Rethinking recidivism through a causal lens
arxiv(2020)
摘要
Predictive modeling of criminal recidivism, or whether people will re-offend
in the future, has a long and contentious history. Modern causal inference
methods allow us to move beyond prediction and target the "treatment effect" of
a specific intervention on an outcome in an observational dataset. In this
paper, we look specifically at the effect of incarceration (prison time) on
recidivism, using a well-known dataset from North Carolina. Two popular causal
methods for addressing confounding bias are explained and demonstrated:
directed acyclic graph (DAG) adjustment and double machine learning (DML),
including a sensitivity analysis for unobserved confounders. We find that
incarceration has a detrimental effect on recidivism, i.e., longer prison
sentences make it more likely that individuals will re-offend after release,
although this conclusion should not be generalized beyond the scope of our
data. We hope that this case study can inform future applications of causal
inference to criminal justice analysis.
更多查看译文
AI 理解论文
溯源树
样例
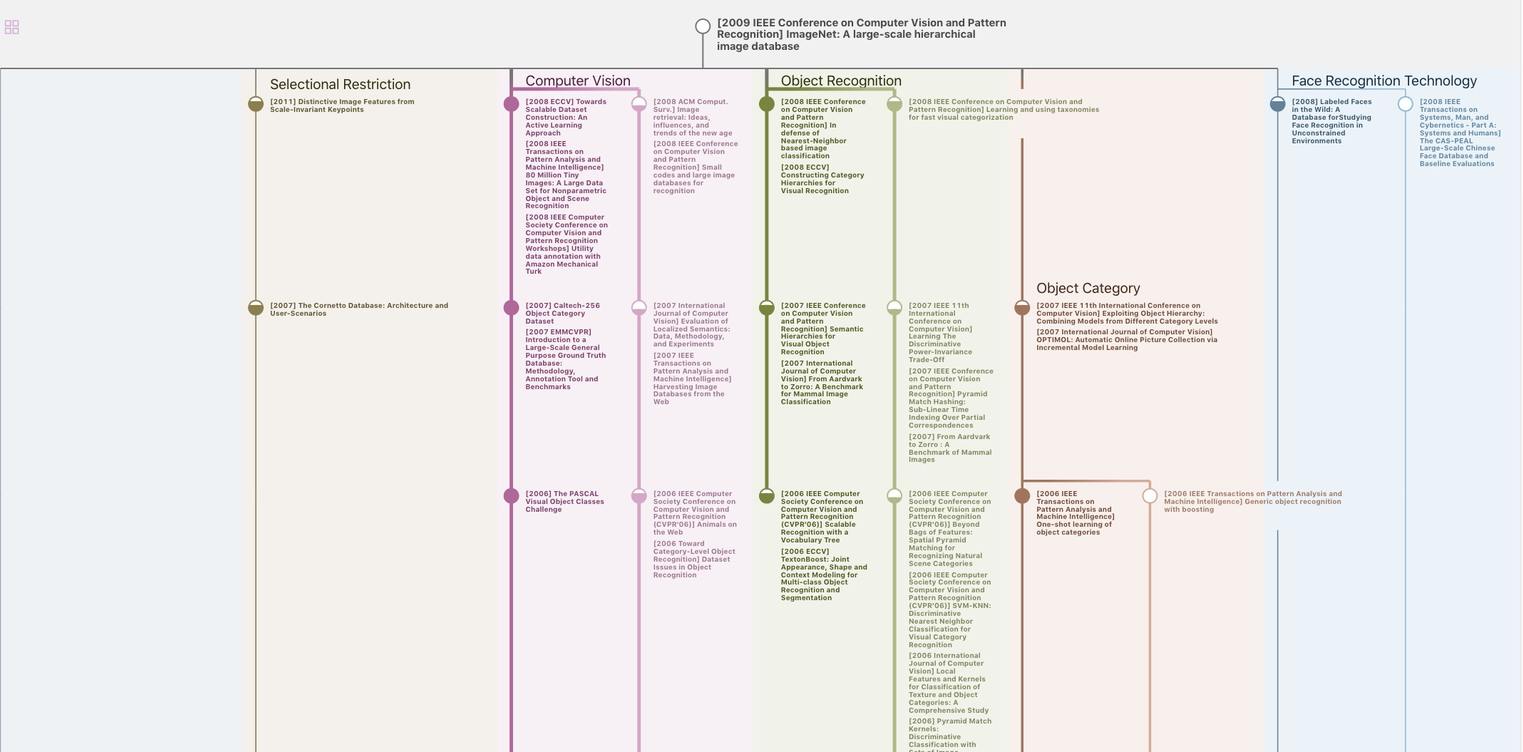
生成溯源树,研究论文发展脉络
Chat Paper
正在生成论文摘要