Correction: On the asymptotic rate of convergence of Stochastic Newton algorithms and their Weighted Averaged versions
Computational Optimization and Applications(2024)
摘要
Most machine learning methods can be regarded as the minimization of an unavailable risk function. To optimize the latter, with samples provided in a streaming fashion, we define general (weighted averaged) stochastic Newton algorithms, for which a theoretical analysis of their asymptotic efficiency is conducted. The corresponding implementations are shown not to require the inversion of a Hessian estimate at each iteration under a quite flexible framework that covers the case of linear, logistic or softmax regressions to name a few. Numerical experiments on simulated and real data give the empirical evidence of the pertinence of the proposed methods, which outperform popular competitors particularly in case of bad initializations.
更多查看译文
关键词
Stochastic optimization,Newton algorithm,Averaged stochastic algorithm,Online learning
AI 理解论文
溯源树
样例
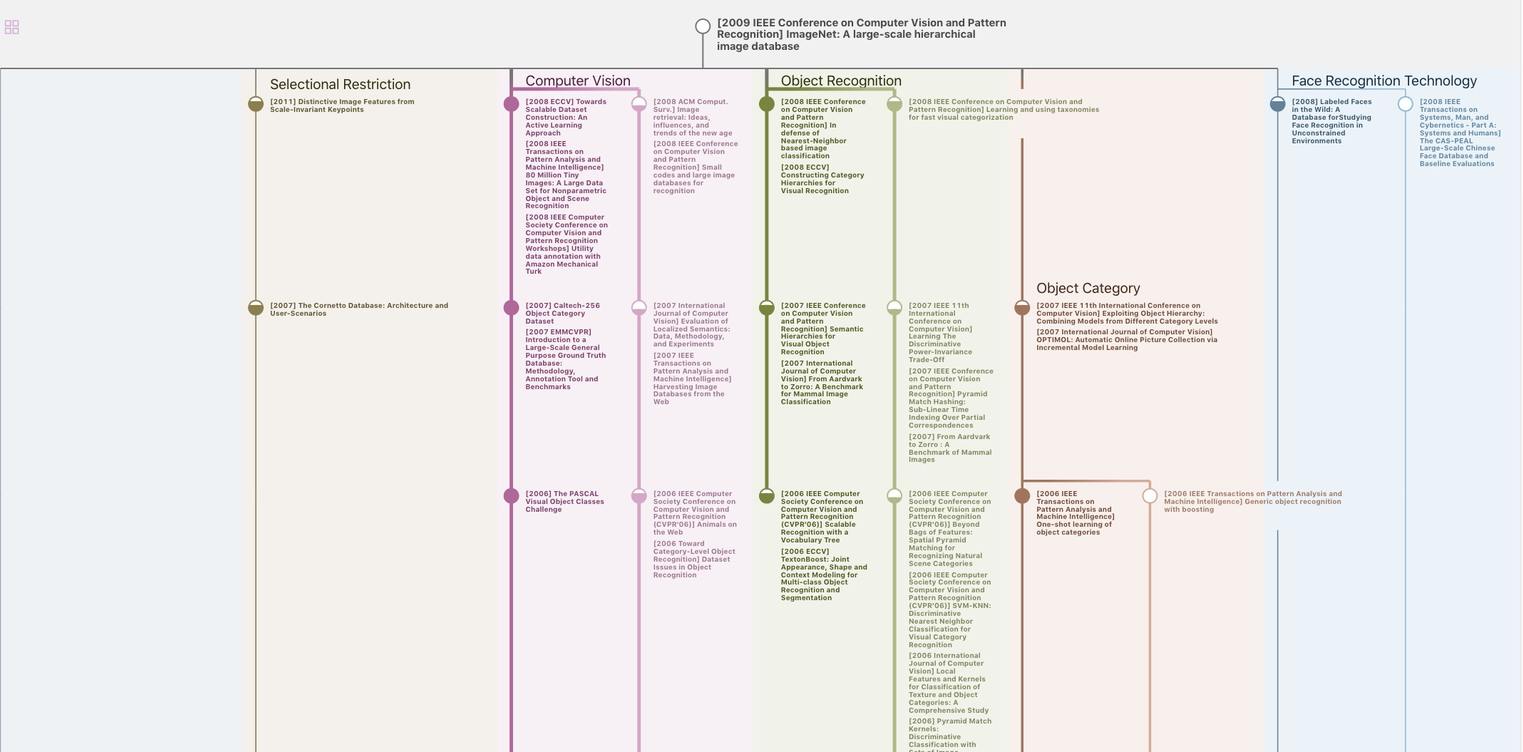
生成溯源树,研究论文发展脉络
Chat Paper
正在生成论文摘要