
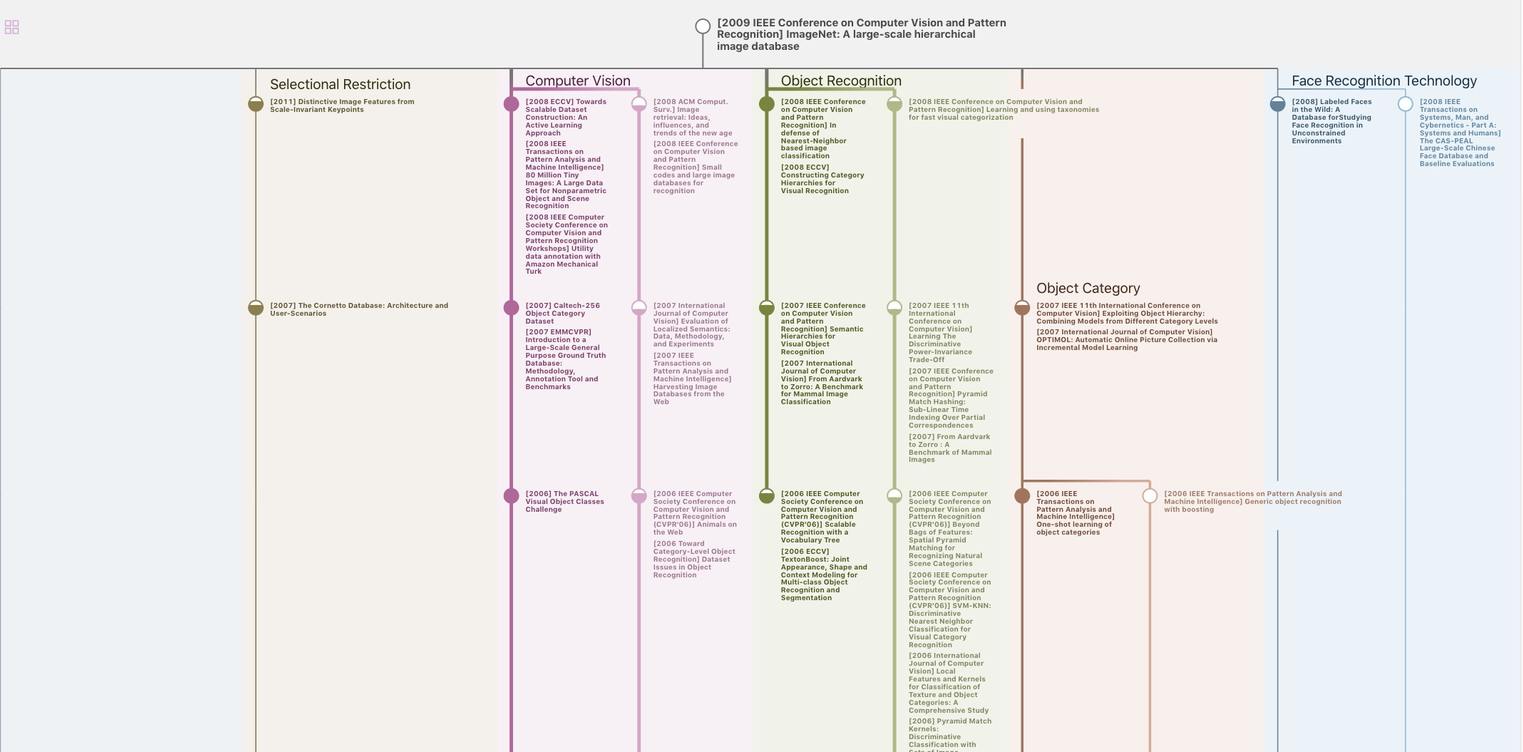
生成溯源树,研究论文发展脉络
Chat Paper
正在生成论文摘要