ONE SHOT LEARNING FOR SPEECH SEPARATION
2021 IEEE INTERNATIONAL CONFERENCE ON ACOUSTICS, SPEECH AND SIGNAL PROCESSING (ICASSP 2021)(2021)
摘要
Despite the recent success of speech separation models, they fail to separate sources properly while facing different sets of people or noisy environments. To tackle this problem, we proposed to apply meta-learning to the speech separation task. We aimed to find a meta-initialization model, which can quickly adapt to new speakers by seeing only one mixture generated by those people. In this paper, we use model-agnostic meta-learning(MAML) algorithm and almost no inner loop(ANIL) algorithm in Conv-TasNet to achieve this goal. The experiment results show that our model can adapt not only to a new set of speakers but also noisy environments. Furthermore, we found out that the encoder and decoder serve as the feature-reuse layers, while the separator is the task-specific module.
更多查看译文
关键词
Speech separation, meta-learning, MAML, ANIL
AI 理解论文
溯源树
样例
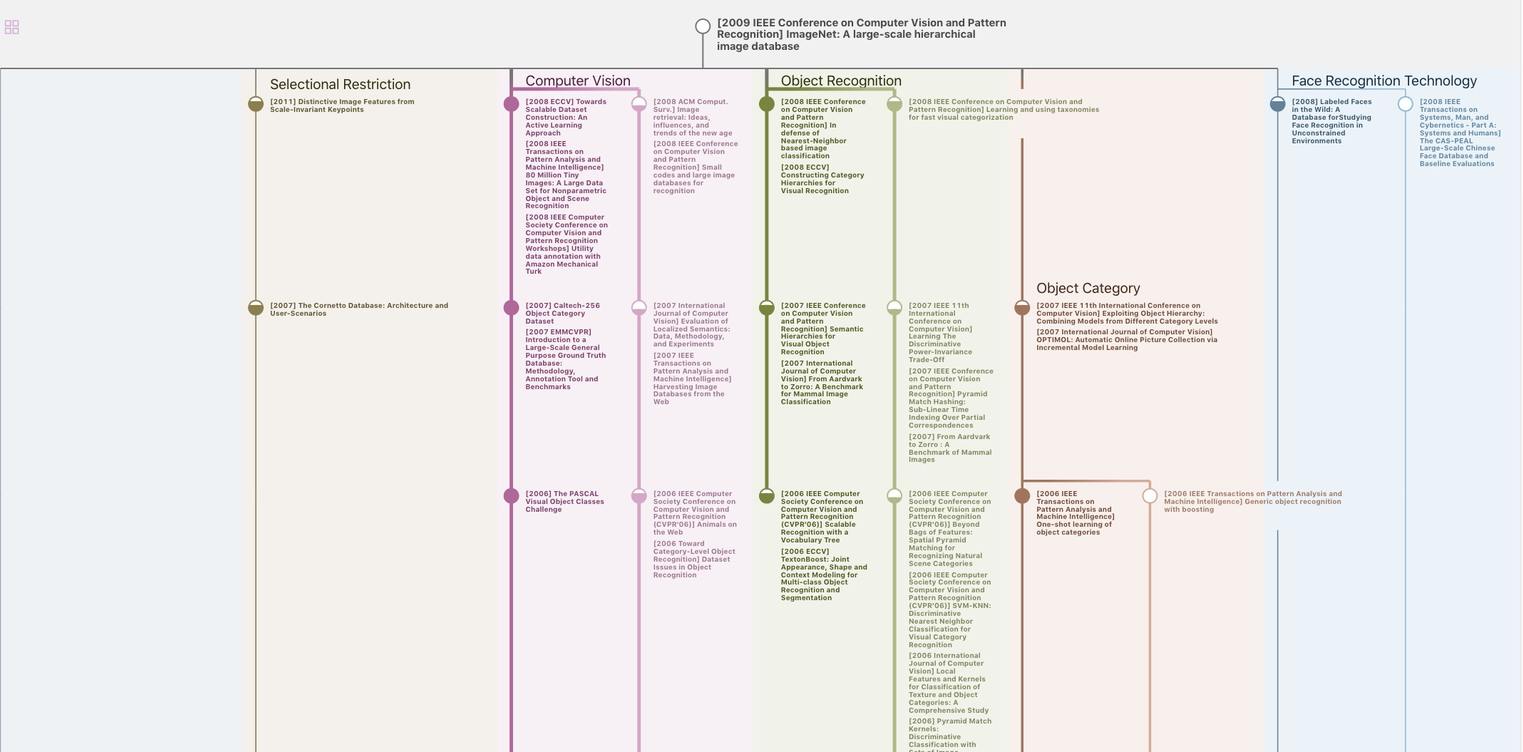
生成溯源树,研究论文发展脉络
Chat Paper
正在生成论文摘要