Self-Supervised Small Soccer Player Detection and Tracking
MM(2020)
Abstract
ABSTRACTIn a soccer game, the information provided by detecting and tracking brings crucial clues to further analyze and understand some tactical aspects of the game, including individual and team actions. State-of-the-art tracking algorithms achieve impressive results in scenarios on which they have been trained for, but they fail in challenging ones such as soccer games. This is frequently due to the player small relative size and the similar appearance among players of the same team. Although a straightforward solution would be to retrain these models by using a more specific dataset, the lack of such publicly available annotated datasets entails searching for other effective solutions. In this work, we propose a self-supervised pipeline which is able to detect and track low-resolution soccer players under different recording conditions without any need of ground-truth data. Extensive quantitative and qualitative experimental results are presented evaluating its performance. We also present a comparison to several state-of-the-art methods showing that both the proposed detector and the proposed tracker achieve top-tier results, in particular in the presence of small players. Code available at "https://github.com/samuro95/Self-Supervised-Small-Soccer-Player-Detection-Tracking".
MoreTranslated text
Key words
small soccer player detection,tracking,self-supervised
AI Read Science
Must-Reading Tree
Example
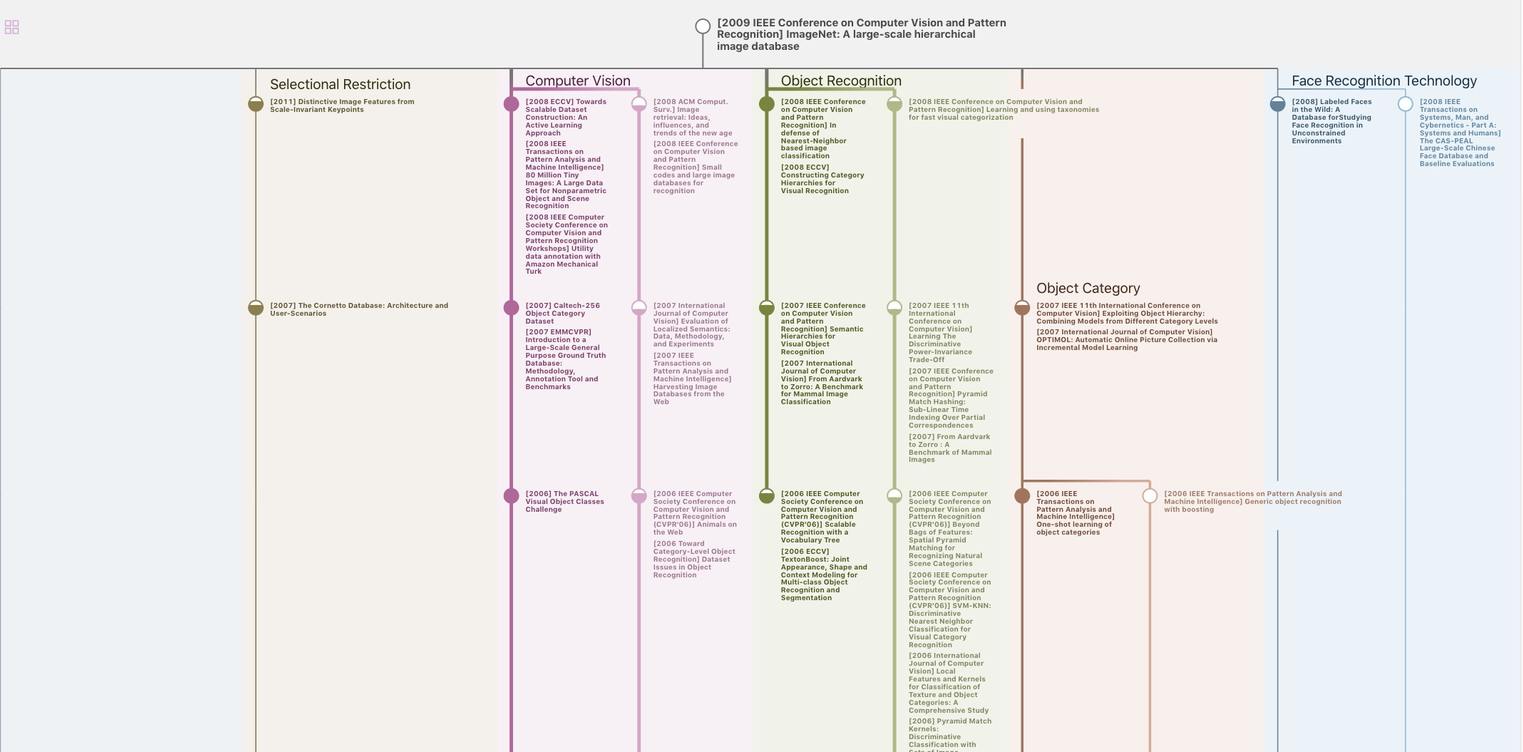
Generate MRT to find the research sequence of this paper
Chat Paper
Summary is being generated by the instructions you defined