Preponderantly Increasing/Decreasing Data In Regression Analysis
CROATIAN OPERATIONAL RESEARCH REVIEW(2016)
摘要
For the given data (w(i), x(i), y(i)), i = 1, ..., n, and the given model function f (x;theta), where theta is a vector of unknown parameters, the goal of regression analysis is to obtain estimator theta* of the unknown parameters theta such that the vector of residuals is minimized in some sense. The common approach to this problem of minimization is the least-squares method, that is minimizing the L-2 norm of the vector of residuals. For nonlinear model functions, what is necessary is finding at least the sufficient conditions on the data that will guarantee the existence of the best least-squares estimator. In this paper we will describe and examine in detail the property of preponderant increase/decrease of the data, which ensures the existence of the best estimator for certain important nonlinear model functions.
更多查看译文
关键词
regression analysis, nonlinear least squares, existence problem, preponderant increase/decrease property, Chebyshev inequality
AI 理解论文
溯源树
样例
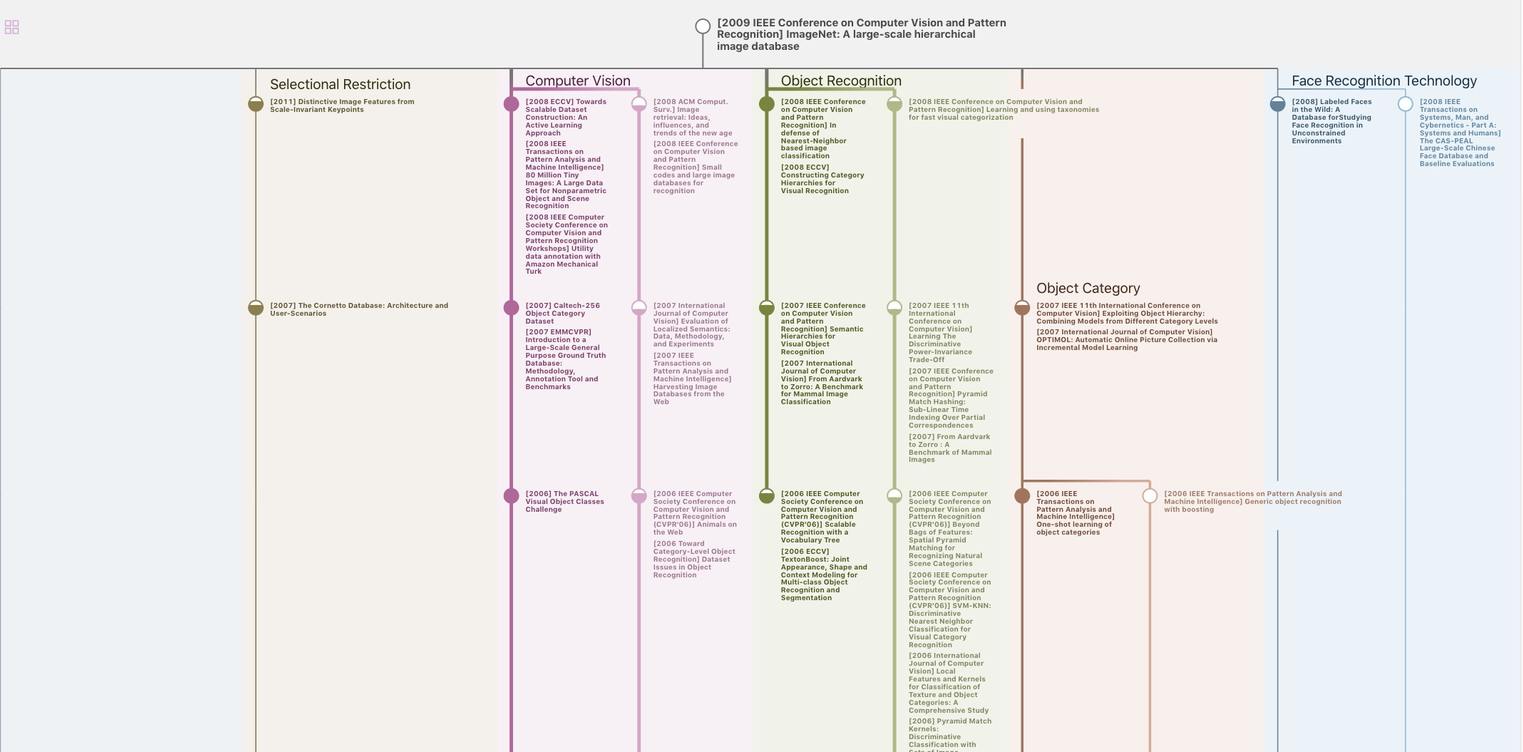
生成溯源树,研究论文发展脉络
Chat Paper
正在生成论文摘要