Simultaneous spatial smoothing and outlier detection using penalized regression, with application to childhood obesity surveillance from electronic health records
BIOMETRICS(2022)
摘要
Electronic health records (EHRs) have become a platform for data-driven granular-level surveillance in recent years. In this paper, we make use of EHRs for early prevention of childhood obesity. The proposed method simultaneously provides smooth disease mapping and outlier information for obesity prevalence that are useful for raising public awareness and facilitating targeted intervention. More precisely, we consider a penalized multilevel generalized linear model. We decompose regional contribution into smooth and sparse signals, which are automatically identified by a combination of fusion and sparse penalties imposed on the likelihood function. In addition, we weigh the proposed likelihood to account for the missingness and potential nonrepresentativeness arising from the EHR data. We develop a novel alternating minimization algorithm, which is computationally efficient, easy to implement, and guarantees convergence. Simulation studies demonstrate superior performance of the proposed method. Finally, we apply our method to the University of Wisconsin Population Health Information Exchange database.
更多查看译文
关键词
childhood obesity surveillance, disease mapping, electronic health records, fusion penalty, outlier detection, sparse penalty
AI 理解论文
溯源树
样例
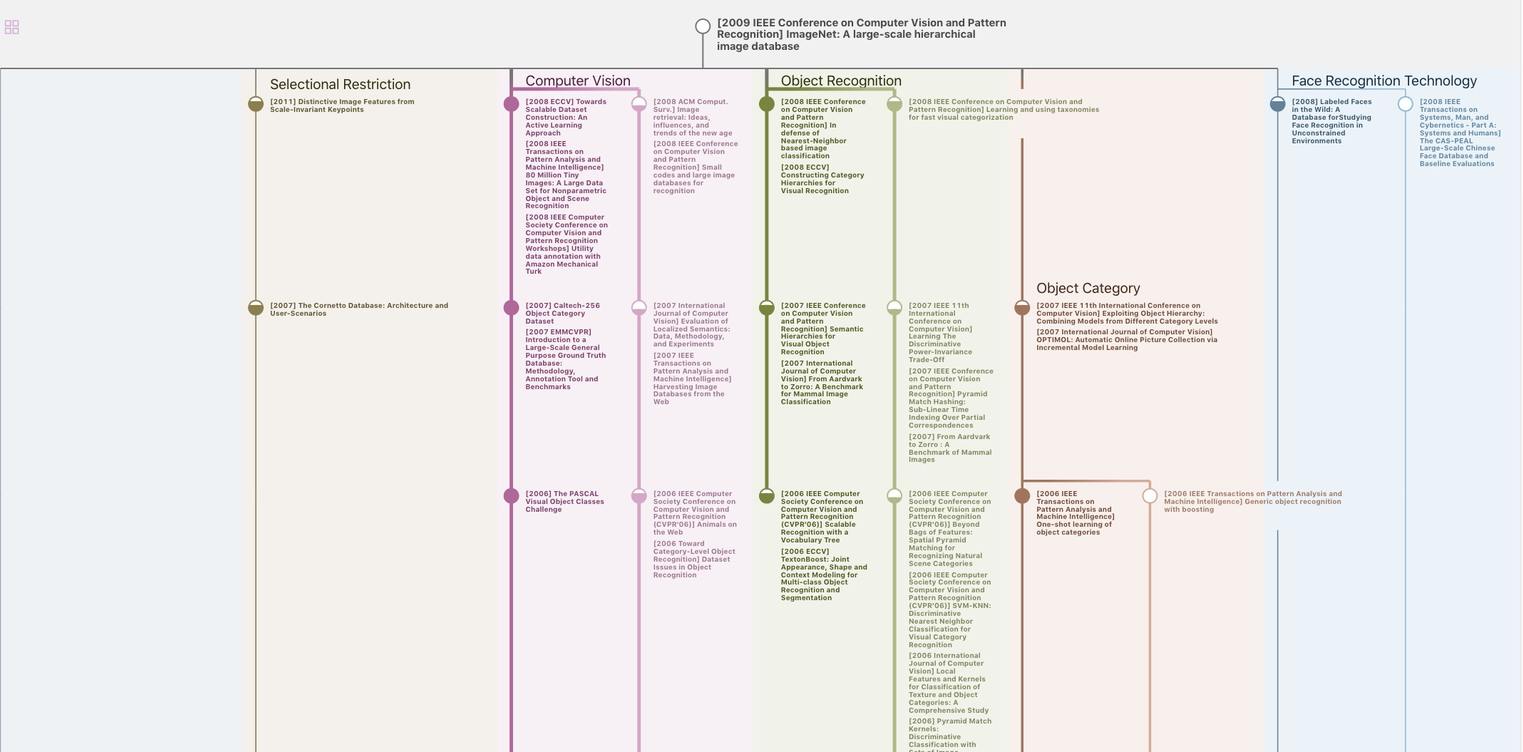
生成溯源树,研究论文发展脉络
Chat Paper
正在生成论文摘要