Multi-experiment Parameter Identifiability of ODEs and Model Theory
SIAM JOURNAL ON APPLIED ALGEBRA AND GEOMETRY(2022)
摘要
Structural identifiability is a property of an ODE model with parameters that allows for the param-eters to be determined from continuous noise-free data. This is a natural prerequisite for practical identifiability. Conducting multiple independent experiments could make more parameters or func-tions of parameters identifiable, which is a desirable property to have. How many experiments are sufficient? In the present paper, we provide an algorithm to determine the exact number of exper-iments for multi-experiment local identifiability and obtain an upper bound that is off at most by one for the number of experiments for multi-experiment global identifiability. Interestingly, the main theoretical ingredient of the algorithm has been discovered and proved using model theory (in the sense of mathematical logic). Based on the insights from the model-theoretic argument, an algebraic proof presented in the paper was obtained. We hope that this unexpected connection will stimulate interactions between applied algebra and model theory, and we provide a short introduction to model theory in the context of parameter identifiability. As another related application of model theory in this area, we construct a nonlinear ODE system with one output such that single-experiment and multiple-experiment identifiability are different for the system. This contrasts with recent results about single-output linear systems. We also present a Monte Carlo randomized version of the algo-rithm with a polynomial arithmetic complexity. Implementation of the algorithm is provided and its performance is demonstrated on several examples.
更多查看译文
关键词
parameter identifiability, multiple experiments, differential algebra, model theory of differential fields
AI 理解论文
溯源树
样例
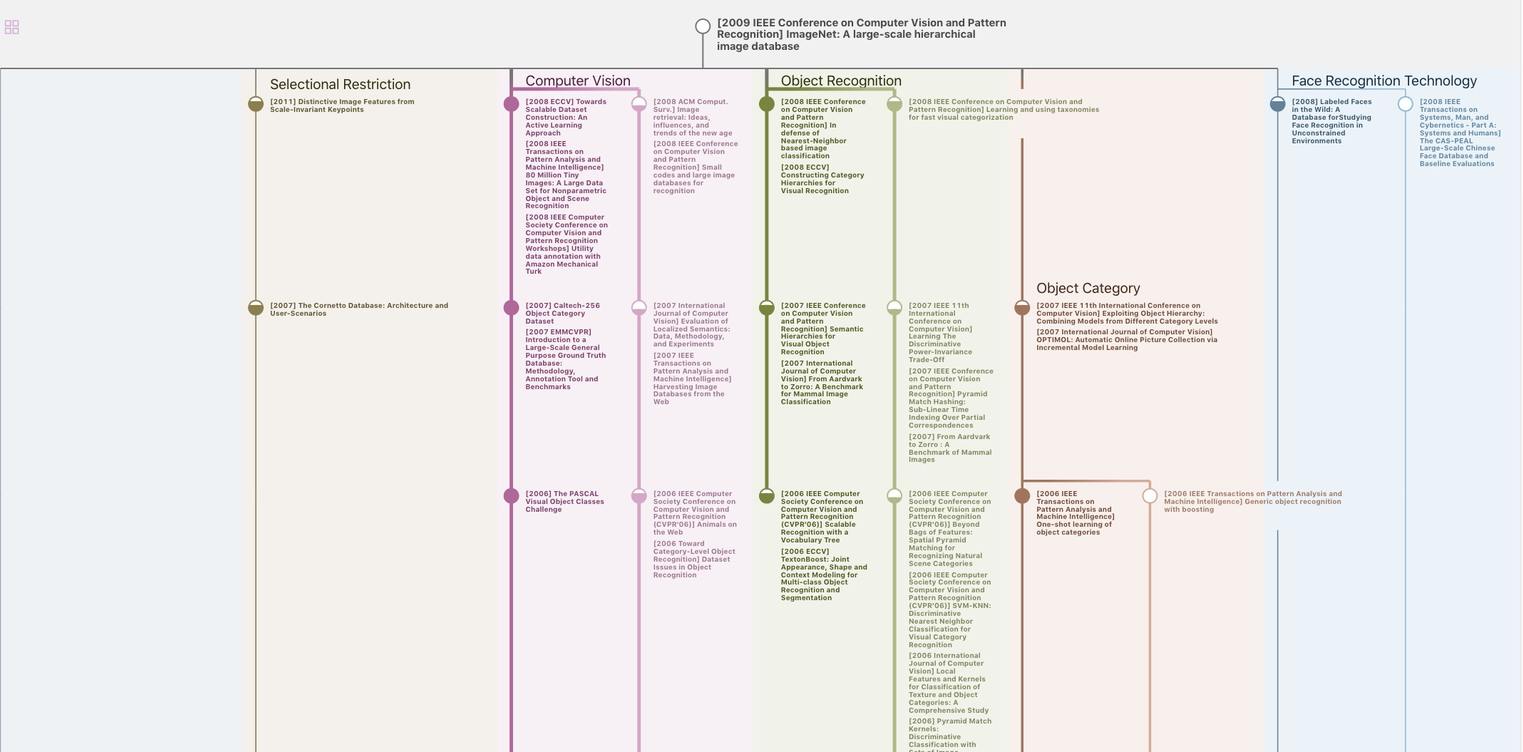
生成溯源树,研究论文发展脉络
Chat Paper
正在生成论文摘要