Towards Robust Data-Driven Control Synthesis for Nonlinear Systems with Actuation Uncertainty.
CDC(2021)
摘要
Modern nonlinear control theory seeks to endow systems with properties such as stability and safety, and has been deployed successfully across various domains. Despite this success, model uncertainty remains a significant challenge in ensuring that model-based controllers transfer to real world systems. This paper develops a data-driven approach to robust control synthesis in the presence of model uncertainty using Control Certificate Functions (CCFs), resulting in a convex optimization based controller for achieving properties like stability and safety. An important benefit of our framework is nuanced data-dependent guarantees, which in principle can yield sample-efficient data collection approaches that need not fully determine the input-to-state relationship. This work serves as a starting point for addressing important questions at the intersection of nonlinear control theory and non-parametric learning, both theoretical and in application. We validate the proposed method in simulation with an inverted pendulum in multiple experimental configurations.
更多查看译文
关键词
robust data-driven control synthesis,nonlinear systems,actuation uncertainty,nonlinear control theory,model uncertainty,model-based controllers,data-driven approach,robust control synthesis,control certificate functions,data-dependent guarantees,sample-efficient data collection approach,input-to-state relationship,nonparametric learning
AI 理解论文
溯源树
样例
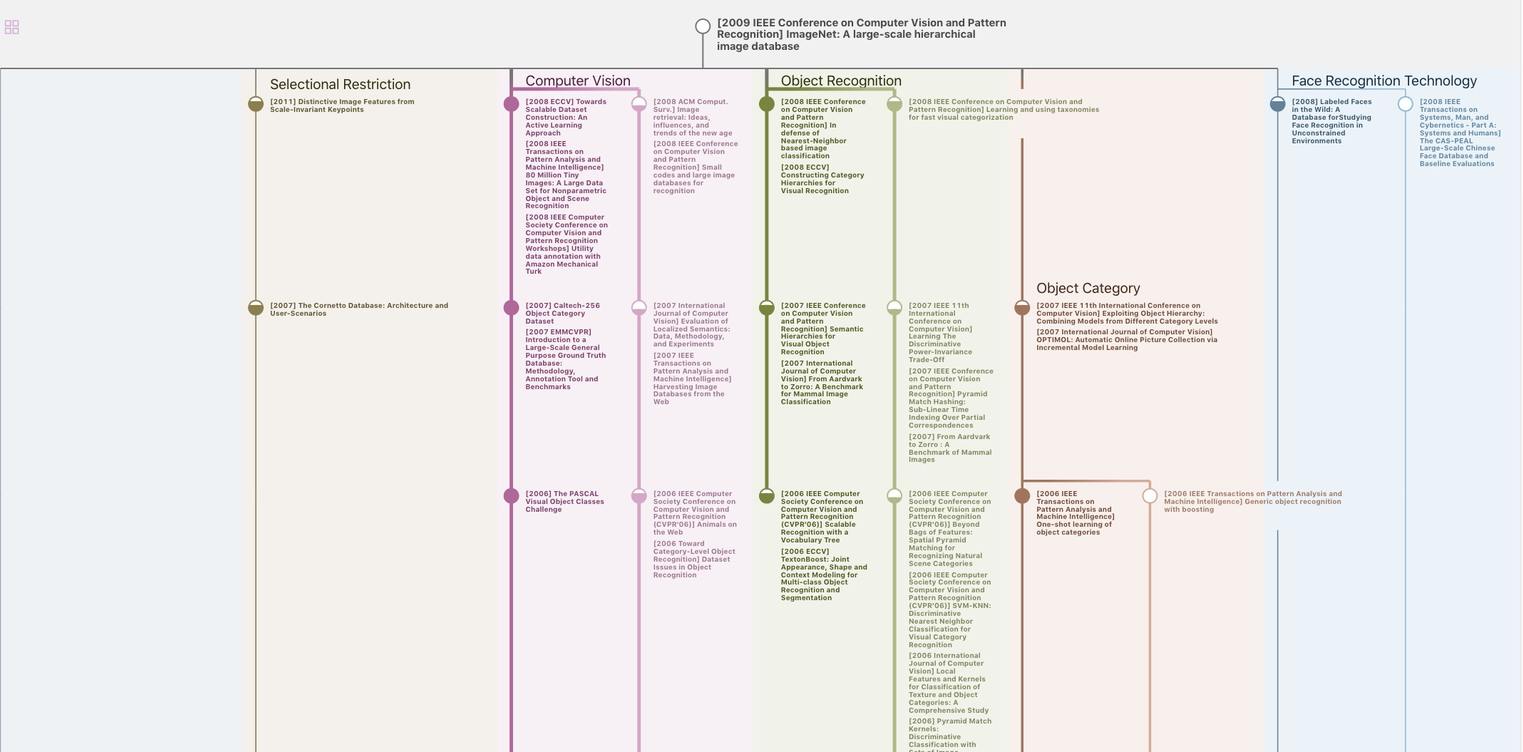
生成溯源树,研究论文发展脉络
Chat Paper
正在生成论文摘要