Benchmarking Inference Performance of Deep Learning Models on Analog Devices
2021 International Joint Conference on Neural Networks (IJCNN)(2021)
摘要
Analog hardware implemented deep learning models are promising for computation and energy constrained systems such as edge computing devices. However, the analog nature of the device and the many associated noise sources will cause changes to the value of the weights in the trained deep learning models deployed on such devices. In this study, systematic evaluation of the inference performance of trained popular deep learning models for image classification deployed on analog devices has been carried out, where additive white Gaussian noise has been added to the weights of the trained models during inference. It is observed that deeper models and models with more redundancy in design, such as VGG, are more robust to the noise in general. Also, it is observed that the performance is affected by the design philosophy of the model, the detailed structure of the model, the exact machine learning task, as well as the datasets.
更多查看译文
关键词
Deep Learning,Hardware Implemented Neural Network,Analog Device,Additive White Gaussian Noise
AI 理解论文
溯源树
样例
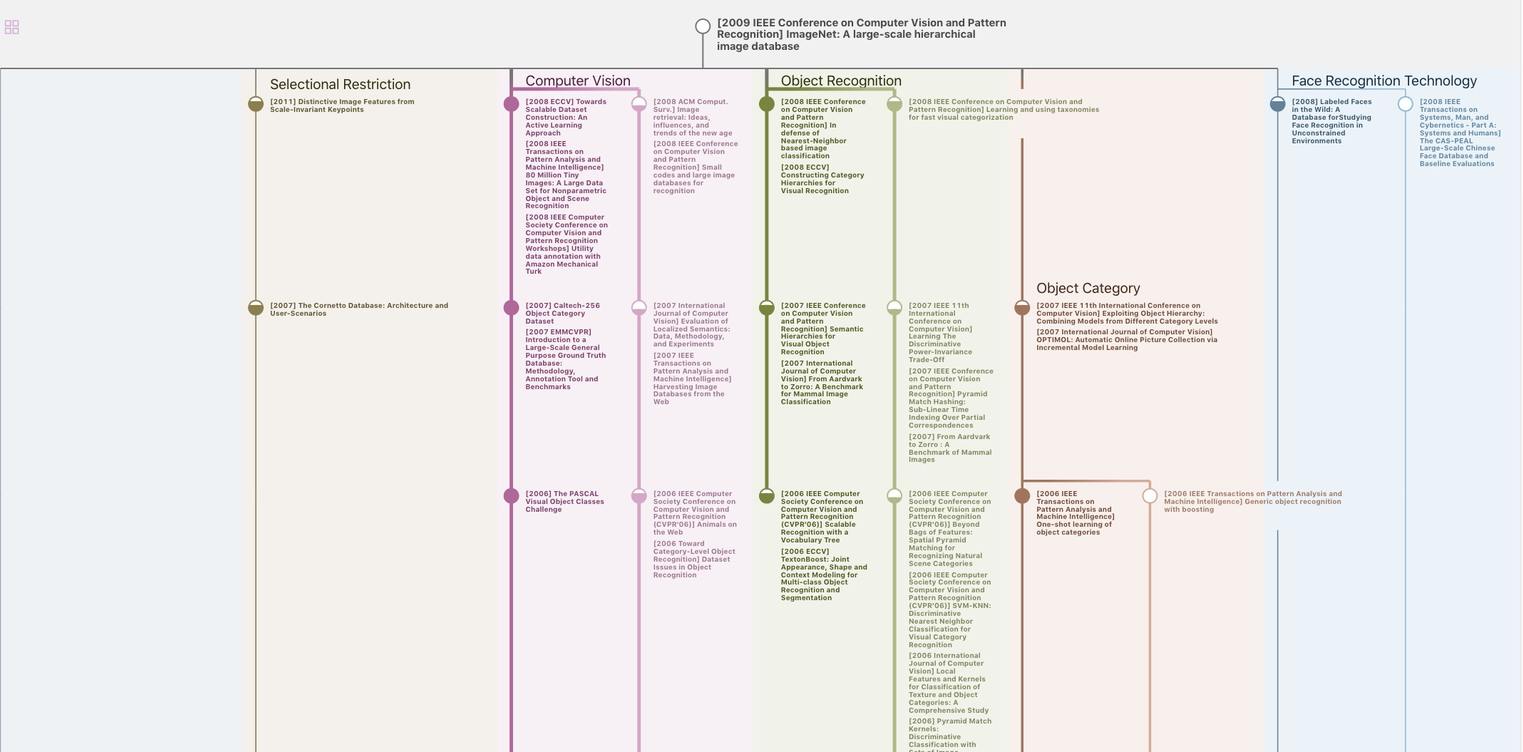
生成溯源树,研究论文发展脉络
Chat Paper
正在生成论文摘要