Bidirectional Lstm-Rnn For Improving Automated Assessment Of Non-Native Children'S Speech
18TH ANNUAL CONFERENCE OF THE INTERNATIONAL SPEECH COMMUNICATION ASSOCIATION (INTERSPEECH 2017), VOLS 1-6: SITUATED INTERACTION(2017)
Abstract
Recent advances in ASR and spoken language processing have led to improved systems for automated assessment for spoken language. However, it is still challenging for automated scoring systems to achieve high performance in terms of the agreement with human experts when applied to non-native children's spontaneous speech. The subpar performance is mainly caused by the relatively low recognition rate on non-native children's speech. In this paper, we investigate different neural network architectures for improving non-native children's speech recognition and the impact of the features extracted from the corresponding ASR output on the automated assessment of speaking proficiency. Experimental results show that bidirectional LSTM-RNN can outperform feed-forward DNN in ASR, with an overall relative WER reduction of 13.4%. The improved speech recognition can then boost the language proficiency assessment performance. Correlations between the rounded automated scores and expert scores range from 0.66 to 0.70 for the three speaking tasks studied, similar to the human human agreement levels for these tasks.
MoreTranslated text
Key words
non-native children's speech, speech recognition, bidirectional LSTM-RNN, DNN and i-vector
AI Read Science
Must-Reading Tree
Example
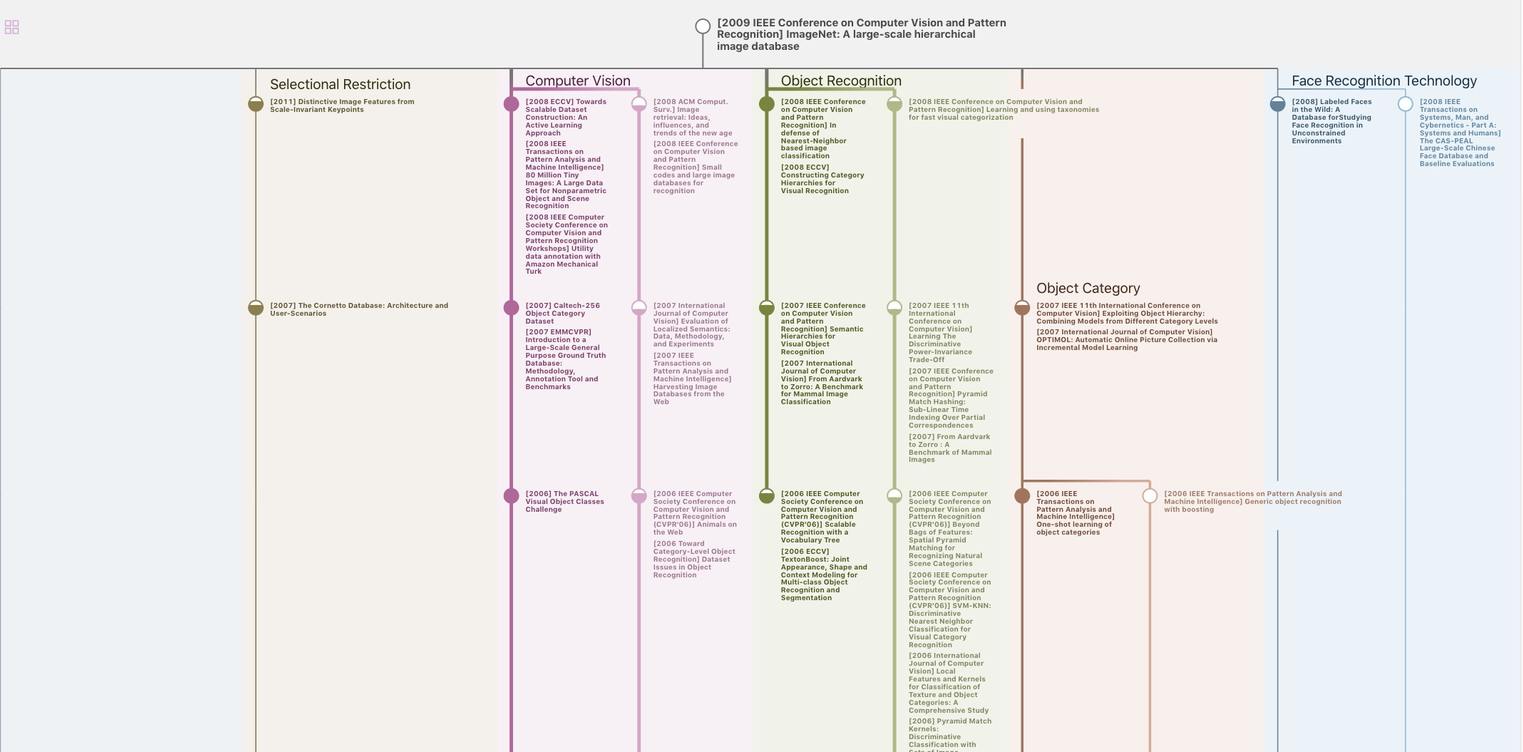
Generate MRT to find the research sequence of this paper
Chat Paper
Summary is being generated by the instructions you defined