KPC: Learning-Based Model Predictive Control with Deterministic Guarantees
L4DC(2021)
摘要
We propose Kernel Predictive Control (KPC), a learning-based predictive control strategy that enjoys deterministic guarantees of safety. Noise-corrupted samples of the unknown system dynamics are used to learn several models through the formalism of non-parametric kernel regression. By treating each prediction step individually, we dispense with the need of propagating sets through highly non-linear maps, a procedure that often involves multiple conservative approximation steps. Finite-sample error bounds are then used to enforce state-feasibility by employing an efficient robust formulation. We then present a relaxation strategy that exploits on-line data to weaken the optimization problem constraints while preserving safety. Two numerical examples are provided to illustrate the applicability of the proposed control method.
更多查看译文
关键词
model predictive control,predictive control,kpc,learning-based
AI 理解论文
溯源树
样例
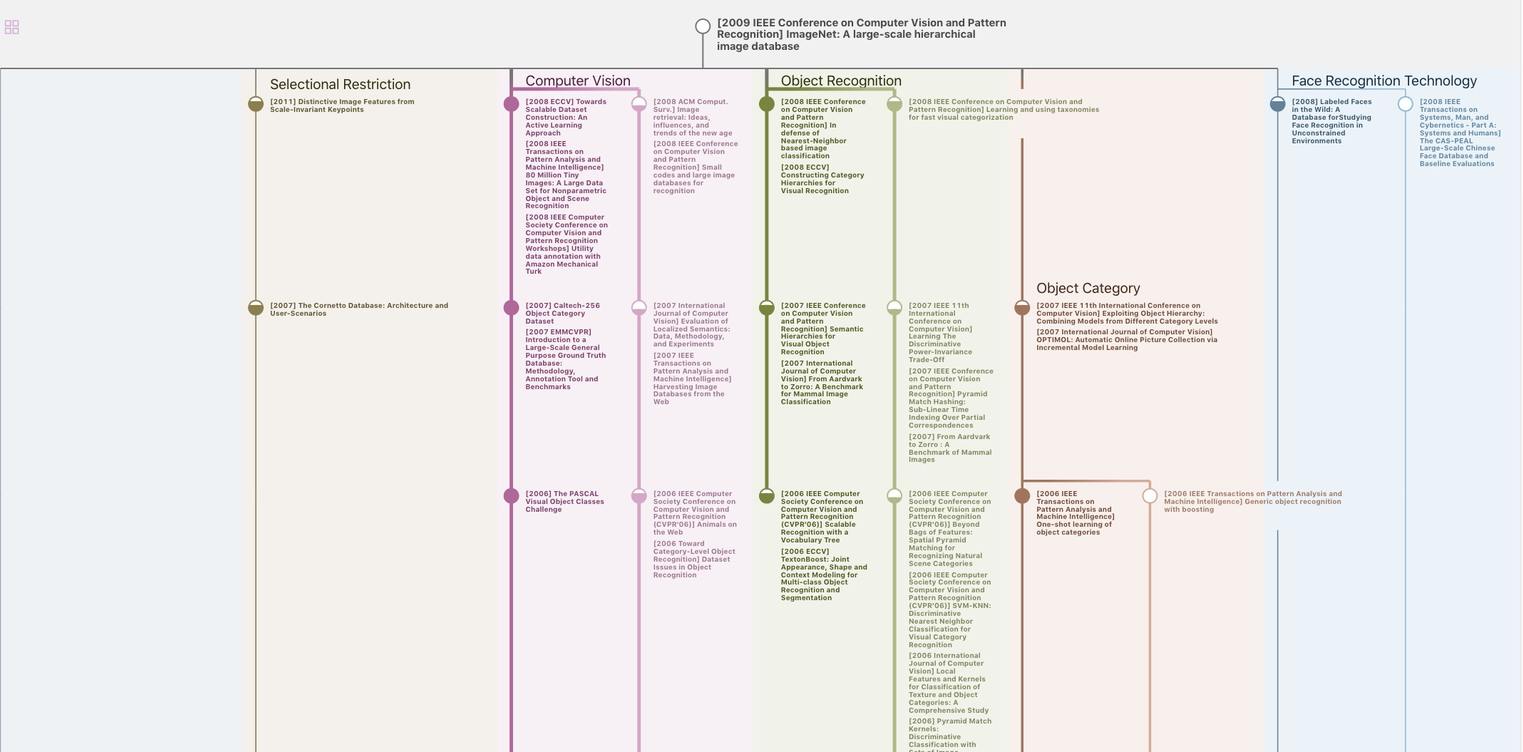
生成溯源树,研究论文发展脉络
Chat Paper
正在生成论文摘要