Hierarchical complementary learning for weakly supervised object localization
Signal Processing: Image Communication(2022)
摘要
Weakly supervised object localization (WSOL) is a challenging problem that aims to localize objects without ground-truth bounding boxes. A common approach is to train the model that generates a class activation map (CAM) to localize the discriminative features of the object. Unfortunately, the limitation of this method is that they detect just a part of the object and not the whole object. To solve this problem, previous works have removed some parts of the image (Zhang et al., 2018; Zhang et al., 2018; Singh and Lee, 2017; Choe and Shim, 2019) to force the model to detect the full object extent. However, these methods require one or many hyper-parameters to erase the appropriate pixels on the image, which could involve a loss of information. In this paper, we propose a Hierarchical Complementary Learning Network method (HCLNet) that helps the CNN to perform better on classification and localization. HCLNet uses a complementary CAM to generate multiple maps that detect different parts of the object. Unlike previous works, this method does not need any extra hyper-parameters, as well as does not introduce a big loss of information. In order to fuse these different maps, two different fusion strategies known as the addition strategy and the l1-norm strategy have been used. These strategies allow to detect the whole object while excluding the background. Extensive experiments show that HCLNet obtains better performance than state-of-the-art methods.
更多查看译文
关键词
Weakly supervised object localization,Class activation map,Complementary map,Fusion strategy
AI 理解论文
溯源树
样例
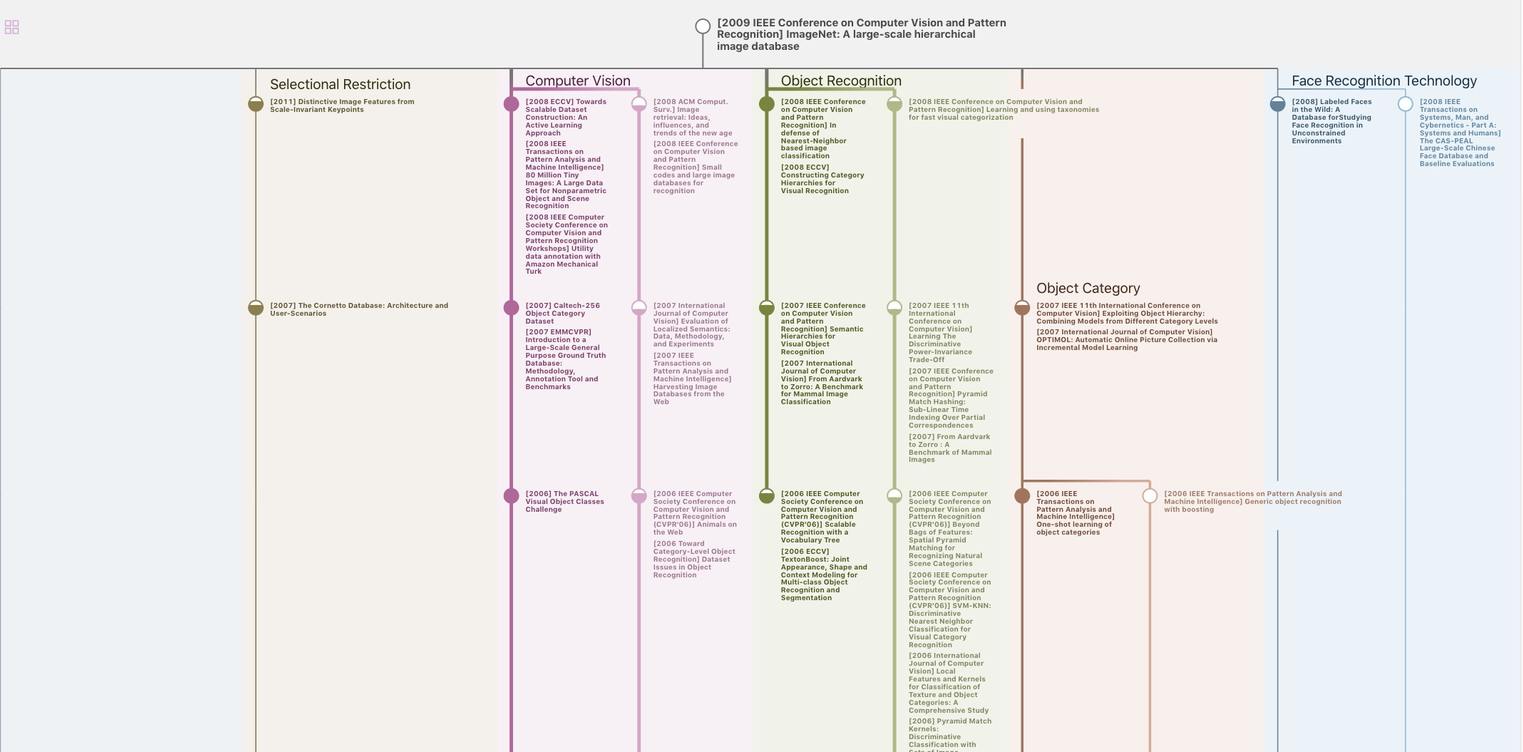
生成溯源树,研究论文发展脉络
Chat Paper
正在生成论文摘要