Improving RNN-T ASR Accuracy Using Untranscribed Context Audio
CoRR(2020)
摘要
We present a new training scheme for streaming automatic speech recognition (ASR) based on recurrent neural network transducers (RNN-T) which allows the encoder network to benefit from longer audio streams as input, while only requiring partial transcriptions of such streams during training. We show that this extension of the acoustic context during training and inference can lead to word error rate reductions of more than 6% in a realistic production setting. We investigate its effect on acoustically challenging data containing background speech and present data points which indicate that this approach helps the network learn both speaker and environment adaptation. Finally, we visualize RNN-T loss gradients with respect to the input features in order to illustrate the ability of a long short-term memory (LSTM) based ASR encoder to exploit long-term context.
更多查看译文
关键词
untranscribed context audio,accuracy
AI 理解论文
溯源树
样例
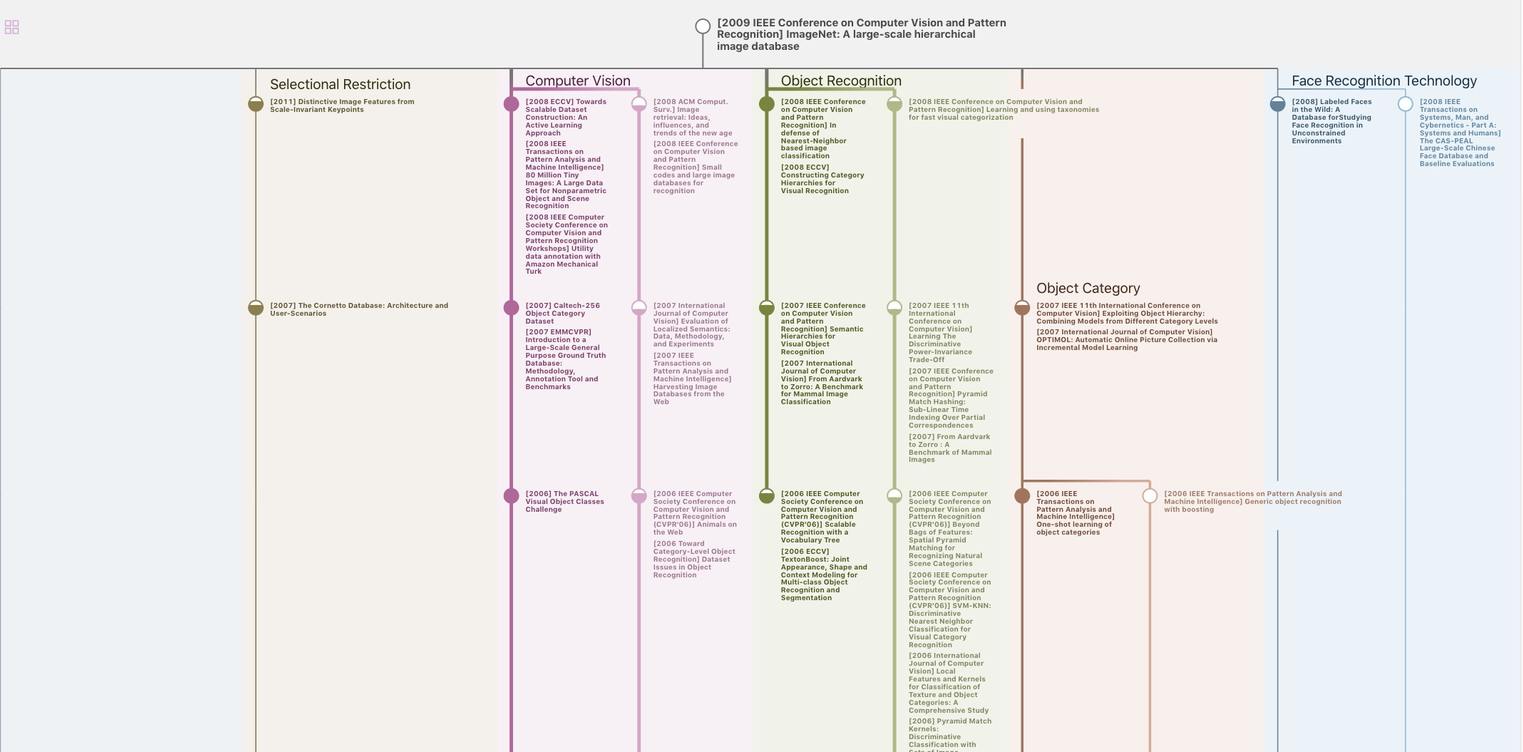
生成溯源树,研究论文发展脉络
Chat Paper
正在生成论文摘要