Density Estimation Using Entropy Maximization For Semi-Continuous Data
DIGITAL SIGNAL PROCESSING(2021)
Abstract
Semi-continuous data comes from a distribution that is a mixture of the point mass at zero and a continuous distribution with support on the positive real line. Such data appear in several real-life situations like blind signal separation problems, modeling of loss data in finance and insurance, sales of consumer goods, daily precipitation data, to name a few. In this paper, we present a novel algorithm to estimate the density function for semi-continuous data using the principle of maximum entropy. Unlike existing methods in the literature, our algorithm provides a consistent estimate of the true maximum entropy distribution and considers both the discrete and continuous parts of the semi-continuous distribution simultaneously. Using simulations, we show that the estimate of the entropy produced by our algorithm has significantly less bias compared to existing methods. An application to the daily rainfall data is provided. (C) 2021 Elsevier Inc. All rights reserved.
MoreTranslated text
Key words
Semi-continuous or mixture data, Density estimation, Entropy maximization, Blind source separation, Daily precipitation
AI Read Science
Must-Reading Tree
Example
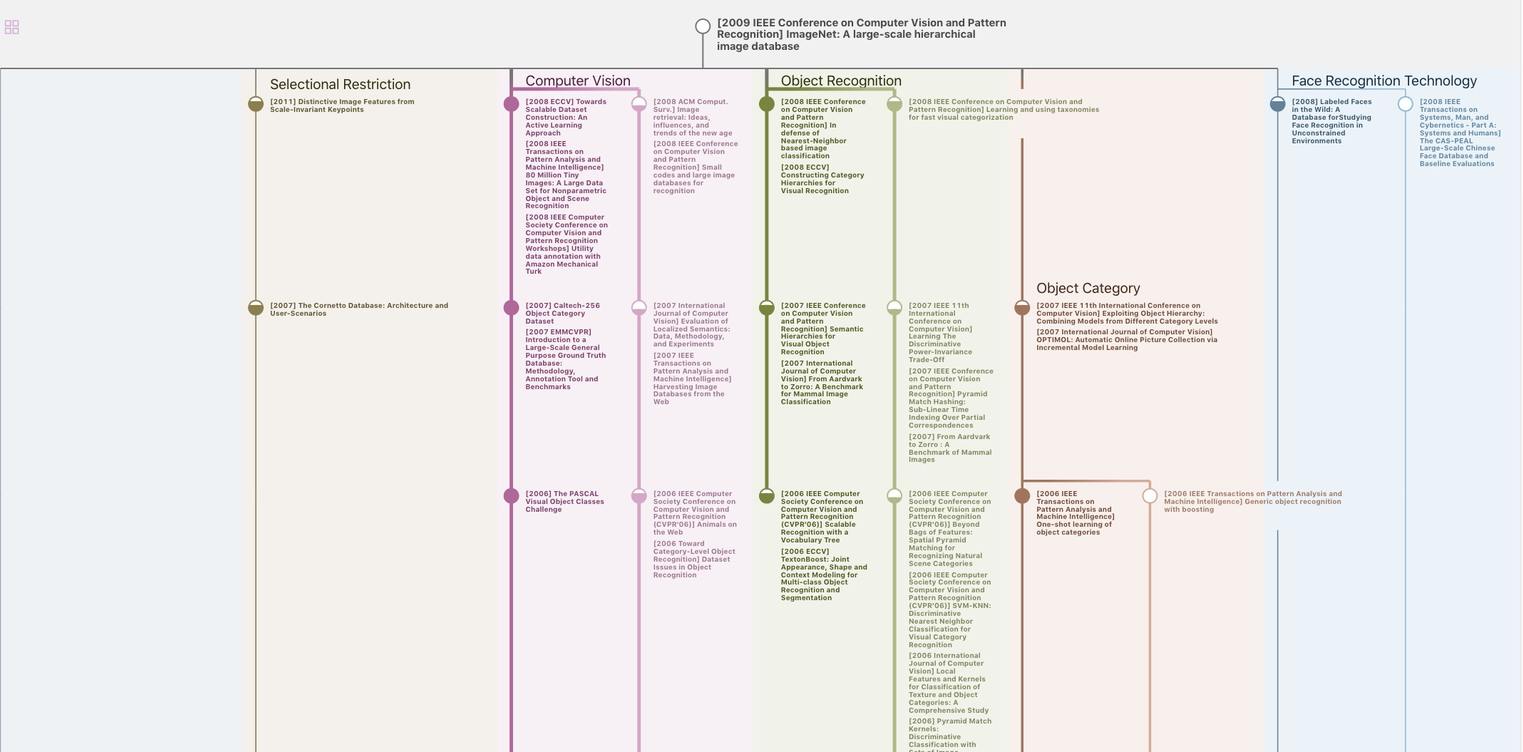
Generate MRT to find the research sequence of this paper
Chat Paper
Summary is being generated by the instructions you defined